Detecting Edit Failures In Large Language Models: An Improved Specificity Benchmark
conf_acl(2023)
摘要
Recent model editing techniques promise to mitigate the problem of memorizing false or outdated associations during LLM training. However, we show that these techniques can introduce large unwanted side effects which are not detected by existing specificity benchmarks. We extend the existing CounterFact benchmark to include a dynamic component and dub our benchmark CounterFact+. Additionally, we extend the metrics used for measuring specificity by a principled KL divergence-based metric. We use this improved benchmark to evaluate recent model editing techniques and find that they suffer from low specificity. Our findings highlight the need for improved specificity benchmarks that identify and prevent unwanted side effects.
更多查看译文
关键词
edit failures,large language models
AI 理解论文
溯源树
样例
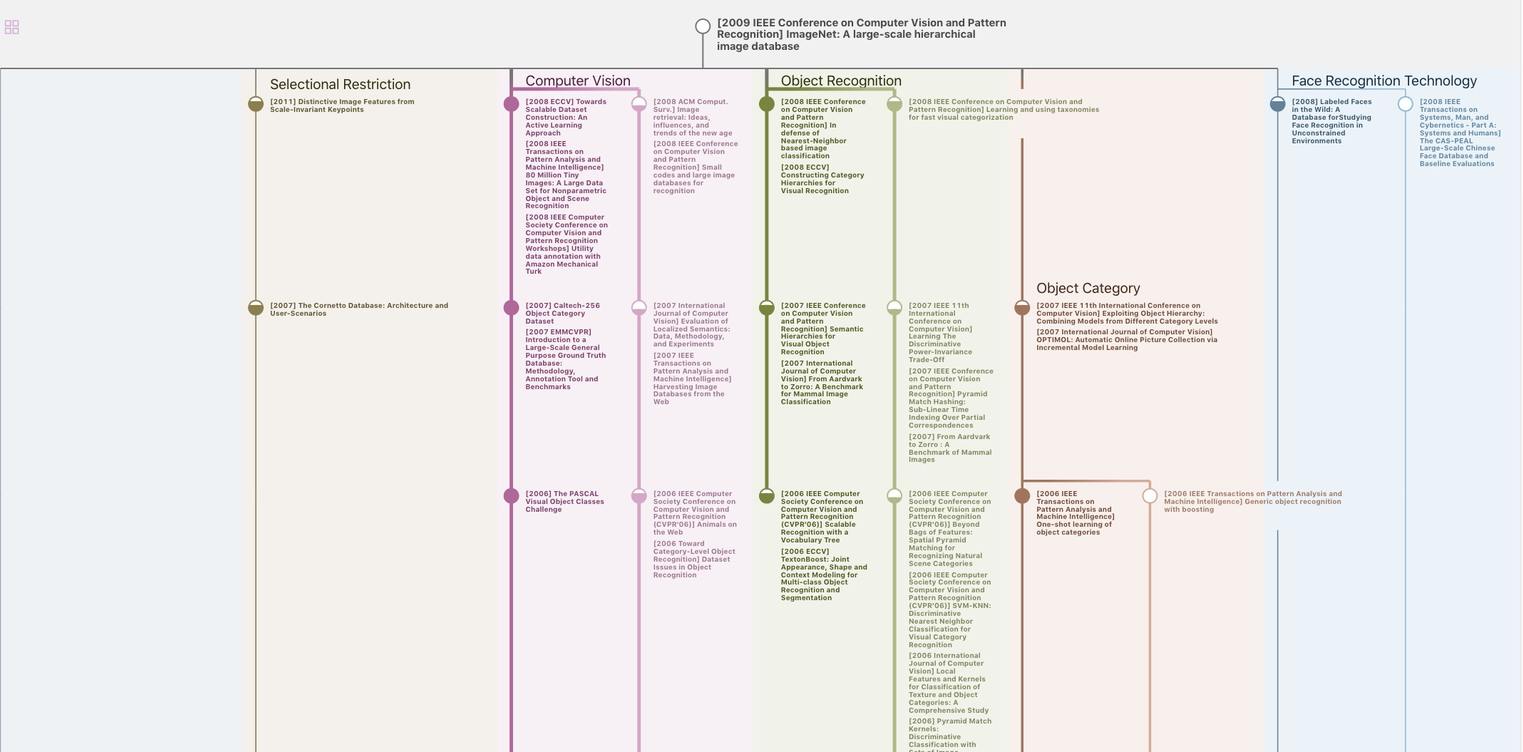
生成溯源树,研究论文发展脉络
Chat Paper
正在生成论文摘要