Monotonic Location Attention for Length Generalization
CoRR(2023)
摘要
We explore different ways to utilize position-based cross-attention in seq2seq networks to enable length generalization in algorithmic tasks. We show that a simple approach of interpolating the original and reversed encoded representations combined with relative attention allows near-perfect length generalization for both forward and reverse lookup tasks or copy tasks that had been generally hard to tackle. We also devise harder diagnostic tasks where the relative distance of the ideal attention position varies with timestep. In such settings, the simple interpolation trick with relative attention is not sufficient. We introduce novel variants of location attention building on top of Dubois et al. (2020) to address the new diagnostic tasks. We also show the benefits of our approaches for length generalization in SCAN (Lake & Baroni, 2018) and CFQ (Keysers et al., 2020). Our code is available on GitHub.
更多查看译文
关键词
attention,generalization,location,length
AI 理解论文
溯源树
样例
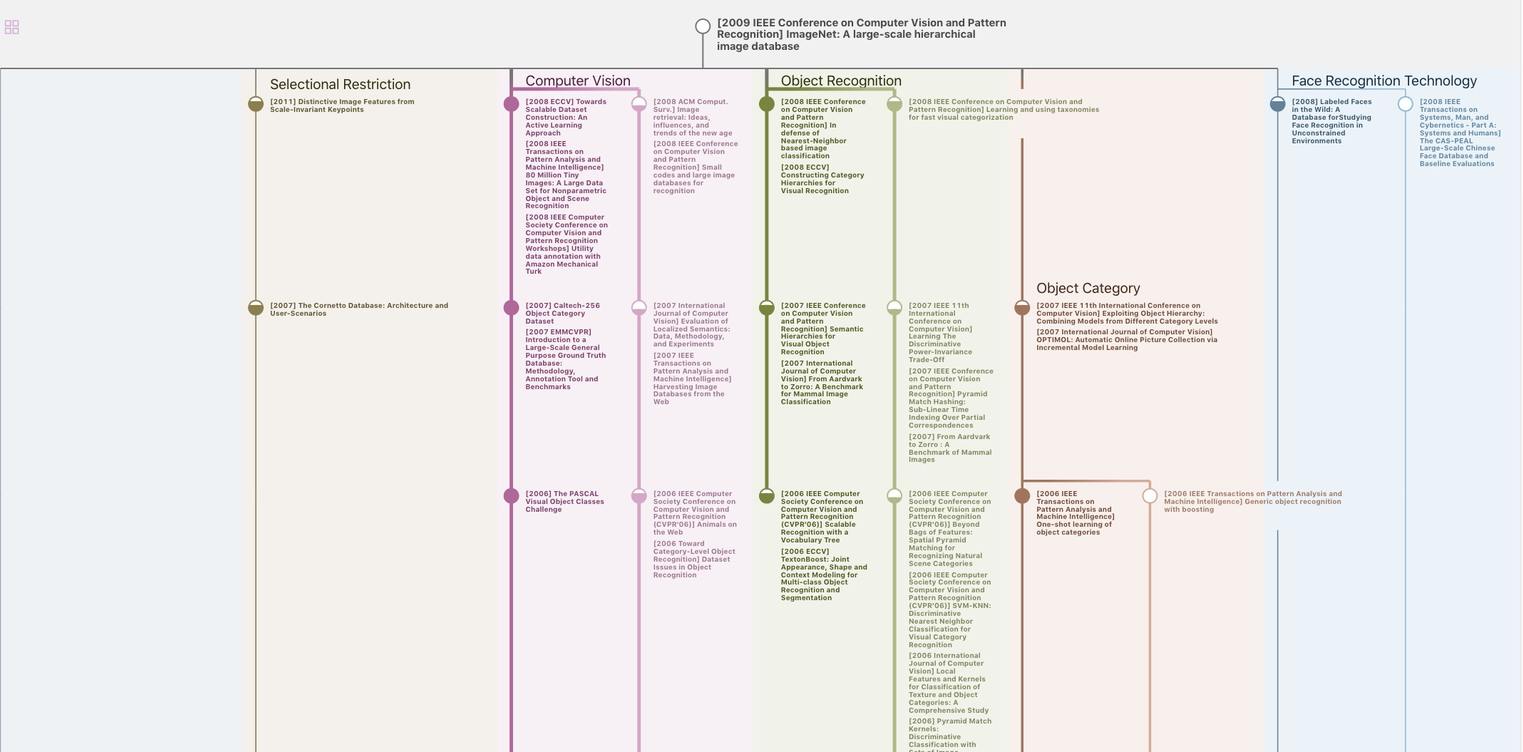
生成溯源树,研究论文发展脉络
Chat Paper
正在生成论文摘要