Treasure in Distribution: A Domain Randomization Based Multi-source Domain Generalization for 2D Medical Image Segmentation
MEDICAL IMAGE COMPUTING AND COMPUTER ASSISTED INTERVENTION, MICCAI 2023, PT IV(2023)
摘要
Although recent years have witnessed the great success of convolutional neural networks (CNNs) in medical image segmentation, the domain shift issue caused by the highly variable image quality of medical images hinders the deployment of CNNs in real-world clinical applications. Domain generalization (DG) methods aim to address this issue by training a robust model on the source domain, which has a strong generalization ability. Previously, many DG methods based on feature-space domain randomization have been proposed, which, however, suffer from the limited and unordered search space of feature styles. In this paper, we propose a multi-source DG method called Treasure in Distribution (TriD), which constructs an unprecedented search space to obtain the model with strong robustness by randomly sampling from a uniform distribution. To learn the domain-invariant representations explicitly, we further devise a style-mixing strategy in our TriD, which mixes the feature styles by randomly mixing the augmented and original statistics along the channel wise and can be extended to other DG methods. Extensive experiments on two medical segmentation tasks with different modalities demonstrate that our TriD achieves superior generalization performance on unseen target-domain data. Code is available at https://github.com/Chen-Ziyang/TriD.
更多查看译文
关键词
Domain generalization,Domain randomization,Medical image segmentation,Deep learning
AI 理解论文
溯源树
样例
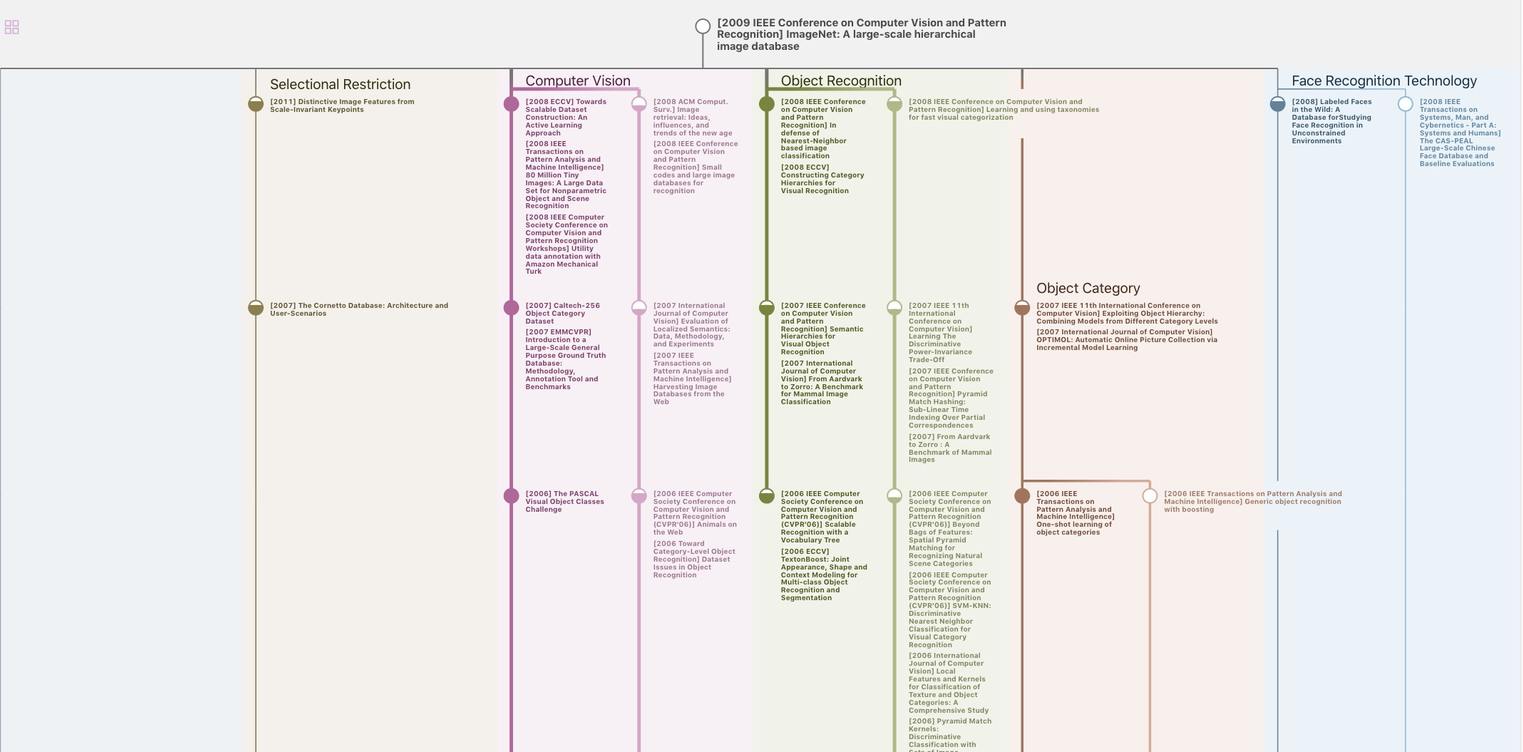
生成溯源树,研究论文发展脉络
Chat Paper
正在生成论文摘要