Neural Network Reduction with Guided Regularizers
CoRR(2023)
摘要
Regularization techniques such as $\mathcal{L}_1$ and $\mathcal{L}_2$ regularizers are effective in sparsifying neural networks (NNs). However, to remove a certain neuron or channel in NNs, all weight elements related to that neuron or channel need to be prunable, which is not guaranteed by traditional regularization. This paper proposes a simple new approach named "Guided Regularization" that prioritizes the weights of certain NN units more than others during training, which renders some of the units less important and thus, prunable. This is different from the scattered sparsification of $\mathcal{L}_1$ and $\mathcal{L}_2$ regularizers where the the components of a weight matrix that are zeroed out can be located anywhere. The proposed approach offers a natural reduction of NN in the sense that a model is being trained while also neutralizing unnecessary units. We empirically demonstrate that our proposed method is effective in pruning NNs while maintaining performance.
更多查看译文
关键词
neural network reduction,neural network
AI 理解论文
溯源树
样例
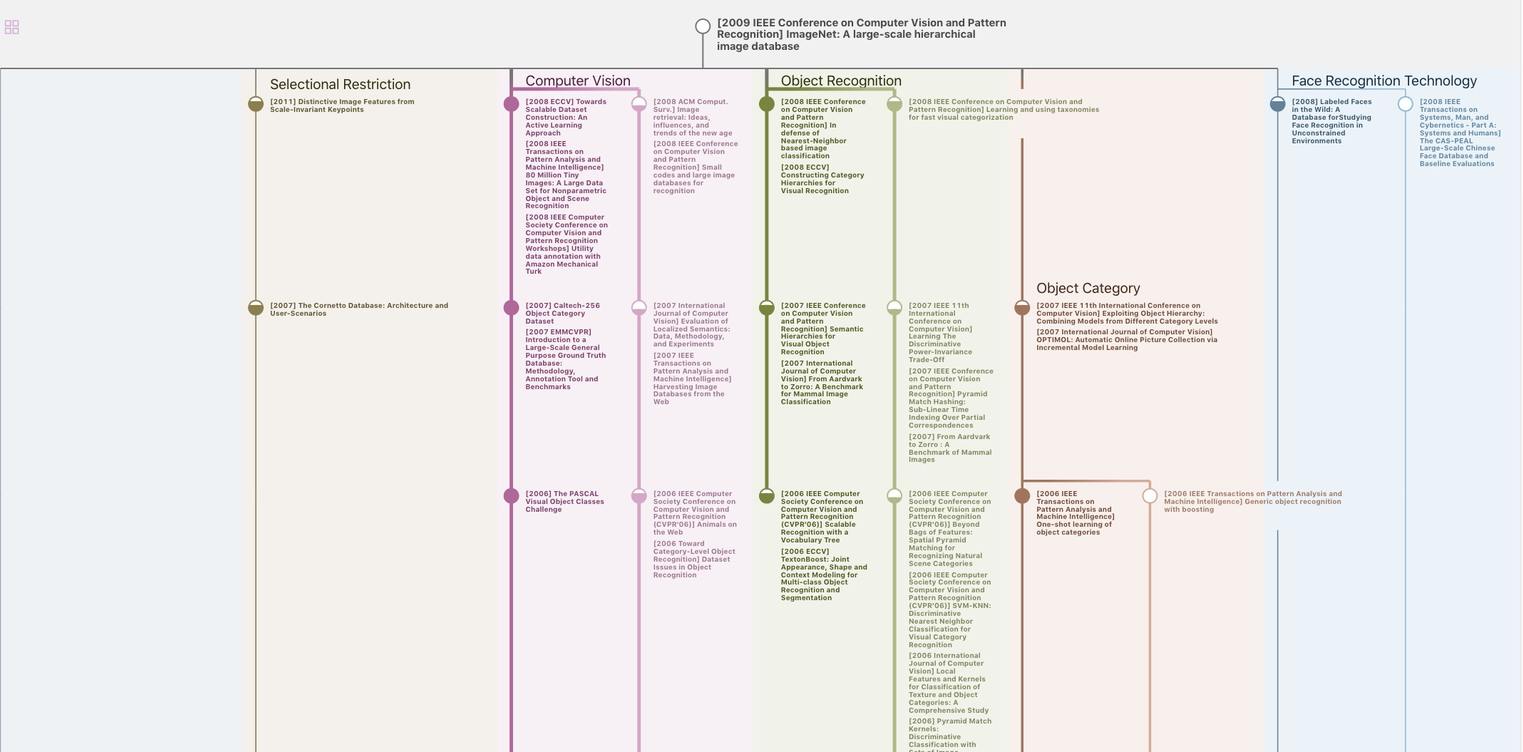
生成溯源树,研究论文发展脉络
Chat Paper
正在生成论文摘要