How to Plant Trees in Language Models: Data and Architectural Effects on the Emergence of Syntactic Inductive Biases
conf_acl(2023)
摘要
Accurate syntactic representations are essential for robust generalization in natural language. Recent work has found that pre-training can teach language models to rely on hierarchical syntactic features - as opposed to incorrect linear features - when performing tasks after fine-tuning. We test what aspects of pre-training are important for endowing encoder-decoder Transformers with an inductive bias that favors hierarchical syntactic generalizations. We focus on architectural features (depth, width, and number of parameters), as well as the genre and size of the pre-training corpus, diagnosing inductive biases using two syntactic transformation tasks: question formation and passivization, both in English. We find that the number of parameters alone does not explain hierarchical generalization: model depth plays greater role than model width. We also find that pre-training on simpler language, such as child-directed speech, induces a hierarchical bias using an order-of-magnitude less data than pre-training on more typical datasets based on web text or Wikipedia; this suggests that in cognitively plausible language acquisition settings, neural language models may be more data-efficient than previously thought.
更多查看译文
关键词
language models,trees,emergence
AI 理解论文
溯源树
样例
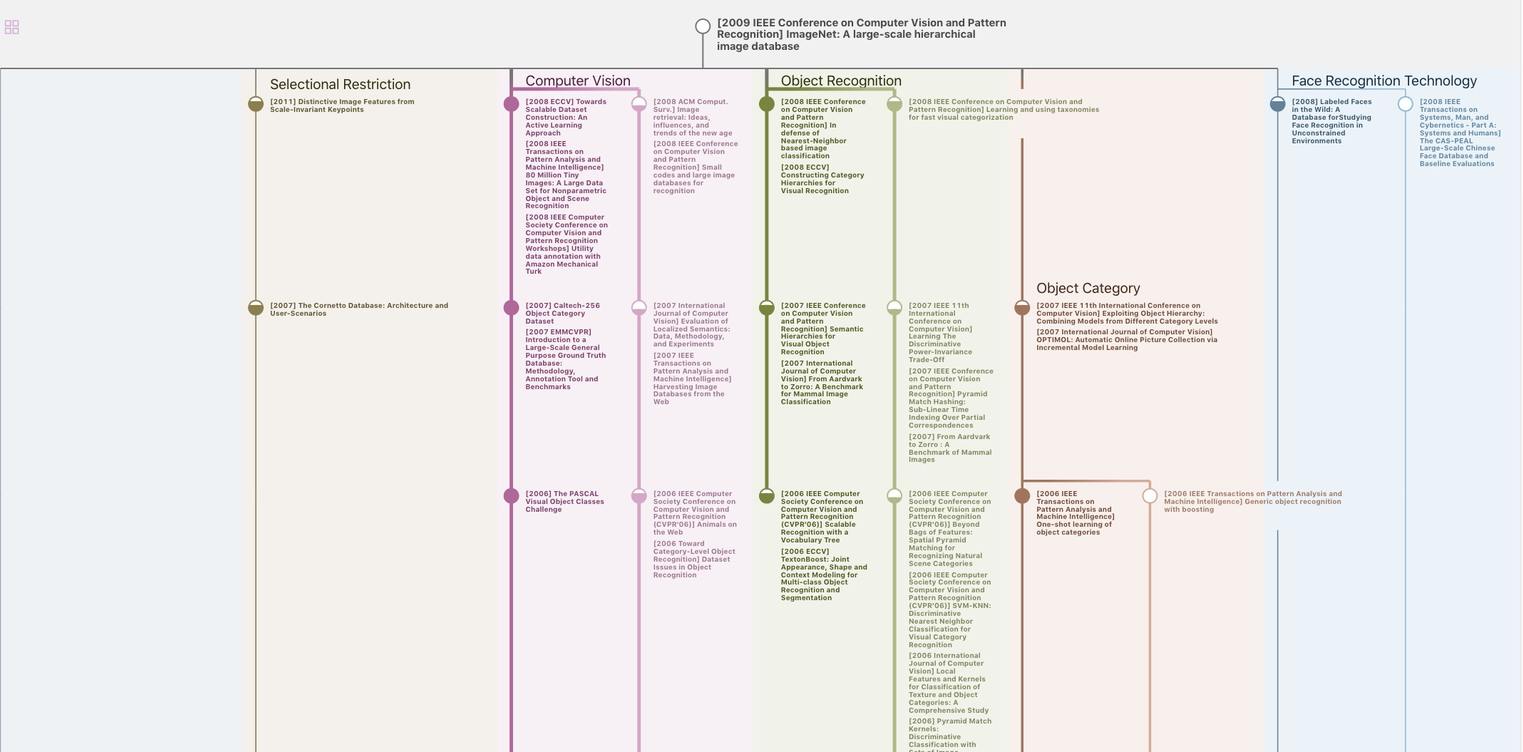
生成溯源树,研究论文发展脉络
Chat Paper
正在生成论文摘要