Physics-Informed Ensemble Representation for Light-Field Image Super-Resolution
CoRR(2023)
摘要
Recent learning-based approaches have achieved significant progress in light field (LF) image super-resolution (SR) by exploring convolution-based or transformer-based network structures. However, LF imaging has many intrinsic physical priors that have not been fully exploited. In this paper, we analyze the coordinate transformation of the LF imaging process to reveal the geometric relationship in the LF images. Based on such geometric priors, we introduce a new LF subspace of virtual-slit images (VSI) that provide sub-pixel information complementary to sub-aperture images. To leverage the abundant correlation across the four-dimensional data with manageable complexity, we propose learning ensemble representation of all $C_4^2$ LF subspaces for more effective feature extraction. To super-resolve image structures from undersampled LF data, we propose a geometry-aware decoder, named EPIXformer, which constrains the transformer's operational searching regions with a LF physical prior. Experimental results on both spatial and angular SR tasks demonstrate that the proposed method outperforms other state-of-the-art schemes, especially in handling various disparities.
更多查看译文
关键词
physics-informed,light-field,super-resolution
AI 理解论文
溯源树
样例
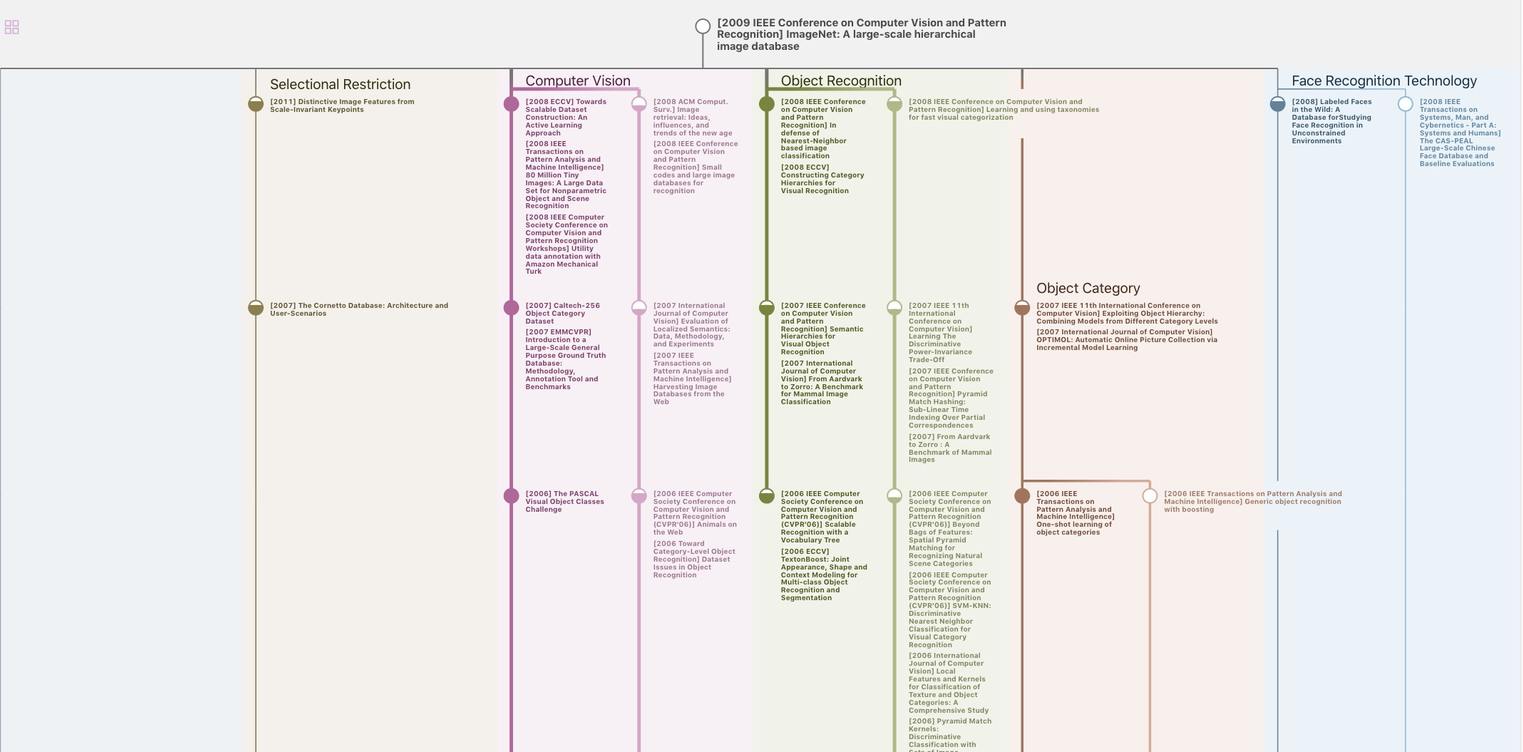
生成溯源树,研究论文发展脉络
Chat Paper
正在生成论文摘要