Domain Generalization for Domain-Linked Classes
CoRR(2023)
摘要
Domain generalization (DG) focuses on transferring domain-invariant knowledge from multiple source domains (available at train time) to an, a priori, unseen target domain(s). This requires a class to be expressed in multiple domains for the learning algorithm to break the spurious correlations between domain and class. However, in the real-world, classes may often be domain-linked, i.e. expressed only in a specific domain, which leads to extremely poor generalization performance for these classes. In this work, we aim to learn generalizable representations for these domain-linked classes by transferring domain-invariant knowledge from classes expressed in multiple source domains (domain-shared classes). To this end, we introduce this task to the community and propose a Fair and cONtrastive feature-space regularization algorithm for Domain-linked DG, FOND. Rigorous and reproducible experiments with baselines across popular DG tasks demonstrate our method and its variants' ability to accomplish state-of-the-art DG results for domain-linked classes. We also provide practical insights on data conditions that increase domain-linked class generalizability to tackle real-world data scarcity.
更多查看译文
关键词
classes,domain-linked
AI 理解论文
溯源树
样例
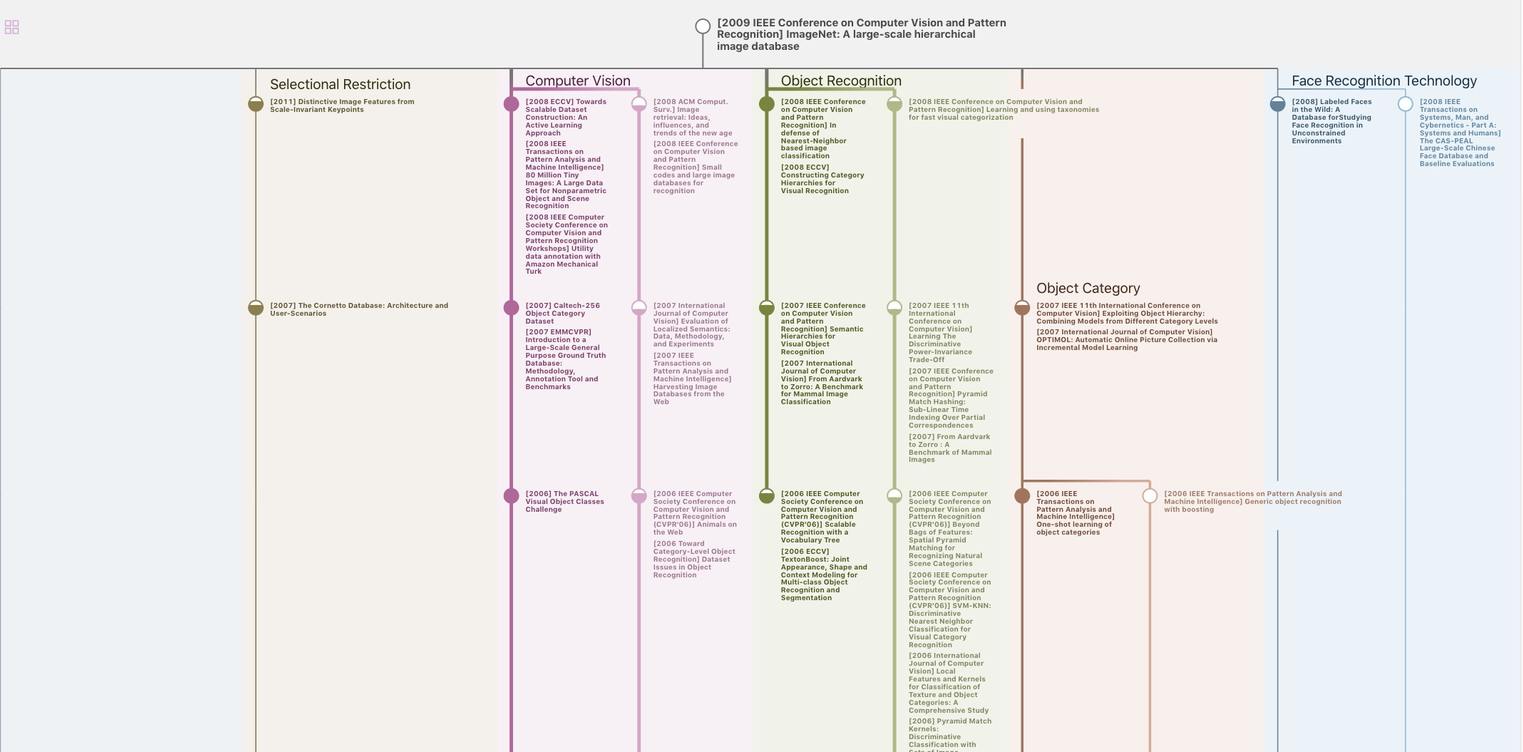
生成溯源树,研究论文发展脉络
Chat Paper
正在生成论文摘要