Unsupervised Adversarial Domain Adaptation for Sim-to-Real Transfer of Tactile Images
IEEE Trans. Instrum. Meas.(2023)
摘要
Transferring optical tactile skills learned from simulated environments to the real world benefits many robotic tactile applications, which can reduce the cost of data collection. However, the models purely trained on simulated data are often difficult to generalize well to the unseen real world due to the domain gap between the faultless training images and testing images with unpredictable manufacturing defects or natural wear. In this article, we propose an adaptively correlation-attentive and task-related network (ACTNet) for tactile image transfer, a novel unsupervised adversarial domain adaptation method to narrow the domain gap for pixel-level tactile perception tasks. An adaptively correlative attention mechanism is introduced to improve the generator, which is capable of leveraging global information and focusing on salient regions. We also construct a task-related constraint loss based on the tactile image classification task to facilitate the zero-shot sim-to-real transfer of the tactile image classifier. Extensive experiment results on tactile image generation and tactile image classification indicate that our method outperforms the state-of-the-art method. Classification accuracy of 92.85% in the real-world environment is achieved without using any real-world classification labels.
更多查看译文
关键词
Attention mechanism,domain adaptation,sim-to-real,tactile sensing,transfer learning
AI 理解论文
溯源树
样例
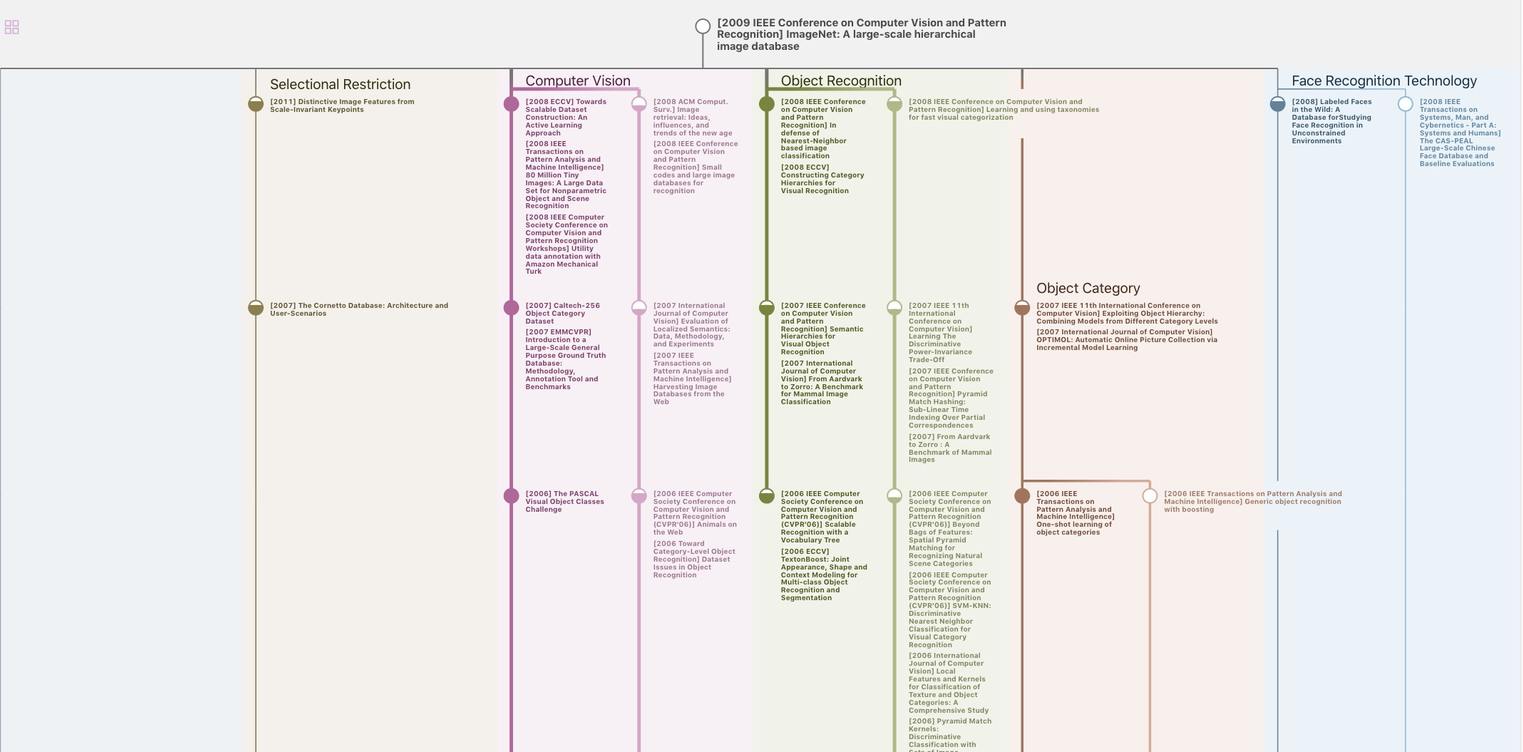
生成溯源树,研究论文发展脉络
Chat Paper
正在生成论文摘要