HyperTime: Hyperparameter Optimization for Combating Temporal Distribution Shifts
CoRR(2023)
摘要
In this work, we propose a hyperparameter optimization method named \emph{HyperTime} to find hyperparameters robust to potential temporal distribution shifts in the unseen test data. Our work is motivated by an important observation that it is, in many cases, possible to achieve temporally robust predictive performance via hyperparameter optimization. Based on this observation, we leverage the `worst-case-oriented' philosophy from the robust optimization literature to help find such robust hyperparameter configurations. HyperTime imposes a lexicographic priority order on average validation loss and worst-case validation loss over chronological validation sets. We perform a theoretical analysis on the upper bound of the expected test loss, which reveals the unique advantages of our approach. We also demonstrate the strong empirical performance of the proposed method on multiple machine learning tasks with temporal distribution shifts.
更多查看译文
关键词
hyperparameter optimization,shifts
AI 理解论文
溯源树
样例
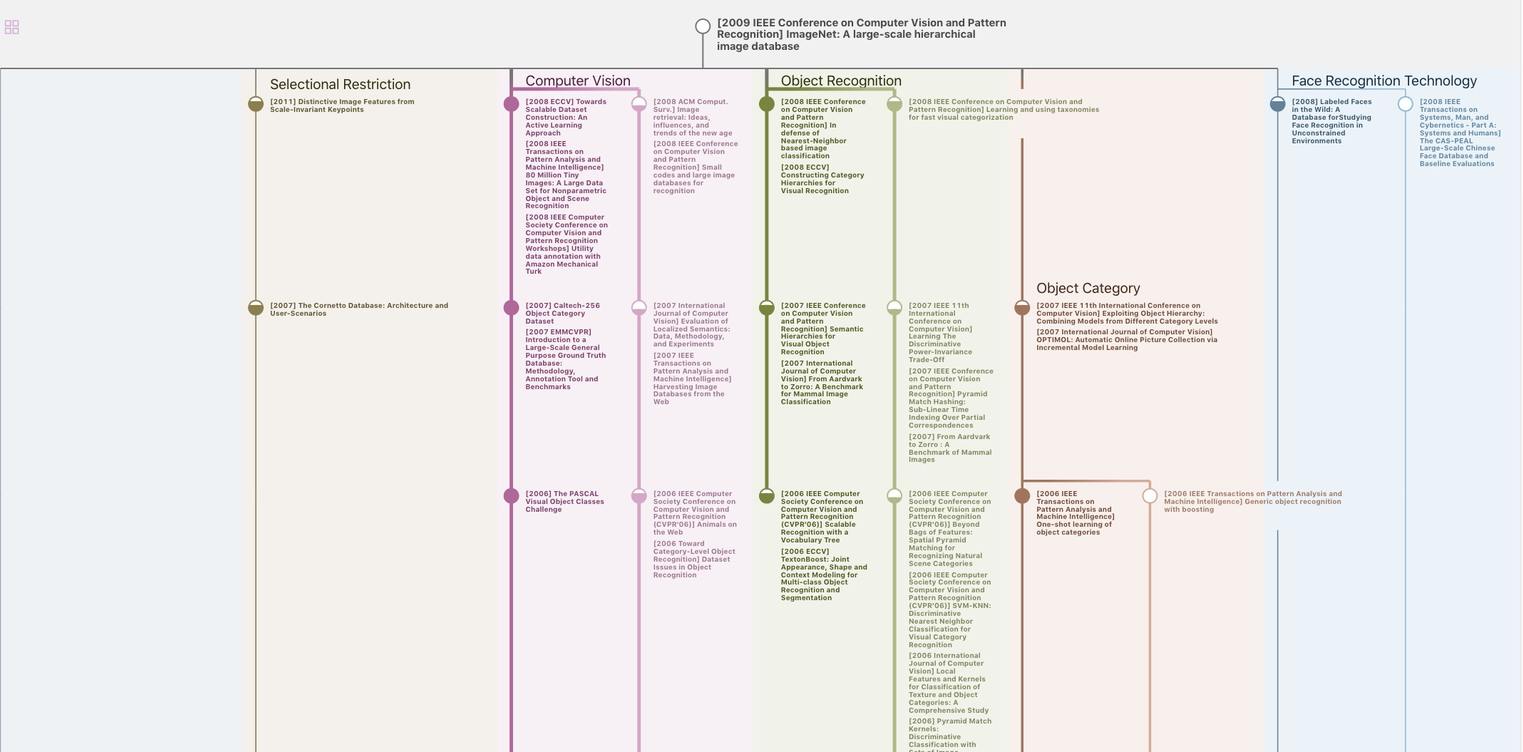
生成溯源树,研究论文发展脉络
Chat Paper
正在生成论文摘要