Optimizing Representation in Redistricting: Dual Bounds for Partitioning Problems with Non-Convex Objectives
arXiv (Cornell University)(2023)
摘要
We investigate optimization models for the purpose of computational
redistricting. Our focus is on nonconvex objectives for estimating expected
black voter and political representation. The objectives are a composition of a
ratio of variables and a normal distribution's cumulative distribution function
(or "probit curve"). We extend the work of Validi et al.
, which presented robust implementation of contiguity
constraints. By developing mixed integer linear programming models that closely
approximate the nonlinear model, our approaches yield tight bounds on these
optimization problems and we exhibit their effectiveness on county-level data.
更多查看译文
关键词
redistricting problems,dual bounds,non-convex
AI 理解论文
溯源树
样例
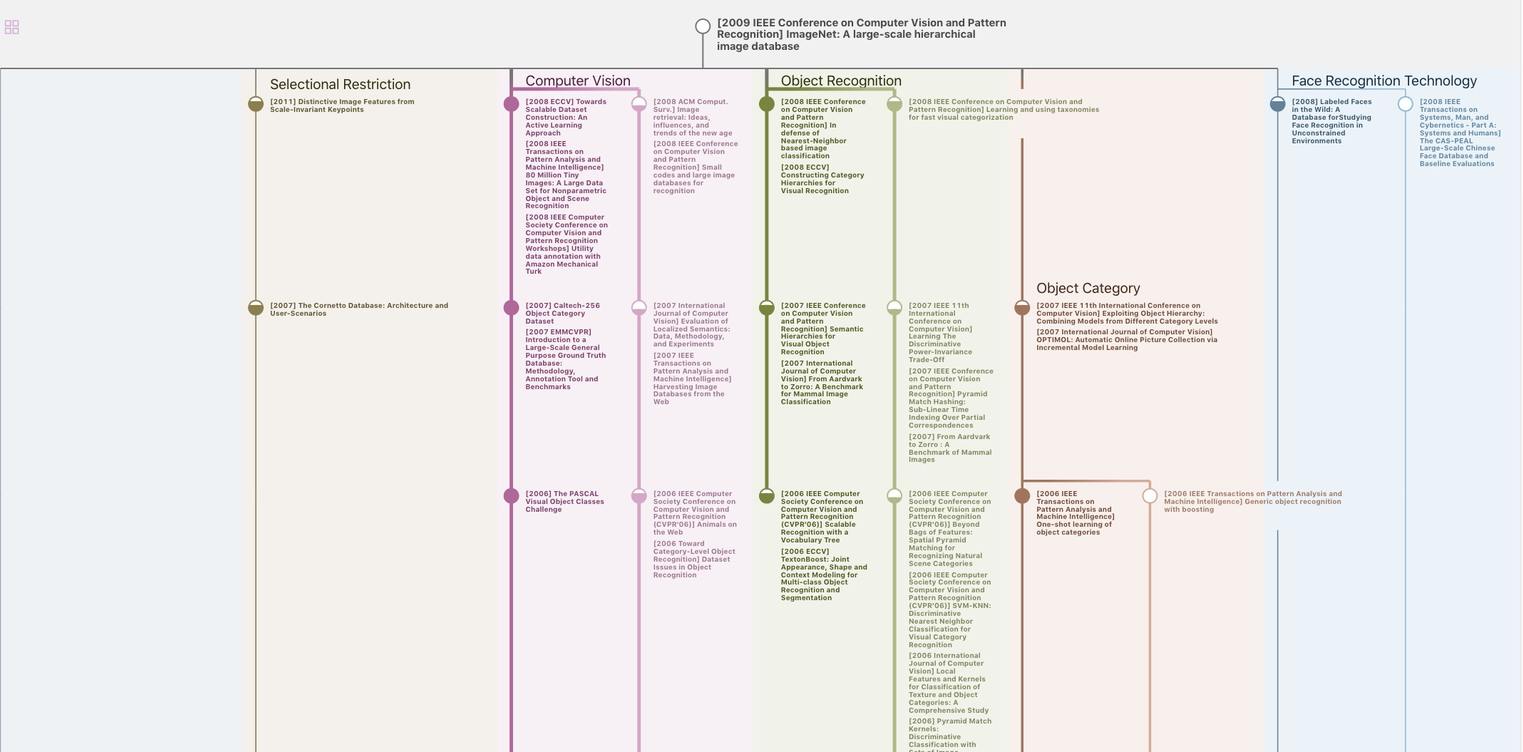
生成溯源树,研究论文发展脉络
Chat Paper
正在生成论文摘要