Exploring Better Text Image Translation with Multimodal Codebook
conf_acl(2023)
摘要
Text image translation (TIT) aims to translate the source texts embedded in the image to target translations, which has a wide range of applications and thus has important research value. However, current studies on TIT are confronted with two main bottlenecks: 1) this task lacks a publicly available TIT dataset, 2) dominant models are constructed in a cascaded manner, which tends to suffer from the error propagation of optical character recognition (OCR). In this work, we first annotate a Chinese-English TIT dataset named OCRMT30K, providing convenience for subsequent studies. Then, we propose a TIT model with a multimodal codebook, which is able to associate the image with relevant texts, providing useful supplementary information for translation. Moreover, we present a multi-stage training framework involving text machine translation, image-text alignment, and TIT tasks, which fully exploits additional bilingual texts, OCR dataset and our OCRMT30K dataset to train our model. Extensive experiments and in-depth analyses strongly demonstrate the effectiveness of our proposed model and training framework.
更多查看译文
关键词
better text image translation,multimodal codebook
AI 理解论文
溯源树
样例
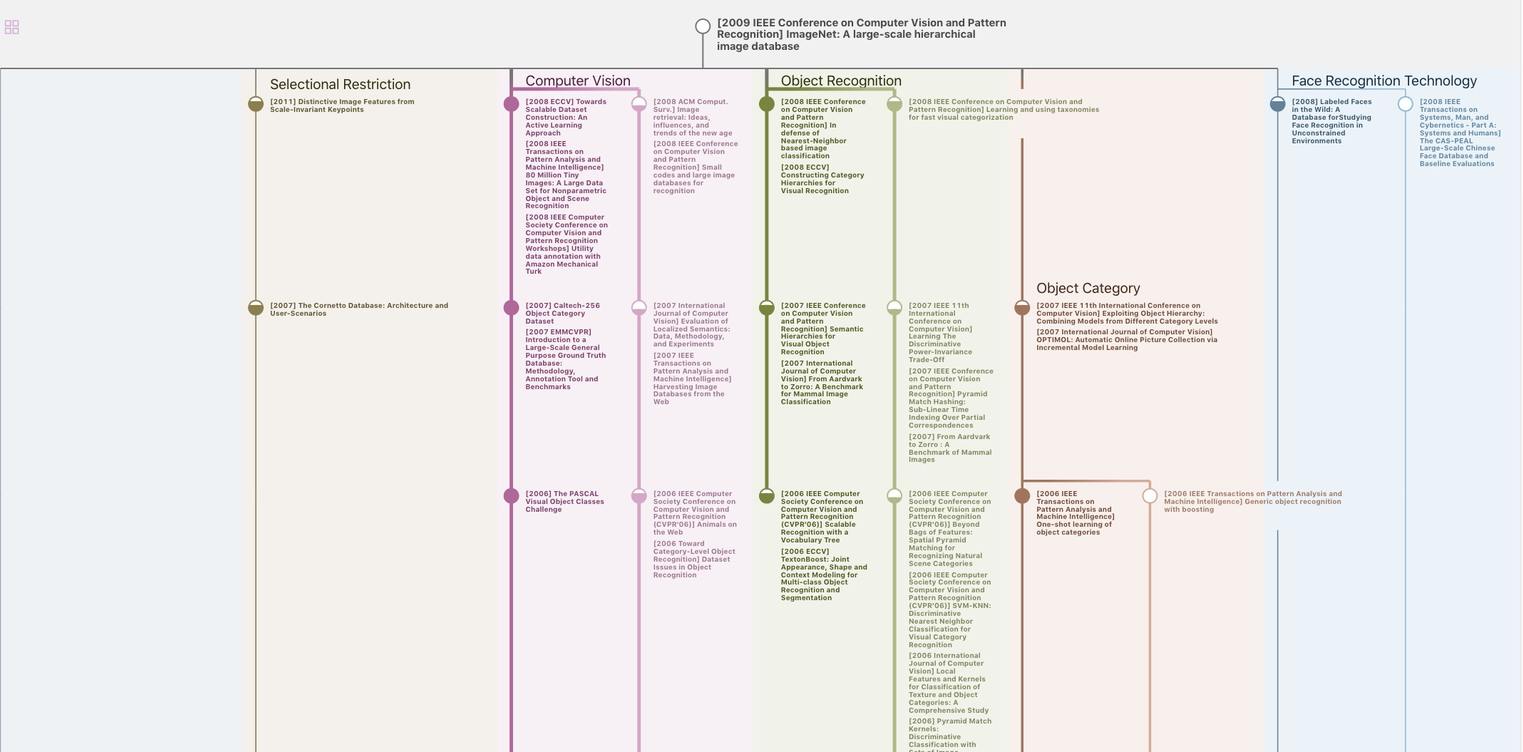
生成溯源树,研究论文发展脉络
Chat Paper
正在生成论文摘要