DyGen: Learning from Noisy Labels via Dynamics-Enhanced Generative Modeling
PROCEEDINGS OF THE 29TH ACM SIGKDD CONFERENCE ON KNOWLEDGE DISCOVERY AND DATA MINING, KDD 2023(2023)
摘要
Learning from noisy labels is a challenge that arises in many real-world applications where training data can contain incorrect or corrupted labels. When fine-tuning language models with noisy labels, models can easily overfit the label noise, leading to decreased performance. Most existing methods for learning from noisy labels use static input features for denoising, but these methods are limited by the information they can provide on true label distributions and can result in biased or incorrect predictions. In this work, we propose the Dynamics-Enhanced Generative Model (DyGen), which uses dynamic patterns in the embedding space during the fine-tuning process of language models to improve noisy label predictions. DyGen uses the variational auto-encoding framework to infer the posterior distributions of true labels from noisy labels and training dynamics. Additionally, a co-regularization mechanism is used to minimize the impact of potentially noisy labels and priors. DyGen demonstrates an average accuracy improvement of 3.10% on two synthetic noise datasets and 1.48% on three real-world noise datasets compared to the previous state-of-the-art. Extensive experiments and analyses show the effectiveness of each component in DyGen. Our code is available for reproducibility on GitHub.
更多查看译文
关键词
Noisy Label Learning,Training Dynamics,Generative Modeling
AI 理解论文
溯源树
样例
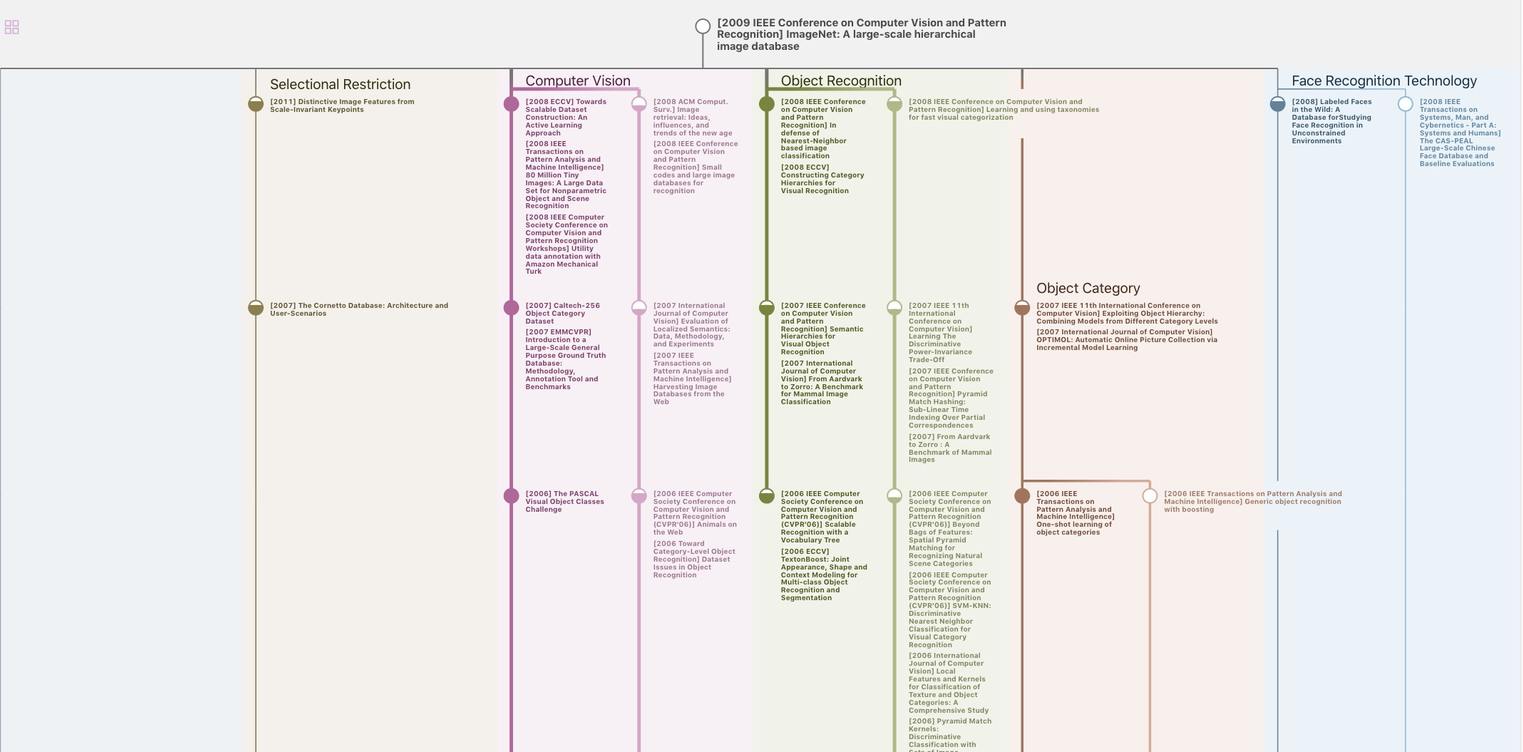
生成溯源树,研究论文发展脉络
Chat Paper
正在生成论文摘要