Online Nonstochastic Model-Free Reinforcement Learning
NeurIPS(2023)
摘要
In this work, we explore robust model-free reinforcement learning algorithms for environments that may be dynamic or even adversarial. Conventional state-based policies fail to accommodate the challenge imposed by the presence of unmodeled disturbances in such settings. Additionally, optimizing linear state-based policies pose obstacle for efficient optimization, leading to nonconvex objectives even in benign environments like linear dynamical systems. Drawing inspiration from recent advancements in model-based control, we introduce a novel class of policies centered on disturbance signals. We define several categories of these signals, referred to as pseudo-disturbances, and corresponding policy classes based on them. We provide efficient and practical algorithms for optimizing these policies. Next, we examine the task of online adaptation of reinforcement learning agents to adversarial disturbances. Our methods can be integrated with any black-box model-free approach, resulting in provable regret guarantees if the underlying dynamics is linear. We evaluate our method over different standard RL benchmarks and demonstrate improved robustness.
更多查看译文
关键词
reinforcement,learning,model-free
AI 理解论文
溯源树
样例
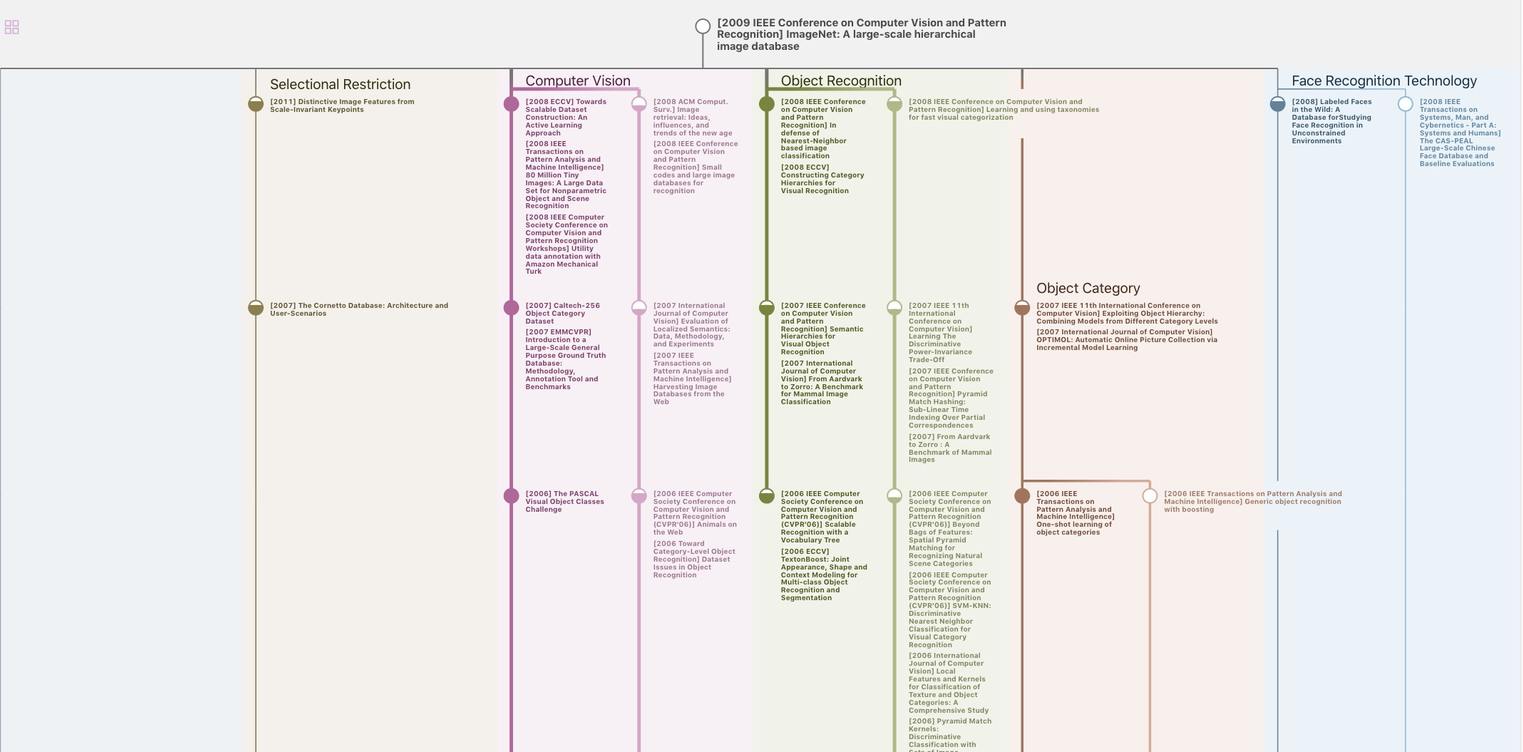
生成溯源树,研究论文发展脉络
Chat Paper
正在生成论文摘要