Rethinking Masked Language Modeling for Chinese Spelling Correction
conf_acl(2023)
摘要
In this paper, we study Chinese Spelling Correction (CSC) as a joint decision made by two separate models: a language model and an error model. Through empirical analysis, we find that fine-tuning BERT tends to over-fit the error model while under-fit the language model, resulting in poor generalization to out-of-distribution error patterns. Given that BERT is the backbone of most CSC models, this phenomenon has a significant negative impact. To address this issue, we are releasing a multi-domain benchmark LEMON, with higher quality and diversity than existing benchmarks, to allow a comprehensive assessment of the open domain generalization of CSC models. Then, we demonstrate that a very simple strategy, randomly masking 20\% non-error tokens from the input sequence during fine-tuning is sufficient for learning a much better language model without sacrificing the error model. This technique can be applied to any model architecture and achieves new state-of-the-art results on SIGHAN, ECSpell, and LEMON.
更多查看译文
关键词
masked language modeling,spelling,chinese,correction
AI 理解论文
溯源树
样例
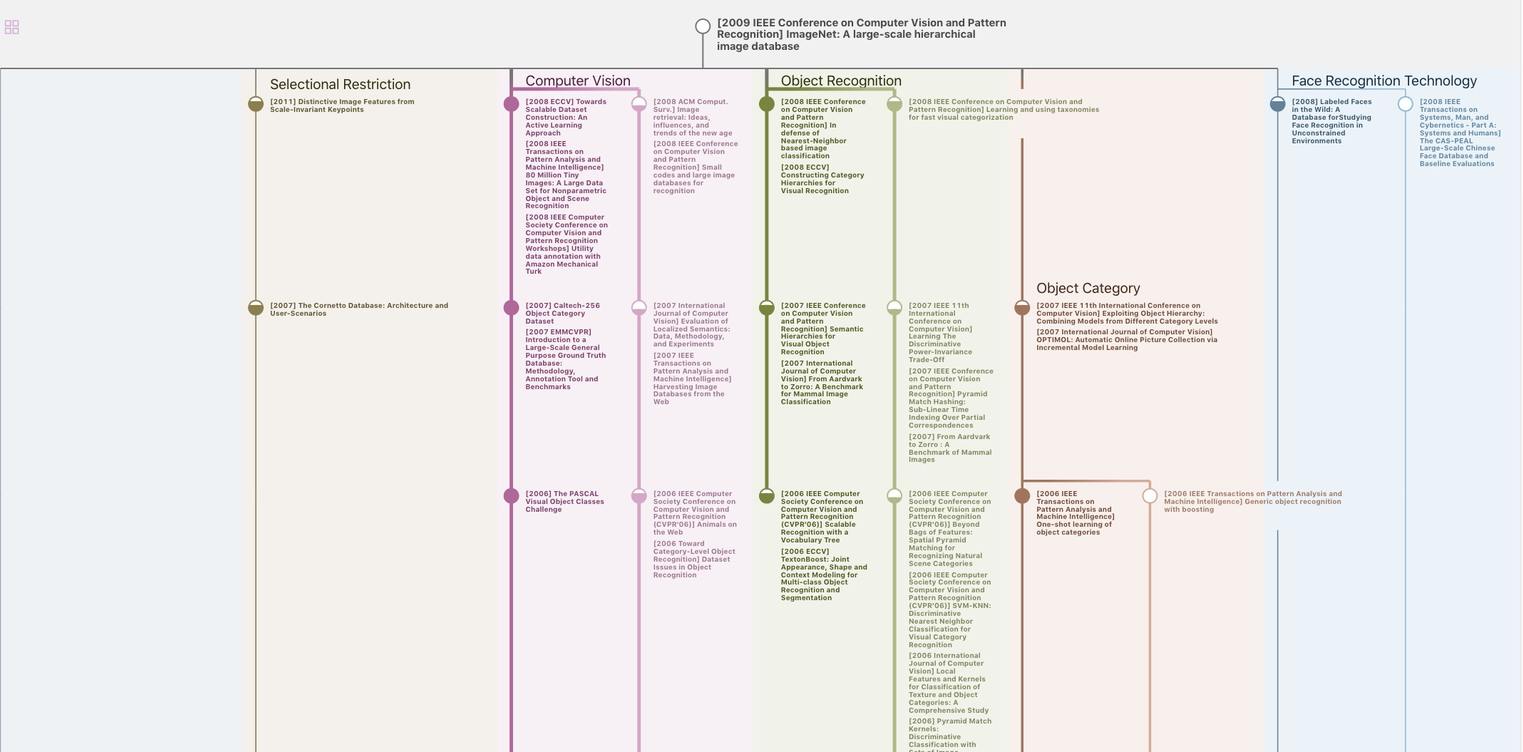
生成溯源树,研究论文发展脉络
Chat Paper
正在生成论文摘要