The Statistical Benefits of Quantile Temporal-Difference Learning for Value Estimation
CoRR(2023)
摘要
We study the problem of temporal-difference-based policy evaluation in reinforcement learning. In particular, we analyse the use of a distributional reinforcement learning algorithm, quantile temporal-difference learning (QTD), for this task. We reach the surprising conclusion that even if a practitioner has no interest in the return distribution beyond the mean, QTD (which learns predictions about the full distribution of returns) may offer performance superior to approaches such as classical TD learning, which predict only the mean return, even in the tabular setting.
更多查看译文
关键词
learning,value,estimation,temporal-difference
AI 理解论文
溯源树
样例
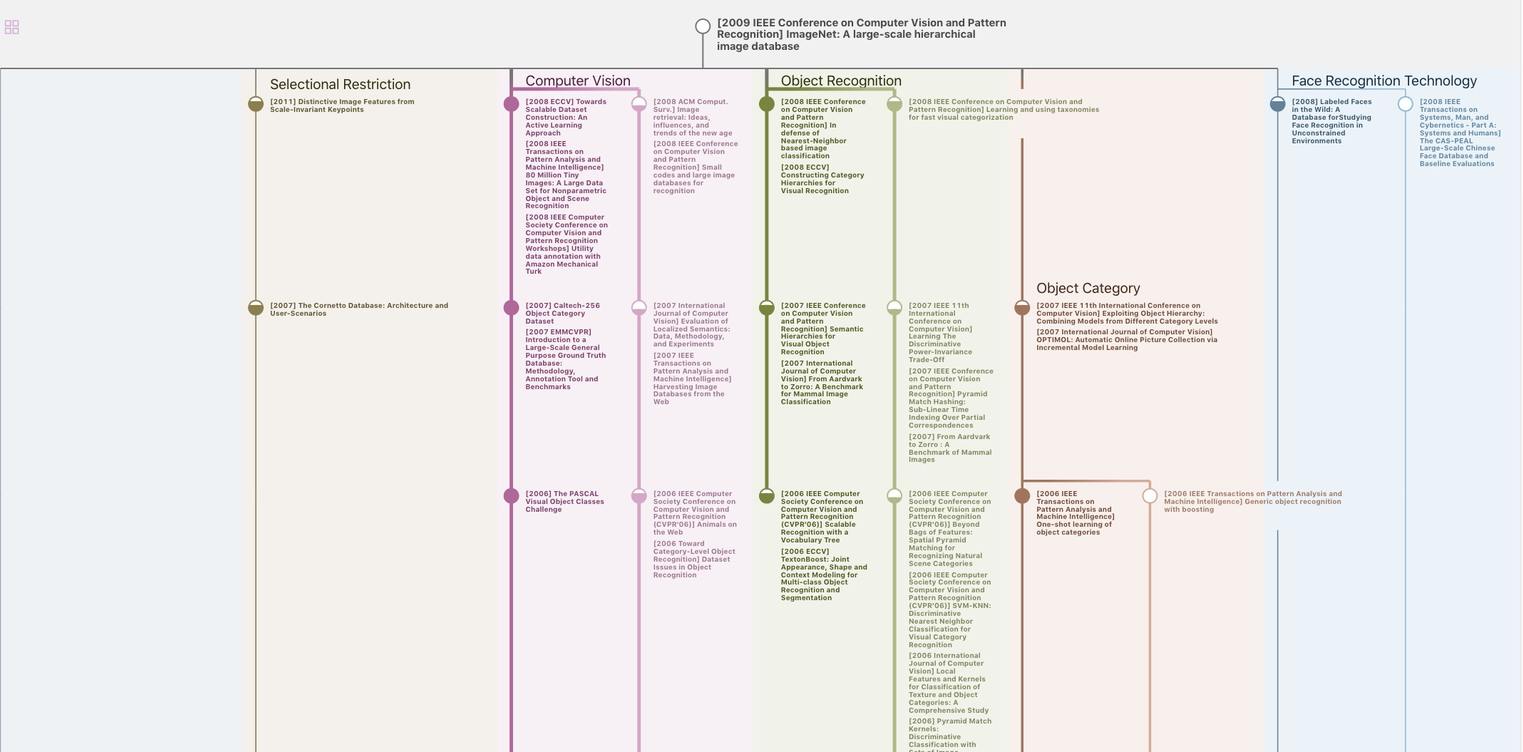
生成溯源树,研究论文发展脉络
Chat Paper
正在生成论文摘要