A transformer-based representation-learning model with unified processing of multimodal input for clinical diagnostics
CoRR(2023)
摘要
During the diagnostic process, clinicians leverage multimodal information, such as the chief complaint, medical images and laboratory test results. Deep-learning models for aiding diagnosis have yet to meet this requirement of leveraging multimodal information. Here we report a transformer-based representation-learning model as a clinical diagnostic aid that processes multimodal input in a unified manner. Rather than learning modality-specific features, the model leverages embedding layers to convert images and unstructured and structured text into visual tokens and text tokens, and uses bidirectional blocks with intramodal and intermodal attention to learn holistic representations of radiographs, the unstructured chief complaint and clinical history, and structured clinical information such as laboratory test results and patient demographic information. The unified model outperformed an image-only model and non-unified multimodal diagnosis models in the identification of pulmonary disease (by 12% and 9%, respectively) and in the prediction of adverse clinical outcomes in patients with COVID-19 (by 29% and 7%, respectively). Unified multimodal transformer-based models may help streamline the triaging of patients and facilitate the clinical decision-making process.
更多查看译文
关键词
multimodal input,clinical diagnostics,transformer-based,representation-learning
AI 理解论文
溯源树
样例
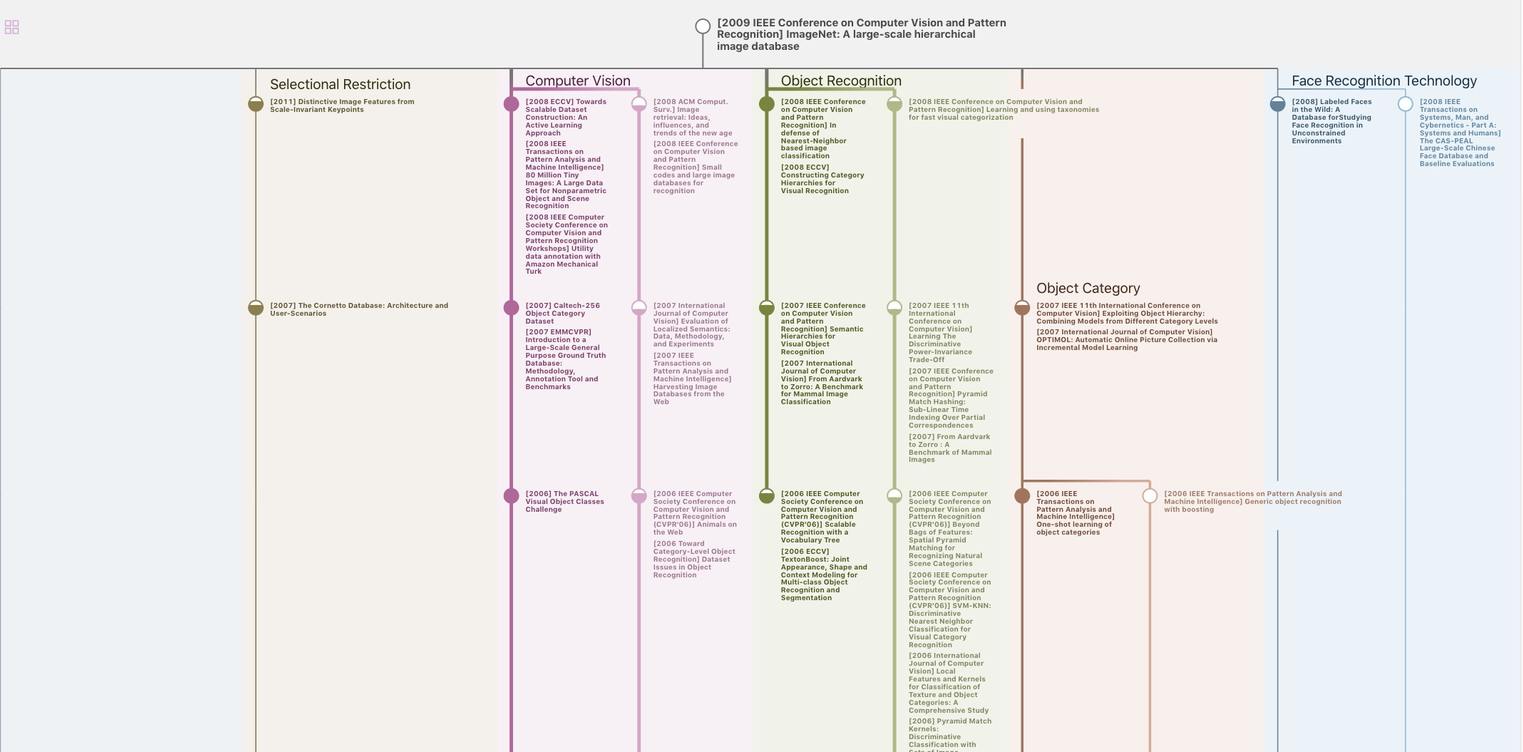
生成溯源树,研究论文发展脉络
Chat Paper
正在生成论文摘要