StyleS2ST: Zero-shot Style Transfer for Direct Speech-to-speech Translation
CoRR(2023)
摘要
Direct speech-to-speech translation (S2ST) has gradually become popular as it has many advantages compared with cascade S2ST. However, current research mainly focuses on the accuracy of semantic translation and ignores the speech style transfer from a source language to a target language. The lack of high-fidelity expressive parallel data makes such style transfer challenging, especially in more practical zero-shot scenarios. To solve this problem, we first build a parallel corpus using a multi-lingual multi-speaker text-to-speech synthesis (TTS) system and then propose the StyleS2ST model with cross-lingual speech style transfer ability based on a style adaptor on a direct S2ST system framework. Enabling continuous style space modeling of an acoustic model through parallel corpus training and non-parallel TTS data augmentation, StyleS2ST captures cross-lingual acoustic feature mapping from the source to the target language. Experiments show that StyleS2ST achieves good style similarity and naturalness in both in-set and out-of-set zero-shot scenarios.
更多查看译文
关键词
direct,translation,zero-shot,speech-to-speech
AI 理解论文
溯源树
样例
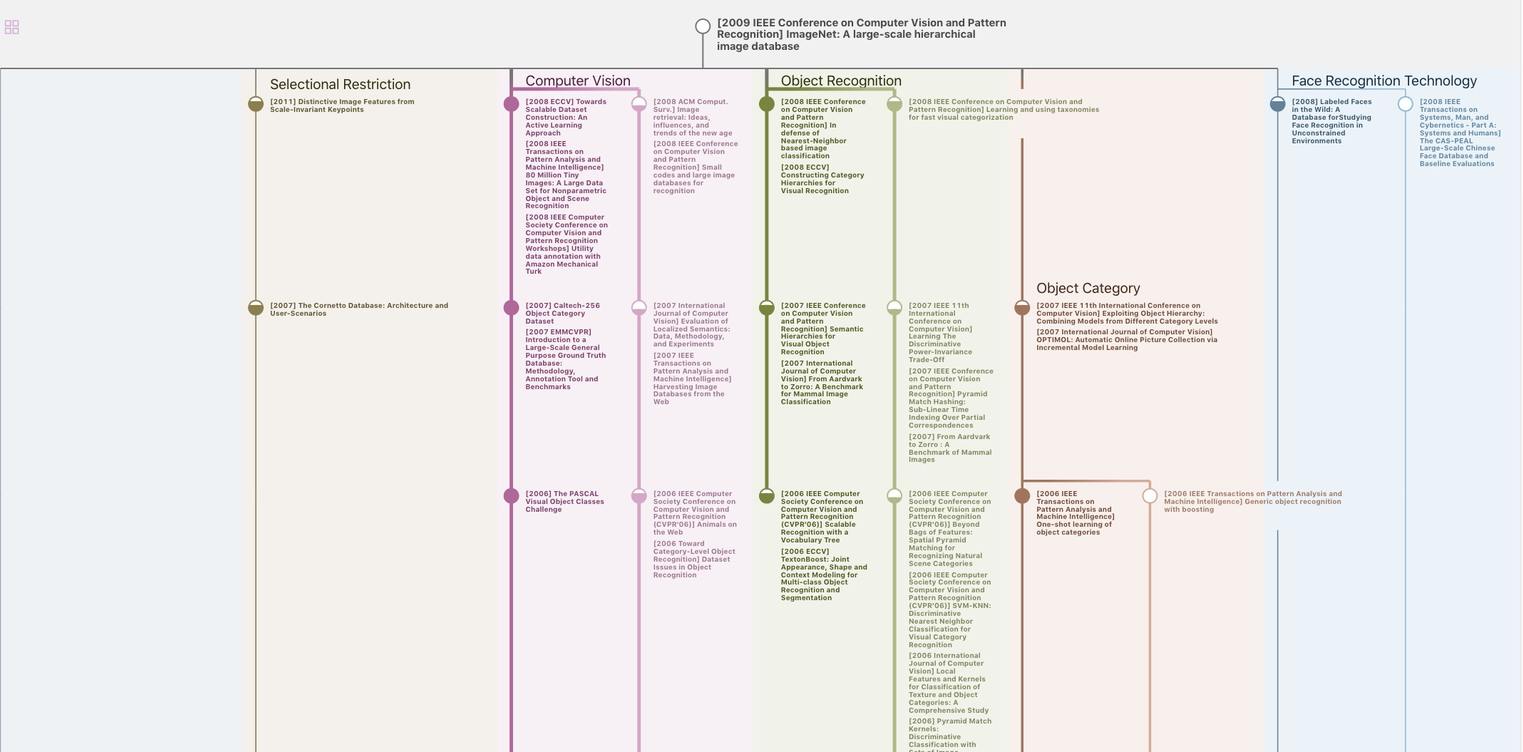
生成溯源树,研究论文发展脉络
Chat Paper
正在生成论文摘要