Active Learning of Deterministic Timed Automata with Myhill-Nerode Style Characterization
CoRR(2023)
摘要
We present an algorithm to learn a deterministic timed automaton (DTA) via membership and equivalence queries. Our algorithm is an extension of the L* algorithm with a Myhill-Nerode style characterization of recognizable timed languages, which is the class of timed languages recognizable by DTAs. We first characterize the recognizable timed languages with a Nerode-style congruence. Using it, we give an algorithm with a smart teacher answering symbolic membership queries in addition to membership and equivalence queries. With a symbolic membership query, one can ask the membership of a certain set of timed words at one time. We prove that for any recognizable timed language, our learning algorithm returns a DTA recognizing it. We show how to answer a symbolic membership query with finitely many membership queries. We also show that our learning algorithm requires a polynomial number of queries with a smart teacher and an exponential number of queries with a normal teacher. We applied our algorithm to various benchmarks and confirmed its effectiveness with a normal teacher.
更多查看译文
关键词
deterministic timed automata,myhill-nerode
AI 理解论文
溯源树
样例
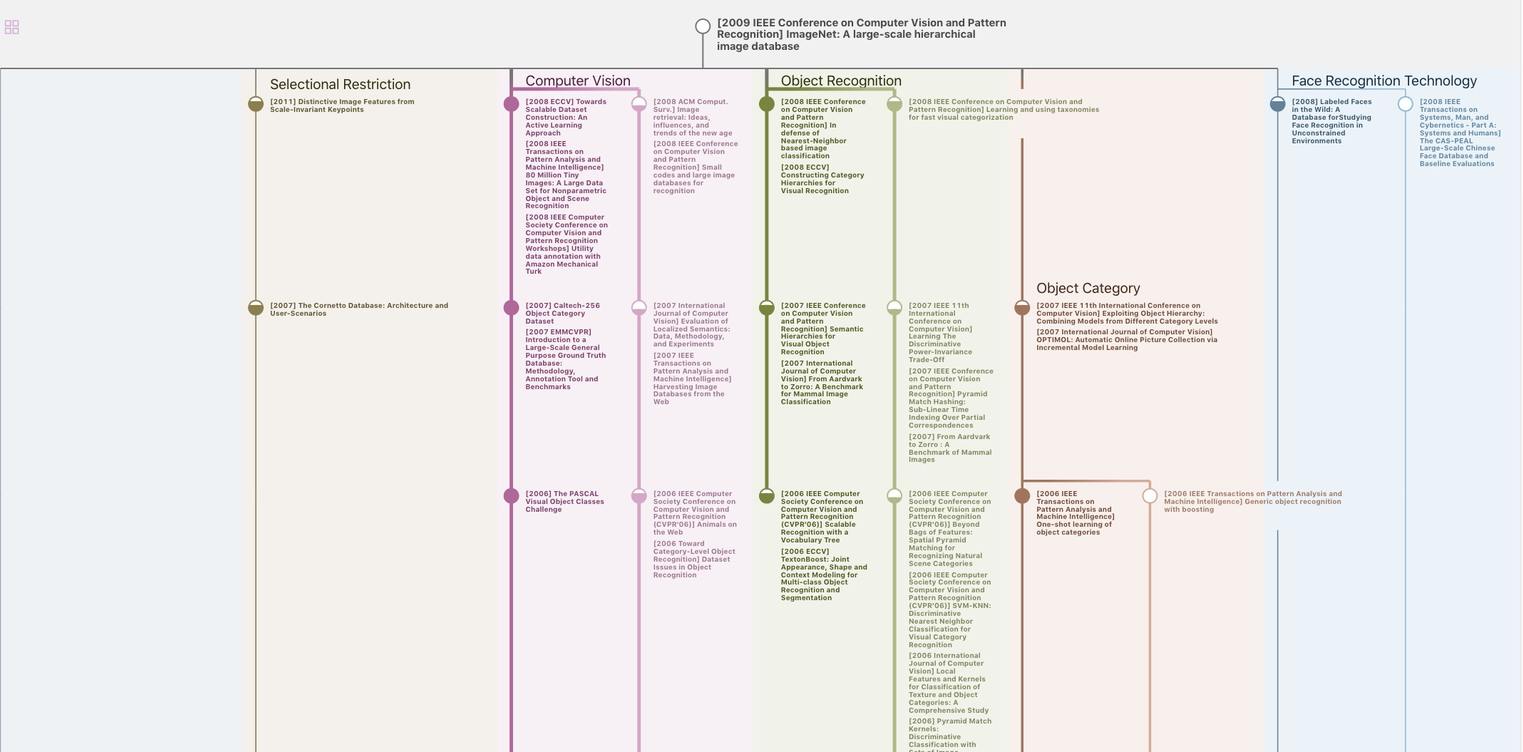
生成溯源树,研究论文发展脉络
Chat Paper
正在生成论文摘要