A General Framework for Learning-Augmented Online Allocation
CoRR(2023)
摘要
Online allocation is a broad class of problems where items arriving online have to be allocated to agents who have a fixed utility/cost for each assigned item so to maximize/minimize some objective. This framework captures a broad range of fundamental problems such as the Santa Claus problem (maximizing minimum utility), Nash welfare maximization (maximizing geometric mean of utilities), makespan minimization (minimizing maximum cost), minimization of $\ell_p$-norms, and so on. We focus on divisible items (i.e., fractional allocations) in this paper. Even for divisible items, these problems are characterized by strong super-constant lower bounds in the classical worst-case online model. In this paper, we study online allocations in the {\em learning-augmented} setting, i.e., where the algorithm has access to some additional (machine-learned) information about the problem instance. We introduce a {\em general} algorithmic framework for learning-augmented online allocation that produces nearly optimal solutions for this broad range of maximization and minimization objectives using only a single learned parameter for every agent. As corollaries of our general framework, we improve prior results of Lattanzi et al. (SODA 2020) and Li and Xian (ICML 2021) for learning-augmented makespan minimization, and obtain the first learning-augmented nearly-optimal algorithms for the other objectives such as Santa Claus, Nash welfare, $\ell_p$-minimization, etc. We also give tight bounds on the resilience of our algorithms to errors in the learned parameters, and study the learnability of these parameters.
更多查看译文
关键词
learning-augmented
AI 理解论文
溯源树
样例
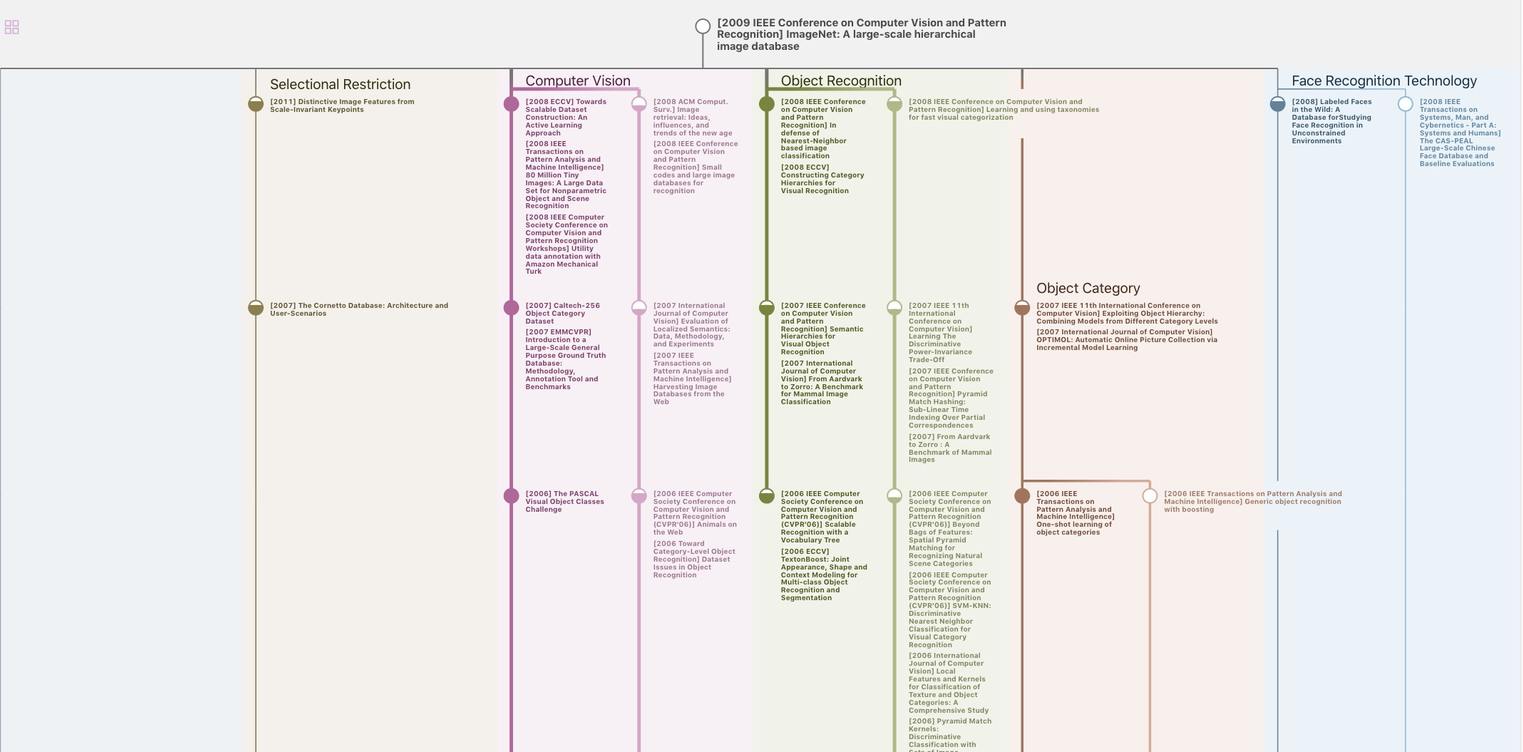
生成溯源树,研究论文发展脉络
Chat Paper
正在生成论文摘要