Efficient Estimation of Pairwise Effective Resistance
Proceedings of the ACM on Management of Data(2023)
摘要
Given an undirected graph G, the effective resistance r(s,t) measures the
dissimilarity of node pair s,t in G, which finds numerous applications in
real-world problems, such as recommender systems, combinatorial optimization,
molecular chemistry, and electric power networks. Existing techniques towards
pairwise effective resistance estimation either trade approximation guarantees
for practical efficiency, or vice versa. In particular, the state-of-the-art
solution is based on a multitude of Monte Carlo random walks, rendering it
rather inefficient in practice, especially on large graphs.
Motivated by this, this paper first presents an improved Monte Carlo
approach, AMC, which reduces both the length and amount of random walks
required without degrading the theoretical accuracy guarantee, through careful
theoretical analysis and an adaptive sampling scheme. Further, we develop a
greedy approach, GEER, which combines AMC with sparse matrix-vector
multiplications in an optimized and non-trivial way. GEER offers significantly
improved practical efficiency over AMC without compromising its asymptotic
performance and accuracy guarantees. Extensive experiments on multiple
benchmark datasets reveal that GEER is orders of magnitude faster than the
state of the art in terms of computational time when achieving the same
accuracy.
更多查看译文
关键词
resistance,estimation
AI 理解论文
溯源树
样例
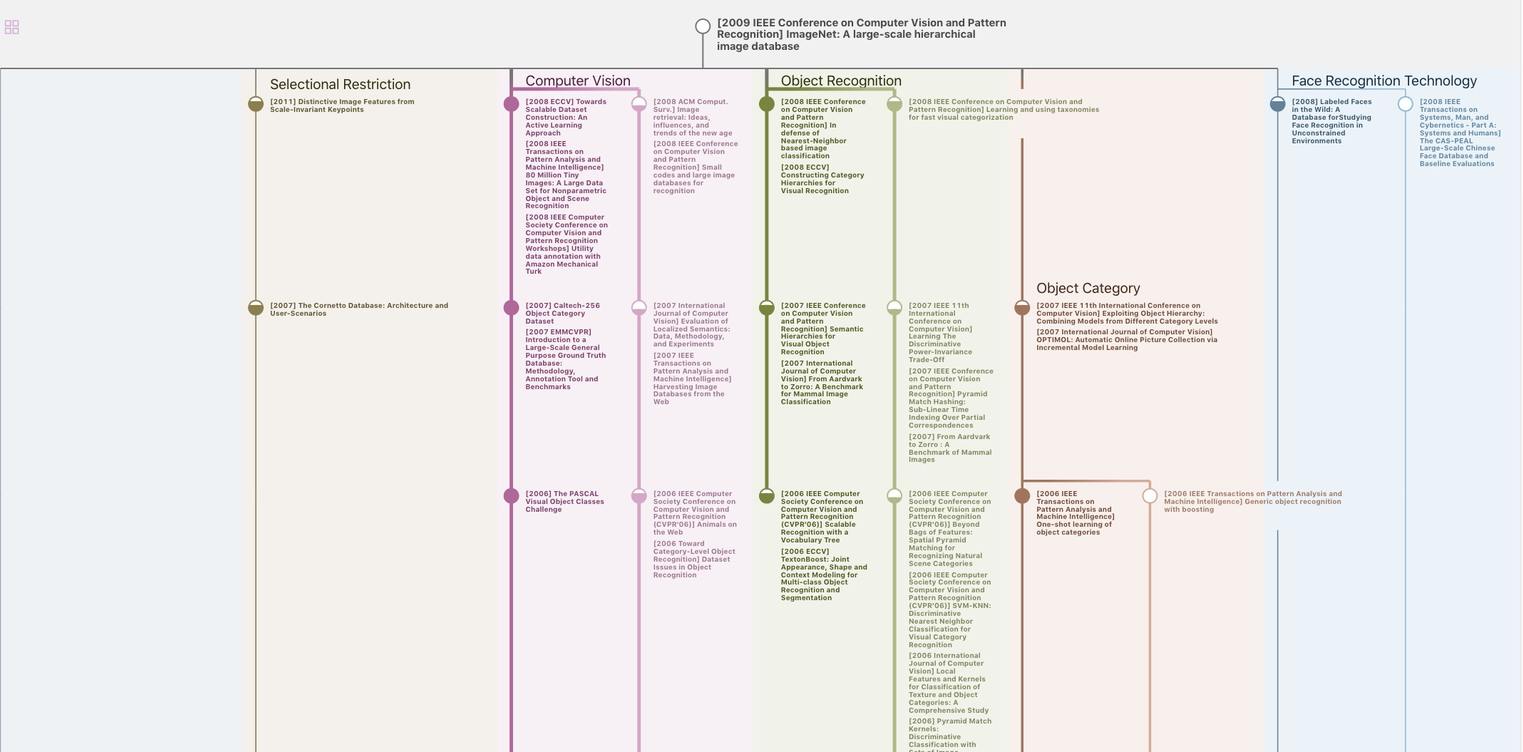
生成溯源树,研究论文发展脉络
Chat Paper
正在生成论文摘要