Counterfactually Fair Dynamic Assignment: A Case Study on Policing
AAMAS '23: Proceedings of the 2023 International Conference on Autonomous Agents and Multiagent Systems(2023)
摘要
Resource assignment algorithms for decision-making in dynamic environments have been shown to sometimes lead to negative impacts on individuals from minority populations. We propose a framework for algorithmic assignment of scarce resources in a dynamic setting that seeks to minimize concerns around unfairness and the potential for runaway feedback loops that create injustices. Our model estimates an underlying true latent confounder in a biased dataset, and makes allocation decisions based on a notion of fair intervention. We present evidence for the plausibility of our model by analyzing a novel dataset obtained from the City of Chicago through FOIA requests, and plan to release this dataset along with a visualization tool for use by various stakeholders. We also show that, in a simulated environment, our counterfactually fair policy can allocate limited resources near optimally, and better than baseline alternatives.
更多查看译文
AI 理解论文
溯源树
样例
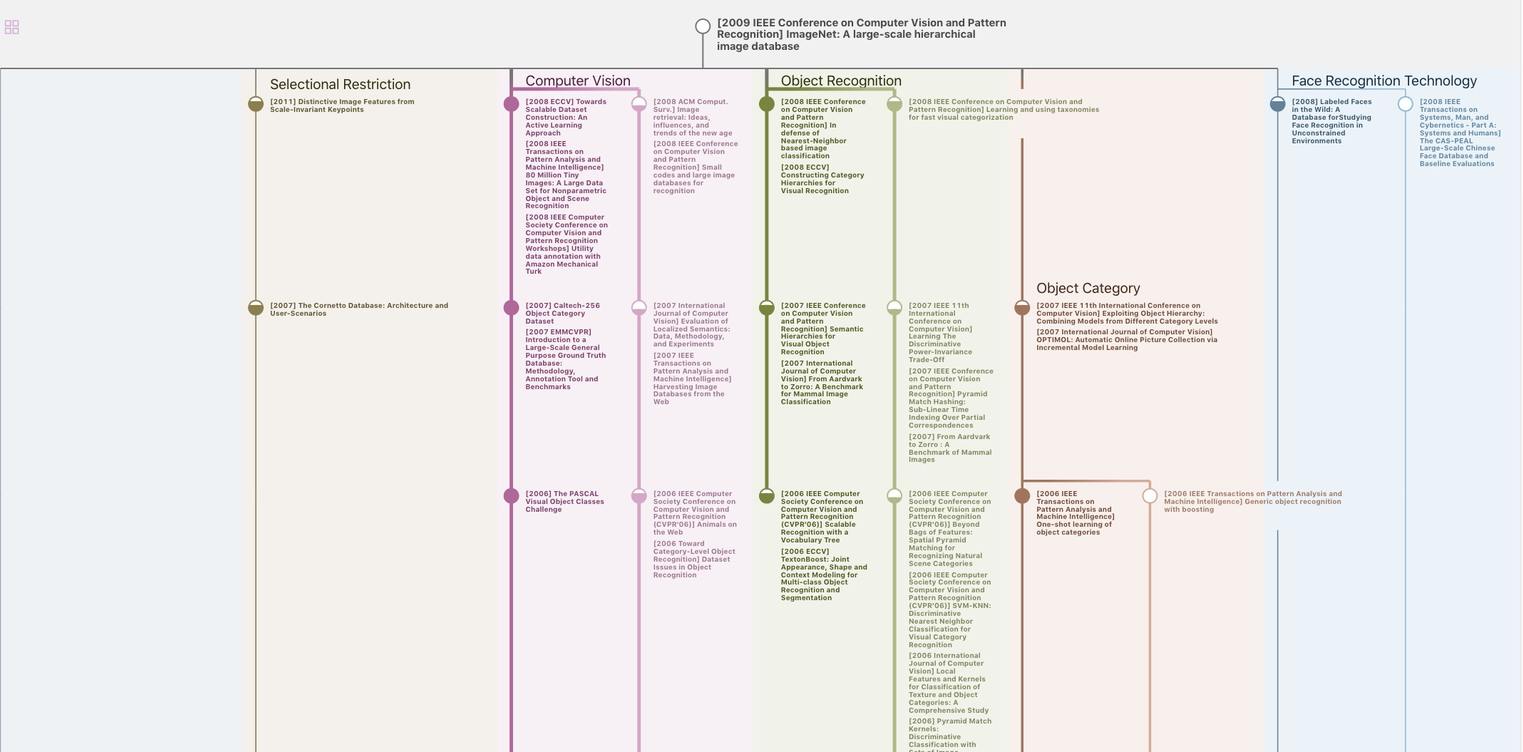
生成溯源树,研究论文发展脉络
Chat Paper
正在生成论文摘要