TrueDeep: A systematic approach of crack detection with less data
EXPERT SYSTEMS WITH APPLICATIONS(2024)
摘要
Supervised and semi-supervised semantic segmentation algorithms require significant amount of annotated data to achieve a good performance. In many situations, the data is either not available or the annotation is expensive. The objective of this work is to show that by incorporating domain knowledge along with deep learning architectures, we can achieve similar performance with less data. We have used publicly available crack segmentation datasets and shown that selecting the input images using knowledge can significantly boost the performance of deep-learning based architectures. Our proposed approaches have many fold advantages such as low annotation and training cost, and less energy consumption. We have measured the performance of our algorithm quantitatively in terms of mean intersection over union (mIoU) and F-score. Our representative model, developed with 23% of the overall data; surpasses the baseline model (>1.5% F-score gain) on the test data while matching the benchmark performance and, with a suitable augmentation strategy, outperforms the baseline model (11.5% average F-score gain) and the benchmark (>8.75% average F-score gain) over the selected blind datasets.
更多查看译文
关键词
Cracks,Semantic segmentation,Deep learning,Computer vision,Image processing
AI 理解论文
溯源树
样例
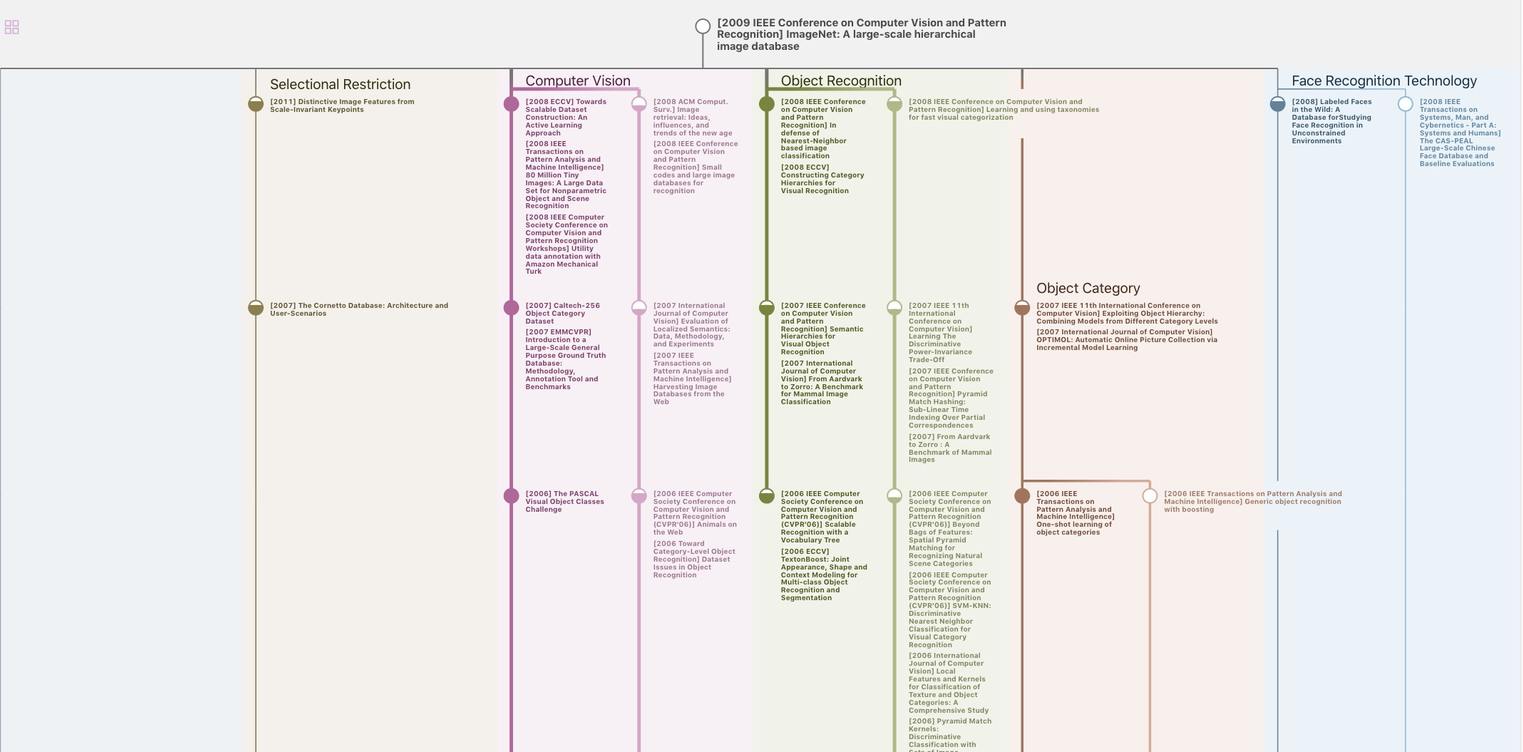
生成溯源树,研究论文发展脉络
Chat Paper
正在生成论文摘要