CamoDiffusion: Camouflaged Object Detection via Conditional Diffusion Models
AAAI 2024(2024)
摘要
Camouflaged Object Detection (COD) is a challenging task in computer vision due to the high similarity between camouflaged objects and their surroundings. Existing COD methods struggle with nuanced object boundaries and overconfident incorrect predictions. In response, we propose a new paradigm that treats COD as a conditional mask-generation task leveraging diffusion models. Our method, dubbed CamoDiffusion, employs the denoising process to progressively refine predictions while incorporating image conditions. Due to the stochastic sampling process of diffusion, our model is capable of sampling multiple possible predictions, avoiding the problem of overconfident point estimation. Moreover, we develop specialized network architecture, training, and sampling strategies, to enhance the model’s expressive power, refinement capabilities and suppress overconfident mis-segmentations, thus aptly tailoring the diffusion model to the demands of COD. Extensive experiments on three COD datasets attest to the superior performance of our model compared to existing state-of-the-art methods, particularly on the most challenging COD10K dataset, where our approach achieves 0.019 in terms of MAE. Codes and models are available at https://github.com/Rapisurazurite/CamoDiffusion.
更多查看译文
关键词
CV: Segmentation
AI 理解论文
溯源树
样例
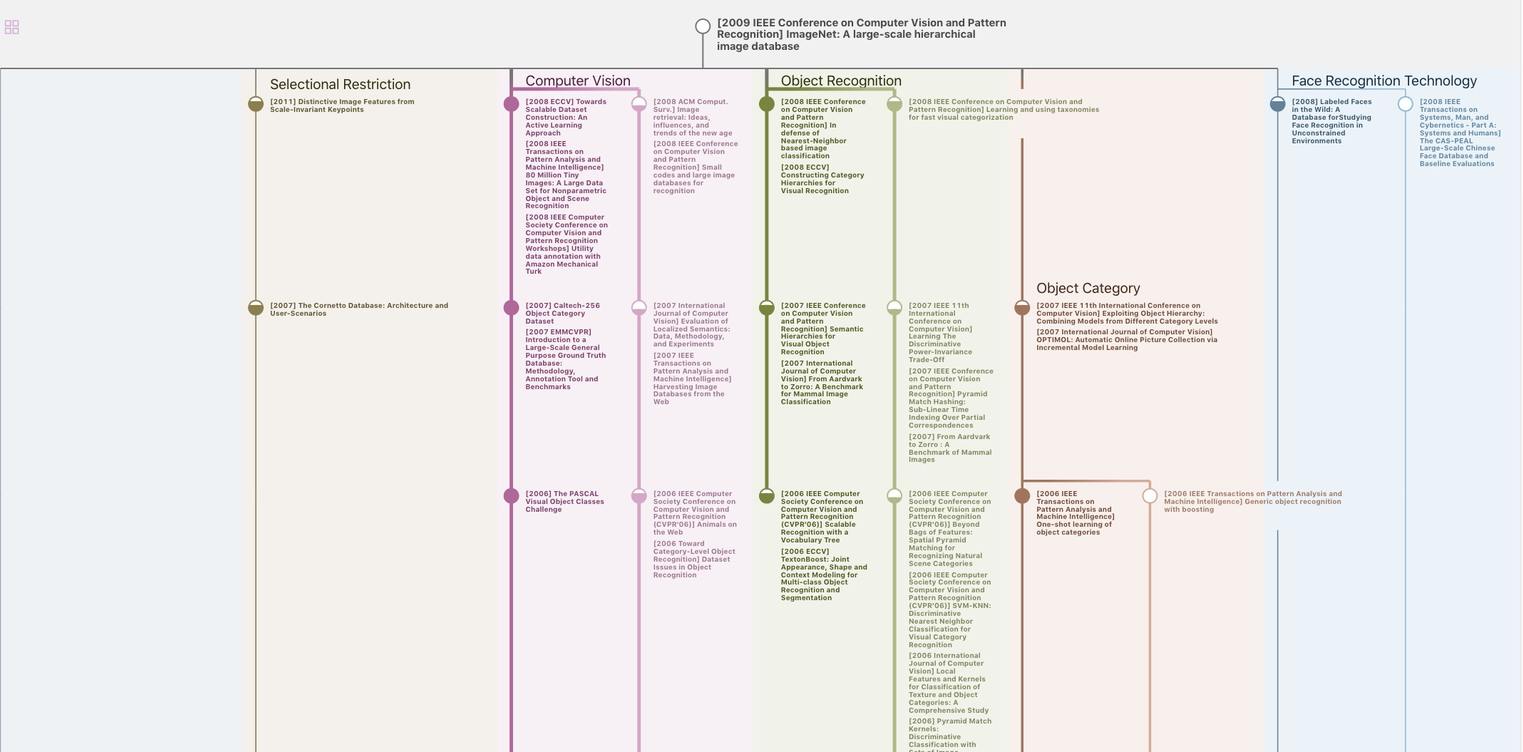
生成溯源树,研究论文发展脉络
Chat Paper
正在生成论文摘要