Adapting Language-Audio Models as Few-Shot Audio Learners
CoRR(2023)
摘要
We presented the Treff adapter, a training-efficient adapter for CLAP, to boost zero-shot classification performance by making use of a small set of labelled data. Specifically, we designed CALM to retrieve the probability distribution of text-audio clips over classes using a set of audio-label pairs and combined it with CLAP's zero-shot classification results. Furthermore, we designed a training-free version of the Treff adapter by using CALM as a cosine similarity measure. Experiments showed that the proposed Treff adapter is comparable and even better than fully-supervised methods and adaptation methods in low-shot and data-abundant scenarios. While the Treff adapter shows that combining large-scale pretraining and rapid learning of domain-specific knowledge is non-trivial for obtaining generic representations for few-shot learning, it is still limited to audio classification tasks. In the future, we will explore how to use audio-language models in diverse audio domains.
更多查看译文
关键词
learners,models,language-audio,few-shot
AI 理解论文
溯源树
样例
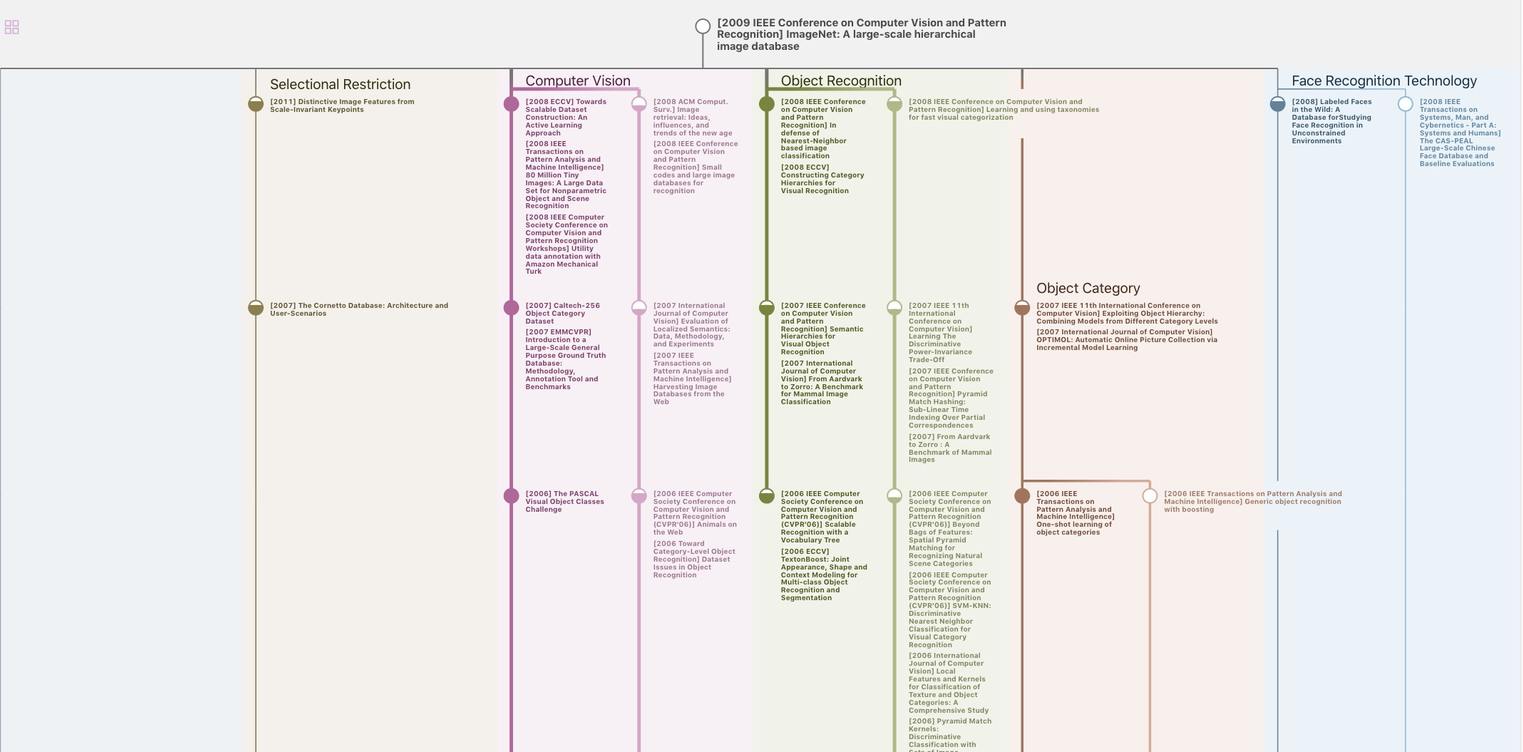
生成溯源树,研究论文发展脉络
Chat Paper
正在生成论文摘要