Deep neural networks generalization and fine-tuning for 12-lead ECG classification
BIOMEDICAL SIGNAL PROCESSING AND CONTROL(2024)
摘要
Numerous studies focus on diagnosing heart diseases using deep learning methods applied to 12-lead electrocardiographic (ECG) records. However, these studies often face limitations due to reliance on specific datasets, which vary in size and parameters such as patient metadata, the number of doctors annotating ECGs, types of devices used for ECG recording, data preprocessing techniques, etc. It is well-known that high-quality deep neural networks trained on one ECG dataset may not necessarily perform well on other datasets or in different clinical settings. In this paper, we propose a methodology designed to enhance the quality of heart disease prediction regardless of the dataset. We achieve this by first training neural networks on a variety of labeled datasets, then fine-tuning for specific datasets, significantly improving prediction accuracy. We demonstrate its applicability by training various neural networks on a large private dataset TIS, which contains a wide range of ECG records from multiple hospitals, and on a relatively smaller public dataset, PTB-XL. Our results show that networks trained on a large dataset improves classification performance. Furthermore, these networks fine-tuned on PTB-XL outperform those trained exclusively on smaller datasets. Additionally, we made the weights of our pre-trained models publicly available, enabling researchers and clinicians to adapt these models to their specific datasets.
更多查看译文
关键词
Electrocardiography,Time series analysis,Deep learning,ECG classification,Fine-tuning,Pre -trained neural networks
AI 理解论文
溯源树
样例
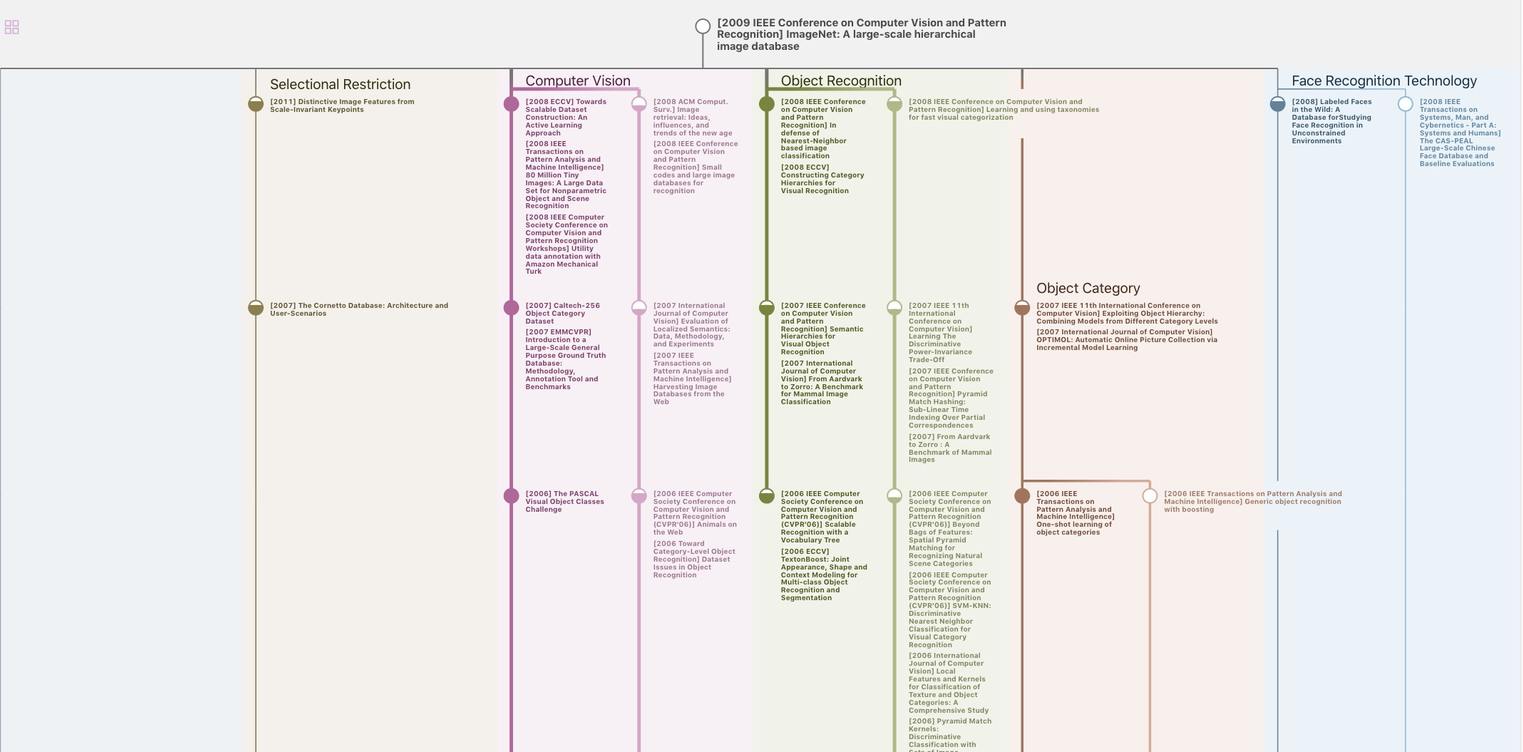
生成溯源树,研究论文发展脉络
Chat Paper
正在生成论文摘要