Learning Solutions in Large Economic Networks using Deep Multi-Agent Reinforcement Learning
AAMAS '23: Proceedings of the 2023 International Conference on Autonomous Agents and Multiagent Systems(2023)
摘要
Real-world economies can be modeled as a network with many heterogeneous and strategic agents. In this setting, it is very challenging to find optimal mechanisms, e.g., taxes, 1) when taking strategic best responses into account and 2) even when using restrictive assumptions, e.g., that supply always meets demand. Deep multi-agent reinforcement learning (MARL) is a natural framework to learn mechanisms and model strategic best responses, but independent MARL often collapses to trivial solutions (e.g., where nobody works) as joint exploration severely distorts rewards and constraints. Here, we show how to use structured learning curricula and GPU-accelerated simulations to find non-trivial solutions in networks with many heterogeneous agents. We validate our approach in models with 100 worker-consumers, 10 firms, and a social planner who taxes and redistributes. We use empirical best-response analyses across agent types to show that it is difficult for agents to benefit by deviating from the learned solutions. In particular, we find income and corporate taxes that achieve 15% higher social welfare compared to baselines.
更多查看译文
AI 理解论文
溯源树
样例
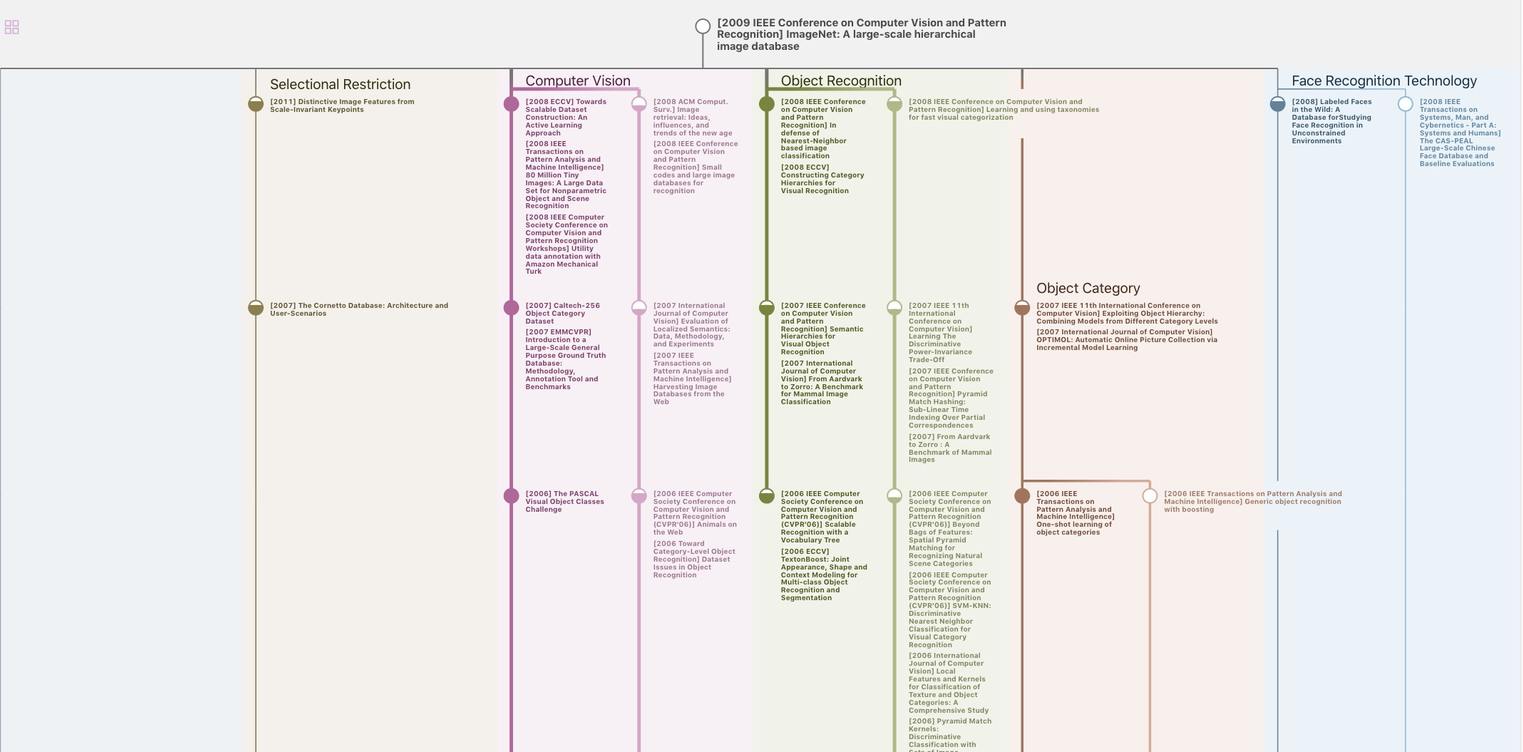
生成溯源树,研究论文发展脉络
Chat Paper
正在生成论文摘要