Prediction of Overall Patient Characteristics that Incorporate Multiple Outcomes in Acute Stroke: Latent Class Analysis
medRxiv (Cold Spring Harbor Laboratory)(2023)
摘要
Background: Previous prediction models have predicted a single outcome (e.g. gait) from several patient characteristics at one point (e.g. on admission). However, in clinical practice, it is important to predict an overall patient characteristic by incorporating multiple outcomes. This study aimed to develop a prediction model of overall patient characteristics in acute stroke patients using latent class analysis.Methods: This retrospective observational study analyzed stroke patients admitted to acute care hospitals (37 hospitals, N=10,270) between January 2005 and March 2016 from the Japan Association of Rehabilitation Database. Overall, 6,881 patients were classified into latent classes based on their outcomes. The prediction model was developed based on patient characteristics and functional ability at admission. We selected the following outcome variables at discharge for classification using latent class analysis: Functional Independence Measure (functional abilities and cognitive functions), subscales of the National Institutes of Health Stroke Scale (upper extremity function), length of hospital stay, and discharge destination. The predictor variables were age, Functional Independence Measure (functional abilities and comprehension), subscales of the National Institutes of Health Stroke Scale (upper extremity function), stroke type, and amount of rehabilitation (physical, occupational, and speech therapies) per day during hospitalization.Results: Patients (N=6,881) were classified into nine classes based on latent class analysis regarding patient characteristics at discharge (class size: 429%). Class 1 was the mildest (shorter stay and highest possibilities of home discharge), and Class 2 was the most severe (longer stay and the highest possibility of transfers inckuding deaths). Different gradations characterized Classes 39; these patient characteristics were clinically acceptable. Predictor variables at admission that predicted class membership were significant (odds ratio: 0.0107.9, P<.001).Conclusions: Based on these findings, the model developed in this study could predict an overall patient characteristic combining multiple outcomes, including the appropriate rehabilitation intensity. In actual clinical practice, internal and external validation is required.
### Competing Interest Statement
The authors have declared no competing interest.
### Funding Statement
This work was supported by JSPS KAKENHI (Grant number: JP20H03914).
### Author Declarations
I confirm all relevant ethical guidelines have been followed, and any necessary IRB and/or ethics committee approvals have been obtained.
Not Applicable
The details of the IRB/oversight body that provided approval or exemption for the research described are given below:
The study was approved by the ethics committee of the Kanagawa University of Human Services (No. 7-20-30).
I confirm that all necessary patient/participant consent has been obtained and the appropriate institutional forms have been archived, and that any patient/participant/sample identifiers included were not known to anyone (e.g., hospital staff, patients or participants themselves) outside the research group so cannot be used to identify individuals.
Not Applicable
I understand that all clinical trials and any other prospective interventional studies must be registered with an ICMJE-approved registry, such as ClinicalTrials.gov. I confirm that any such study reported in the manuscript has been registered and the trial registration ID is provided (note: if posting a prospective study registered retrospectively, please provide a statement in the trial ID field explaining why the study was not registered in advance).
Not Applicable
I have followed all appropriate research reporting guidelines, such as any relevant EQUATOR Network research reporting checklist(s) and other pertinent material, if applicable.
Not Applicable
The datasets presented in this article are not readily available because of the confidential data provided by the Japan Association of Rehabilitation Database. Therefore, this data could not be shared.
更多查看译文
关键词
acute stroke,latent class analysis,overall patient characteristics,incorporate multiple outcomes
AI 理解论文
溯源树
样例
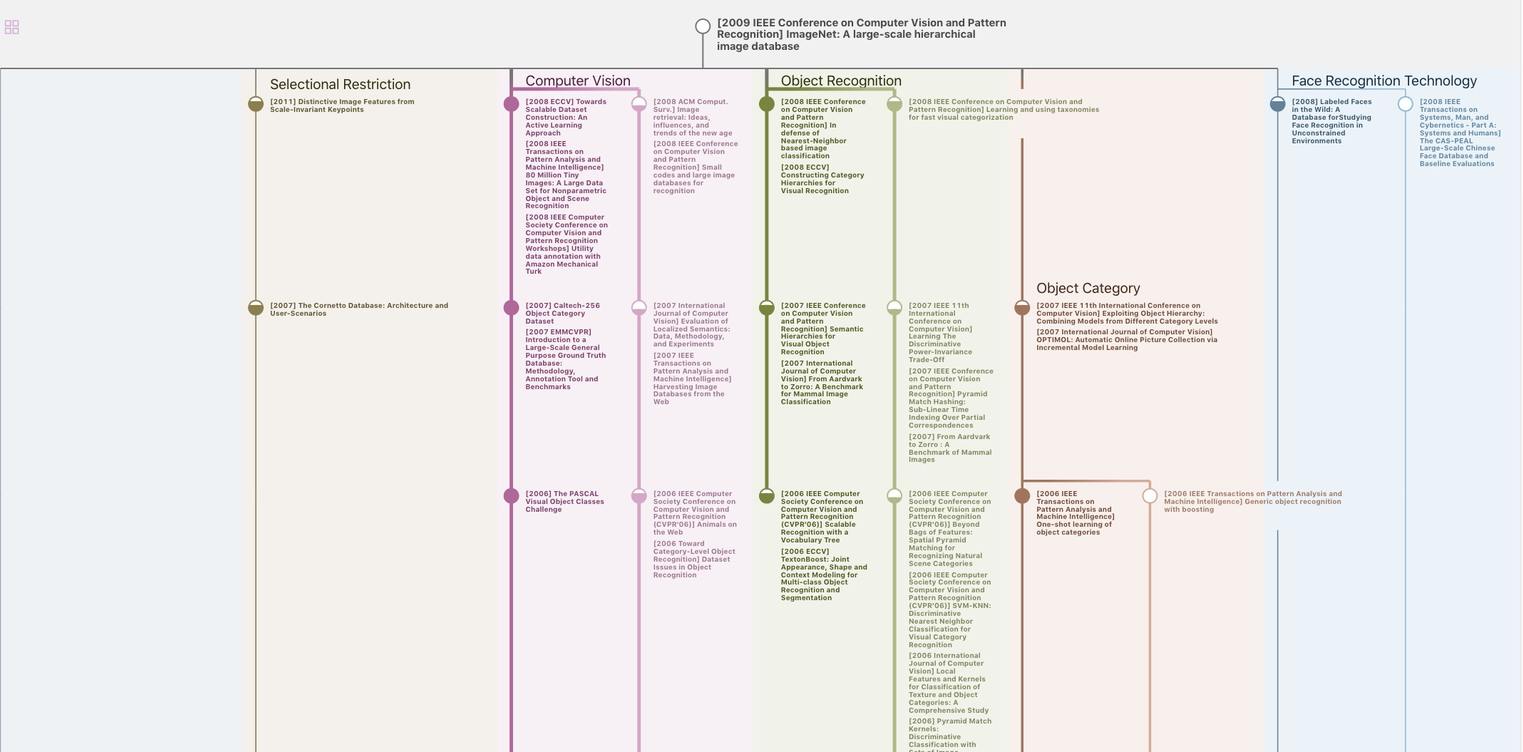
生成溯源树,研究论文发展脉络
Chat Paper
正在生成论文摘要