Generating Behaviorally Diverse Policies with Latent Diffusion Models
arXiv (Cornell University)(2023)
摘要
Recent progress in Quality Diversity Reinforcement Learning (QD-RL) has enabled learning a collection of behaviorally diverse, high performing policies. However, these methods typically involve storing thousands of policies, which results in high space-complexity and poor scaling to additional behaviors. Condensing the archive into a single model while retaining the performance and coverage of the original collection of policies has proved challenging. In this work, we propose using diffusion models to distill the archive into a single generative model over policy parameters. We show that our method achieves a compression ratio of 13x while recovering 98% of the original rewards and 89% of the original coverage. Further, the conditioning mechanism of diffusion models allows for flexibly selecting and sequencing behaviors, including using language. Project website: https://sites.google.com/view/policydiffusion/home
更多查看译文
关键词
diverse policies,diffusion,models
AI 理解论文
溯源树
样例
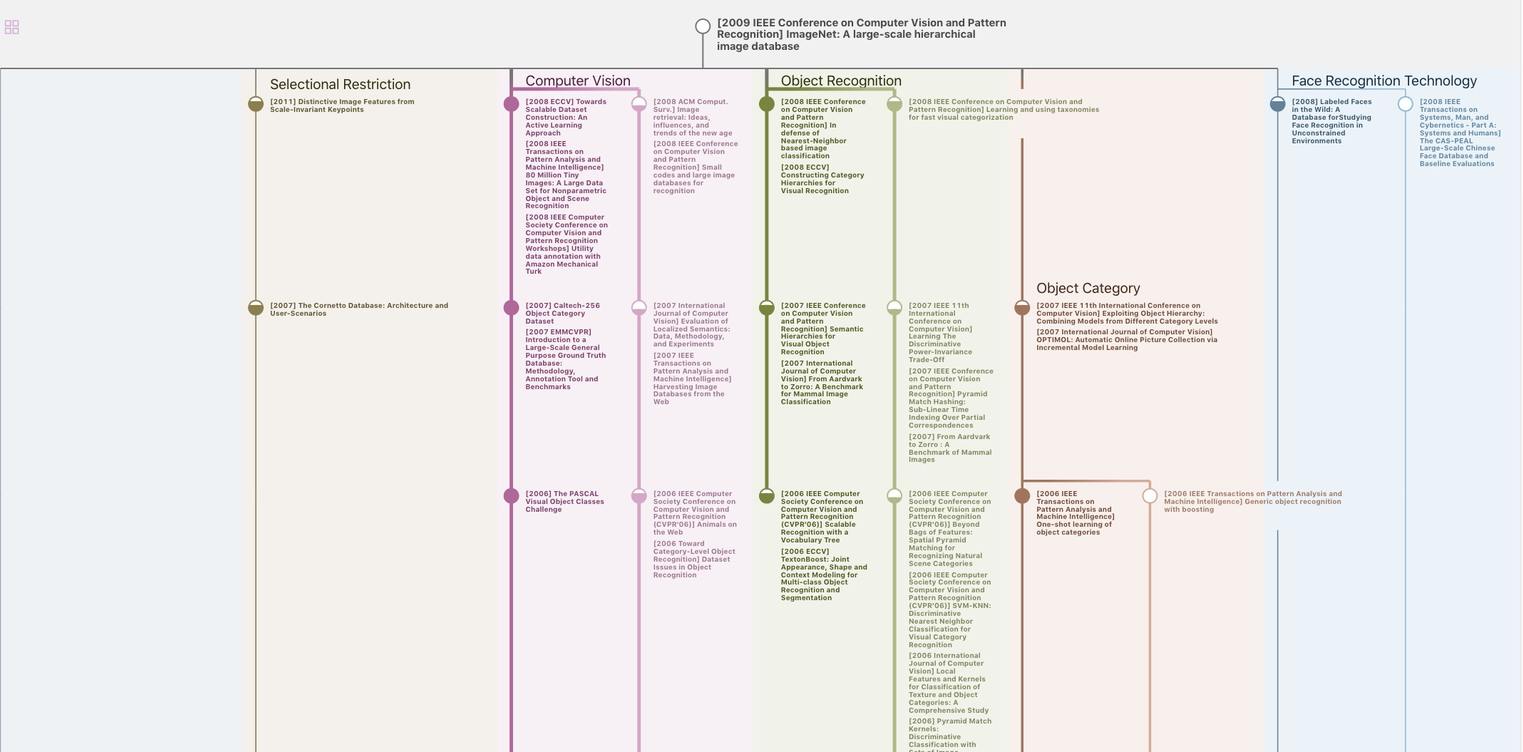
生成溯源树,研究论文发展脉络
Chat Paper
正在生成论文摘要