Investigating model performance in language identification: beyond simple error statistics
CoRR(2023)
摘要
Language development experts need tools that can automatically identify languages from fluent, conversational speech, and provide reliable estimates of usage rates at the level of an individual recording. However, language identification systems are typically evaluated on metrics such as equal error rate and balanced accuracy, applied at the level of an entire speech corpus. These overview metrics do not provide information about model performance at the level of individual speakers, recordings, or units of speech with different linguistic characteristics. Overview statistics may therefore mask systematic errors in model performance for some subsets of the data, and consequently, have worse performance on data derived from some subsets of human speakers, creating a kind of algorithmic bias. In the current paper, we investigate how well a number of language identification systems perform on individual recordings and speech units with different linguistic properties in the MERLIon CCS Challenge. The Challenge dataset features accented English-Mandarin code-switched child-directed speech.
更多查看译文
关键词
language identification,model performance,simple error statistics
AI 理解论文
溯源树
样例
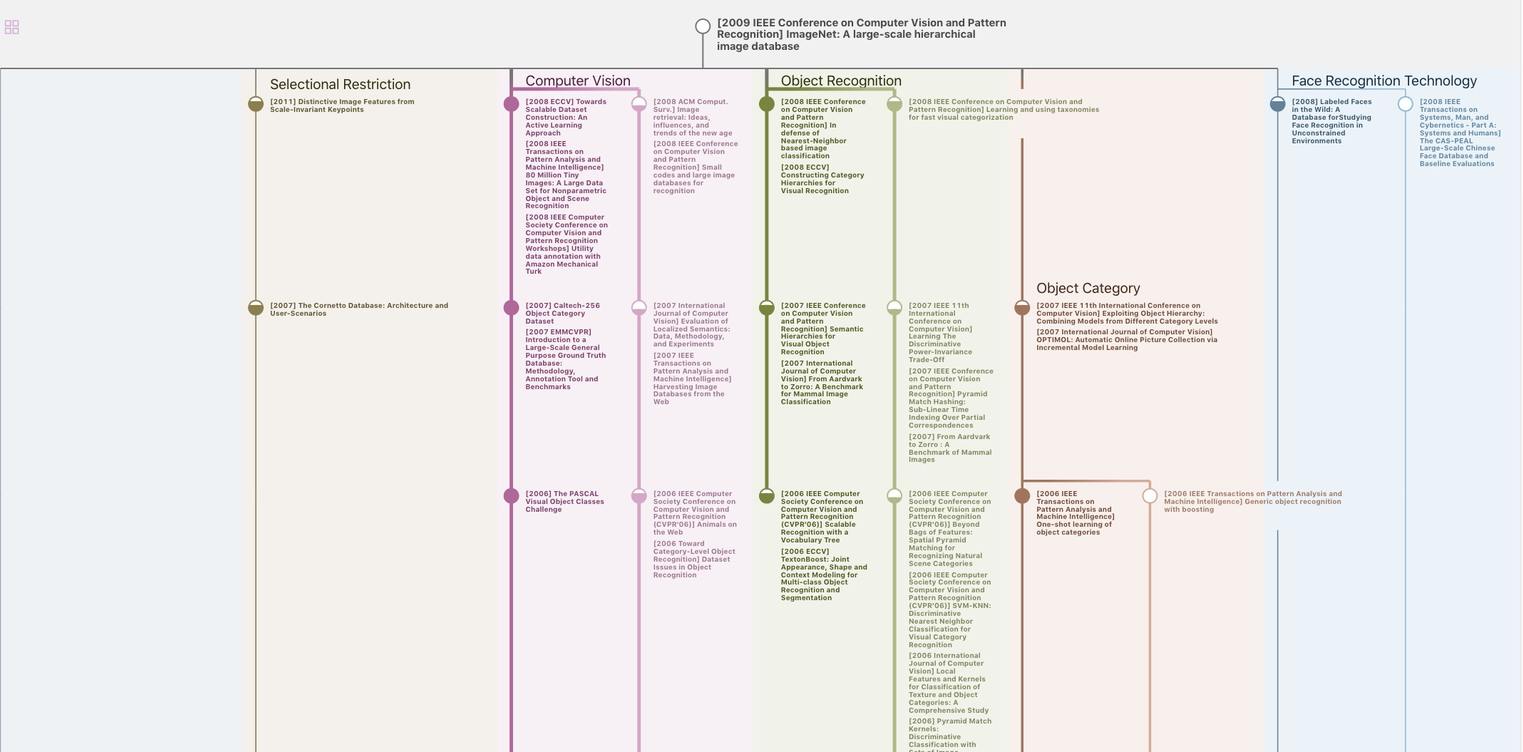
生成溯源树,研究论文发展脉络
Chat Paper
正在生成论文摘要