Learning Properties in Simulation-Based Games
AAMAS '23: Proceedings of the 2023 International Conference on Autonomous Agents and Multiagent Systems(2023)
摘要
Empirical game-theoretic analysis (EGTA) is primarily concerned with learning equilibria of simulation-based games. Recent approaches have tackled this problem by learning a uniform approximation of the game's utilities, and then applying precision-recall theorems: i.e., all equilibria of the true game are approximate equilibria in the estimated game, and vice-versa. In this work, we generalize this approach to all game properties that are well-behaved (i.e., Lipschitz continuous in utilities), including regret (which defines Nash and correlated equilibria), adversarial values, power-mean welfare, and Gini social welfare. We show that, given a well-behaved welfare function, while optimal welfare is well-behaved, the welfare of optimal (i.e., welfare-maximizing or minimizing) equilibria is not well behaved. We thus define a related property based on a Lagrangian relaxation of the equilibrium constraints that is well behaved. We call this property Łambda-stable welfare. As determining the welfare of an optimal equilibrium is an essential step in computing the price of anarchy, we conclude with a discussion of an alternative, more stable notion of anarchy based on lambda-stable welfare, which we call the anarchy gap.
更多查看译文
AI 理解论文
溯源树
样例
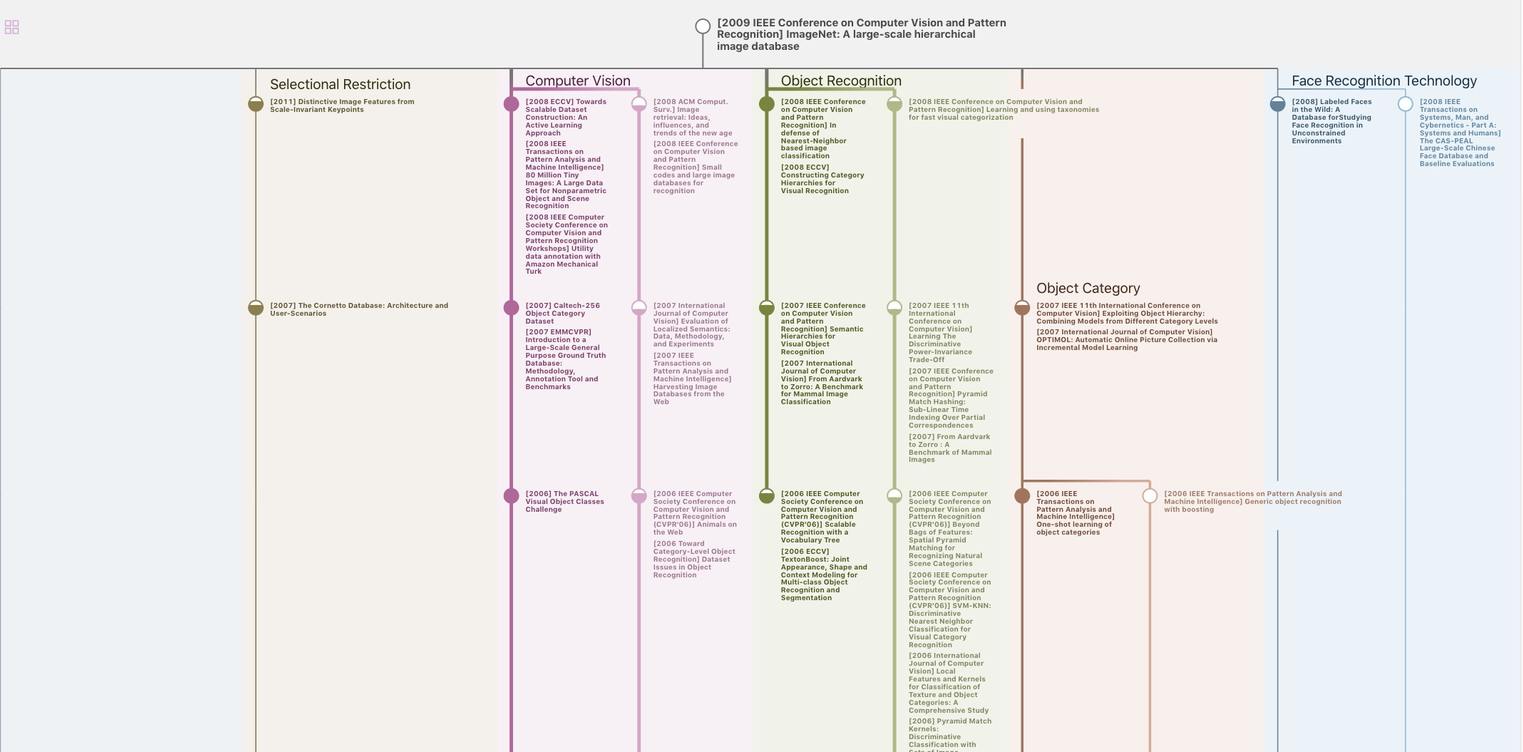
生成溯源树,研究论文发展脉络
Chat Paper
正在生成论文摘要