Variational $f$-Divergence and Derangements for Discriminative Mutual Information Estimation
CoRR(2023)
摘要
The accurate estimation of the mutual information is a crucial task in various applications, including machine learning, communications, and biology, since it enables the understanding of complex systems. High-dimensional data render the task extremely challenging due to the amount of data to be processed and the presence of convoluted patterns. Neural estimators based on variational lower bounds of the mutual information have gained attention in recent years but they are prone to either high bias or high variance as a consequence of the partition function. We propose a novel class of discriminative mutual information estimators based on the variational representation of the $f$-divergence. We investigate the impact of the permutation function used to obtain the marginal training samples and present a novel architectural solution based on derangements. The proposed estimator is flexible as it exhibits an excellent bias/variance trade-off. Experiments on reference scenarios demonstrate that our approach outperforms state-of-the-art neural estimators both in terms of accuracy and complexity.
更多查看译文
关键词
discriminative mutual
AI 理解论文
溯源树
样例
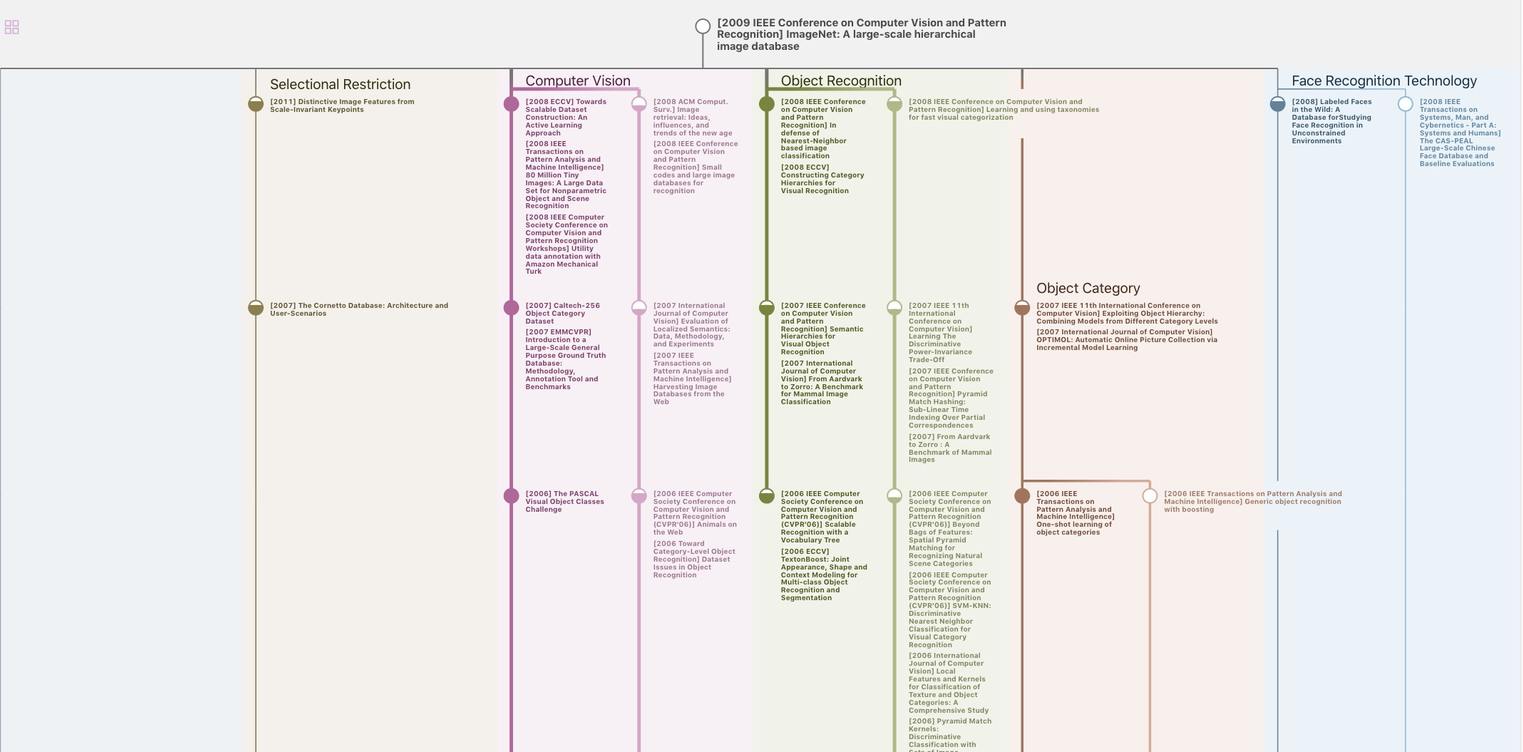
生成溯源树,研究论文发展脉络
Chat Paper
正在生成论文摘要