Measuring and Predicting the Quality of a Join for Data Discovery
CoRR(2023)
摘要
We study the problem of discovering joinable datasets at scale. We approach the problem from a learning perspective relying on profiles. These are succinct representations that capture the underlying characteristics of the schemata and data values of datasets, which can be efficiently extracted in a distributed and parallel fashion. Profiles are then compared, to predict the quality of a join operation among a pair of attributes from different datasets. In contrast to the state-of-the-art, we define a novel notion of join quality that relies on a metric considering both the containment and cardinality proportion between join candidate attributes. We implement our approach in a system called NextiaJD, and present experiments to show the predictive performance and computational efficiency of our method. Our experiments show that NextiaJD obtains greater predictive performance to that of hash-based methods while we are able to scale-up to larger volumes of data.
更多查看译文
关键词
discovery,join,quality
AI 理解论文
溯源树
样例
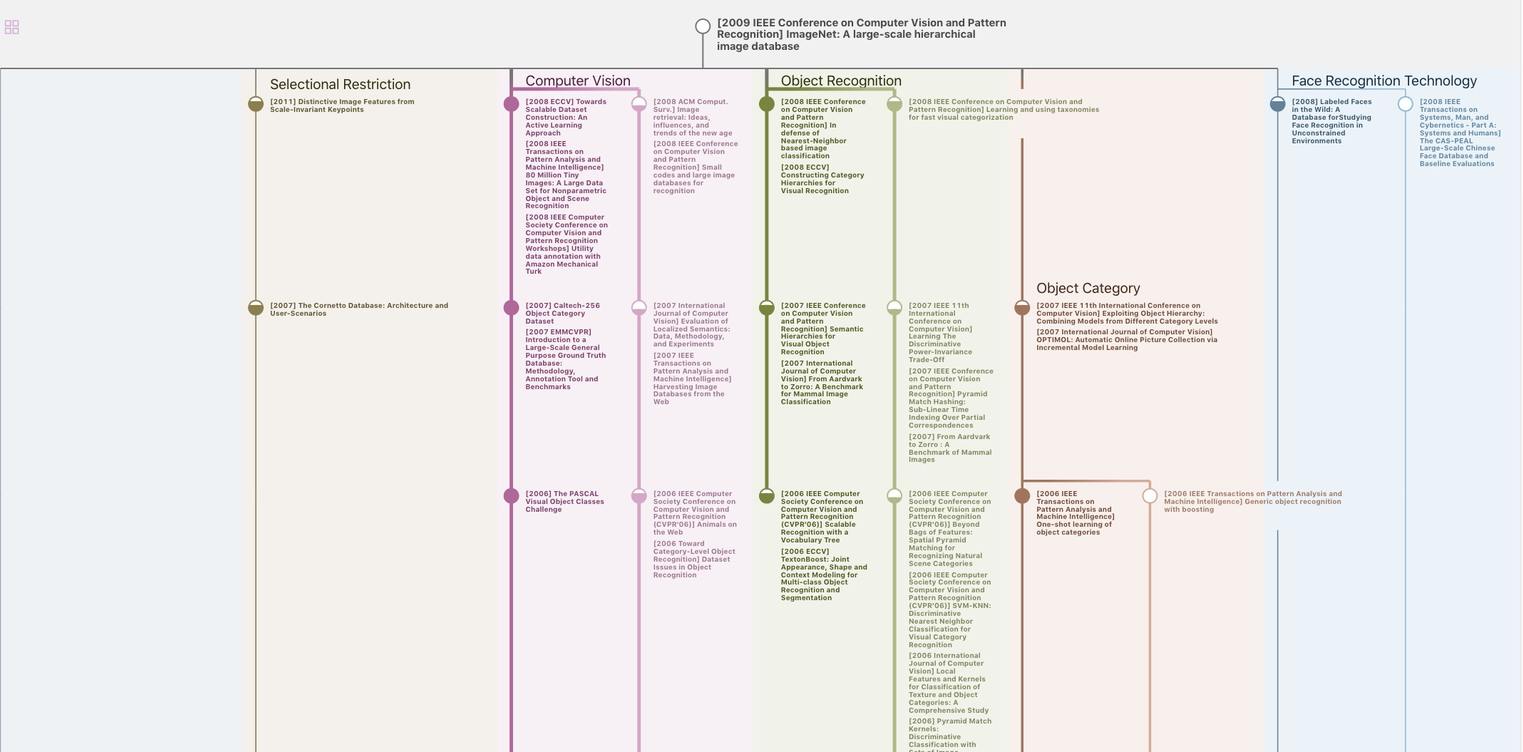
生成溯源树,研究论文发展脉络
Chat Paper
正在生成论文摘要