FoPro-KD: Fourier Prompted Effective Knowledge Distillation for Long-Tailed Medical Image Recognition
IEEE TRANSACTIONS ON MEDICAL IMAGING(2024)
摘要
Representational transfer from publicly available models is a promising technique for improving medical image classification, especially in long-tailed datasets with rare diseases. However, existing methods often overlook the frequency-dependent behavior of these models, thereby limiting their effectiveness in transferring representations and generalizations to rare diseases. In this paper, we propose FoPro-KD, a novel framework that leverages the power of frequency patterns learned from frozen pre-trained models to enhance their transferability and compression, presenting a few unique insights: 1) We demonstrate that leveraging representations from publicly available pre-trained models can substantially improve performance, specifically for rare classes, even when utilizing representations from a smaller pre-trained model. 2) We observe that pre-trained models exhibit frequency preferences, which we explore using our proposed Fourier Prompt Generator (FPG), allowing us to manipulate specific frequencies in the input image, enhancing the discriminative representational transfer. 3) By amplifying or diminishing these frequencies in the input image, we enable Effective Knowledge Distillation (EKD). EKD facilitates the transfer of knowledge from pre-trained models to smaller models. Through extensive experiments in long-tailed gastrointestinal image recognition and skin lesion classification, where rare diseases are prevalent, our FoPro-KD framework outperforms existing methods, enabling more accessible medical models for rare disease classification.
更多查看译文
关键词
Biomedical imaging,Adaptation models,Task analysis,Tuning,Data models,Transformers,Image classification,Visual prompting,knowledge distillation,long tailed learning
AI 理解论文
溯源树
样例
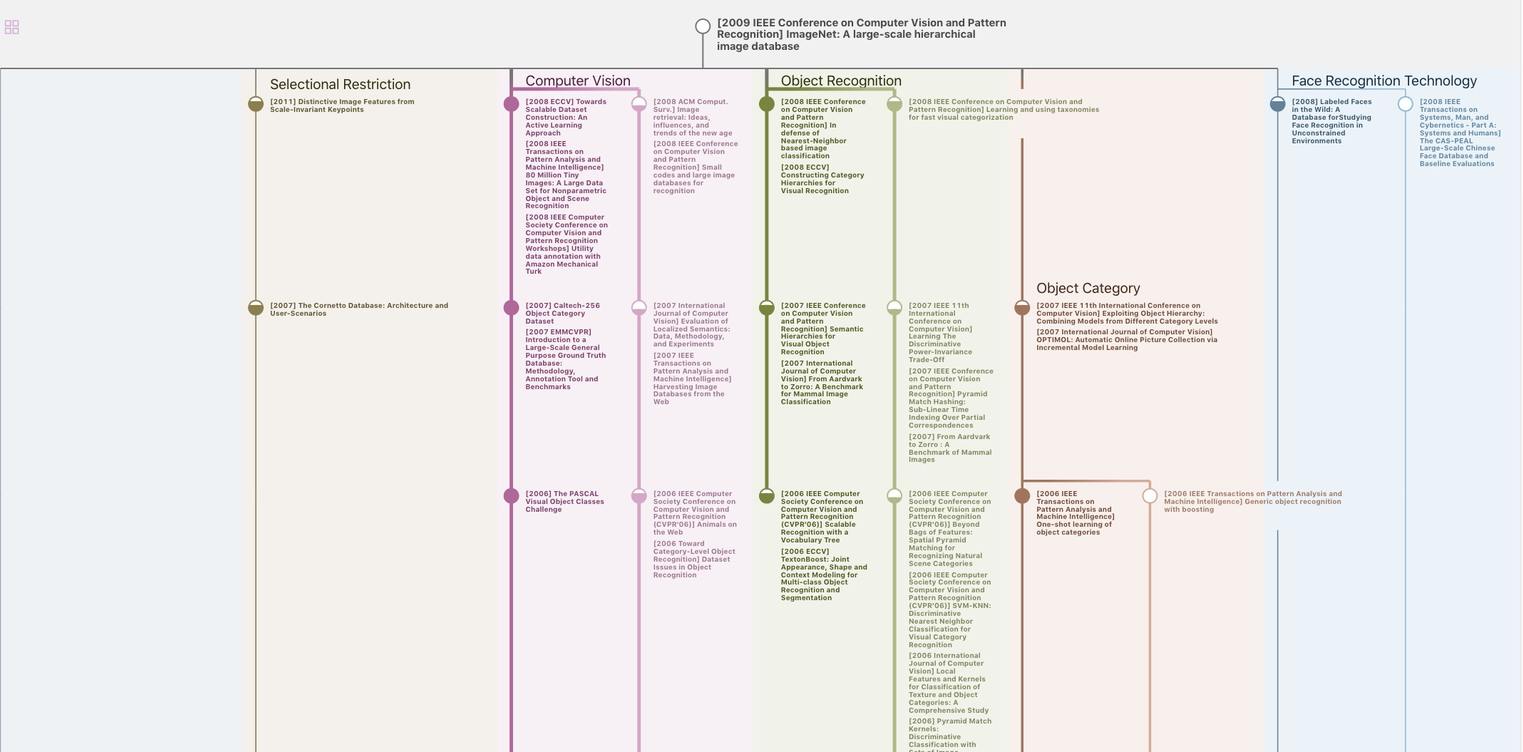
生成溯源树,研究论文发展脉络
Chat Paper
正在生成论文摘要