Efficient Interactive Recommendation via Huffman Tree-based Policy Learning
AAMAS '23: Proceedings of the 2023 International Conference on Autonomous Agents and Multiagent Systems(2023)
摘要
Interactive recommender systems~(IRSs) are an essential part of our daily life, as they can suggest items to persistently satisfy our demands. Due to the interactive nature, conventional static recommendation methods such as matrix factorization, and content-based filtering are ineffective to capture the dynamic preferences of users. Recently, reinforcement learning(RL) has shown great potential in addressing the challenges in IRSs, since it can capture users' dynamic preferences and model the long-term profit of user-item interactions. However, millions of items in real-world IRSs lead to a large discrete action space in the RL setting, rendering RL-based IRSs inefficient and hindering their widespread application. Such an inefficiency issue has not been well addressed in the literature. In order to address this issue, we propose a novel Huffman Tree Policy Recommendation~(HTPR) framework. Specifically, a novel policy learning network based on a newly designed Huffman tree is proposed for policy representation learning, which effectively improves the learning efficiency. Moreover, a novel parameter-sharing scheme is devised to further reduce unnecessary computations. Extensive experiments on two real-world benchmark datasets demonstrate the superiority of HTPR over the state-of-the-art IRS methods in terms of both recommendation accuracy and efficiency.
更多查看译文
AI 理解论文
溯源树
样例
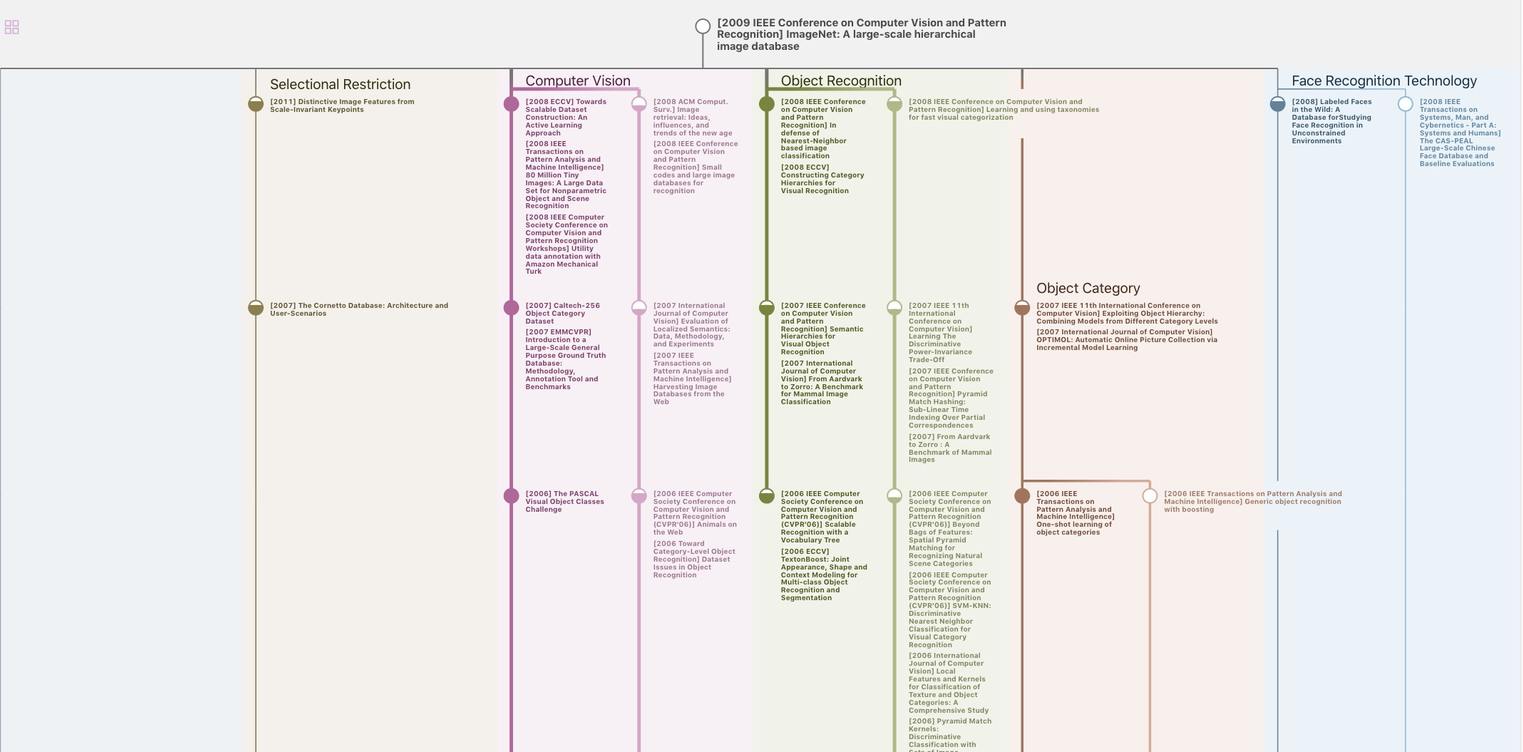
生成溯源树,研究论文发展脉络
Chat Paper
正在生成论文摘要