Autoencoding Conditional Neural Processes for Representation Learning
CoRR(2023)
摘要
Conditional neural processes (CNPs) are a flexible and efficient family of models that learn to learn a stochastic process from observations. In the visual domain, they have seen particular application in contextual image completion - observing pixel values at some locations to predict a distribution over values at other unobserved locations. However, the choice of pixels in learning such a CNP is typically either random or derived from a simple statistical measure (e.g. pixel variance). Here, we turn the problem on its head and ask: which pixels would a CNP like to observe? That is, which pixels allow fitting CNP, and do such pixels tell us something about the underlying image? Viewing the context provided to the CNP as fixed-size latent representations, we construct an amortised variational framework, Partial Pixel Space Variational Autoencoder (PPS-VAE), for predicting this context simultaneously with learning a CNP. We evaluate PPS-VAE on a set of vision datasets, and find that not only is it possible to learn context points while also fitting CNPs, but that their spatial arrangement and values provides strong signal for the information contained in the image - evaluated through the lens of classification. We believe the PPS-VAE provides a promising avenue to explore learning interpretable and effective visual representations.
更多查看译文
关键词
representation learning,conditional neural processes
AI 理解论文
溯源树
样例
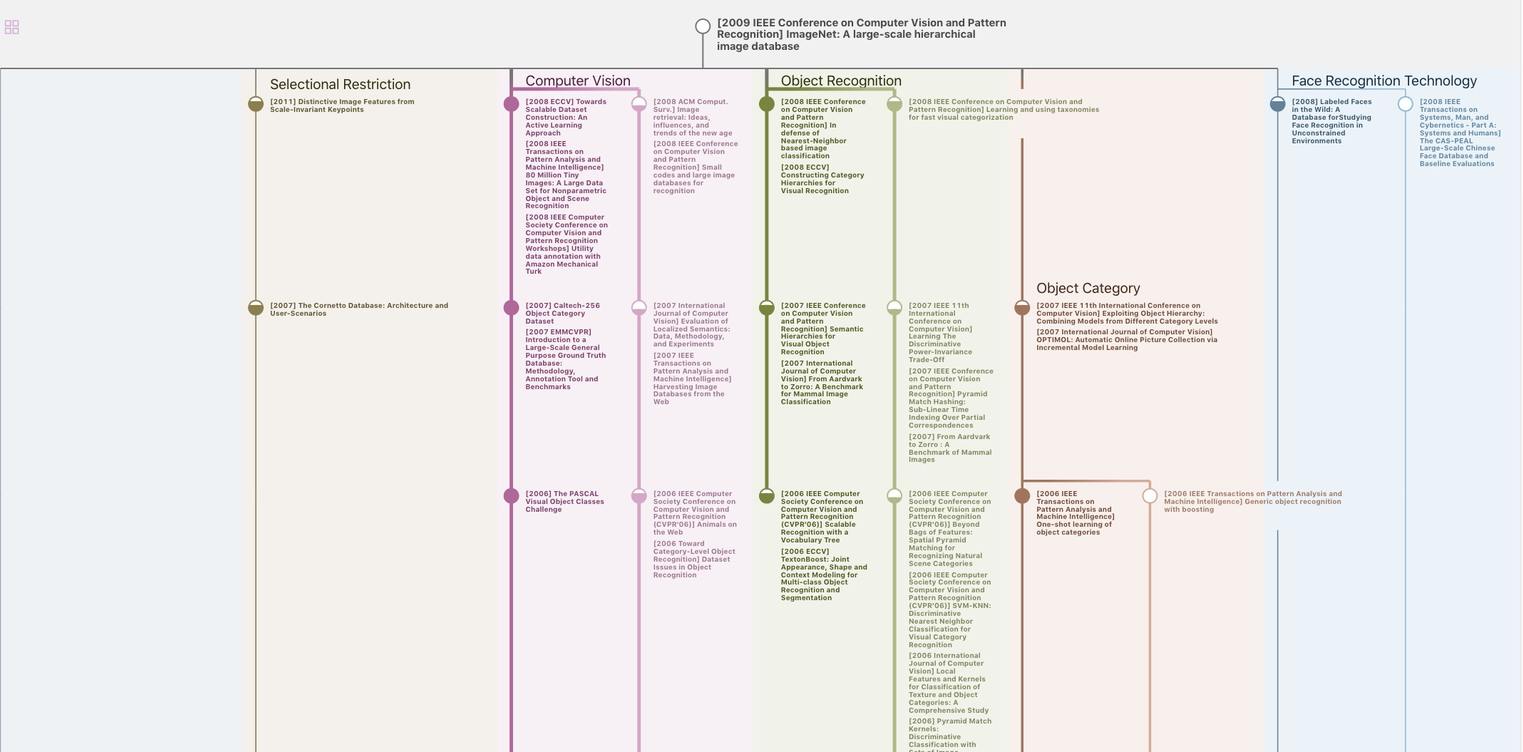
生成溯源树,研究论文发展脉络
Chat Paper
正在生成论文摘要