Building One-class Detector for Anything: Open-vocabulary Zero-shot OOD Detection Using Text-image Models
CoRR(2023)
摘要
We focus on the challenge of out-of-distribution (OOD) detection in deep learning models, a crucial aspect in ensuring reliability. Despite considerable effort, the problem remains significantly challenging in deep learning models due to their propensity to output over-confident predictions for OOD inputs. We propose a novel one-class open-set OOD detector that leverages text-image pre-trained models in a zero-shot fashion and incorporates various descriptions of in-domain and OOD. Our approach is designed to detect anything not in-domain and offers the flexibility to detect a wide variety of OOD, defined via fine- or coarse-grained labels, or even in natural language. We evaluate our approach on challenging benchmarks including large-scale datasets containing fine-grained, semantically similar classes, distributionally shifted images, and multi-object images containing a mixture of in-domain and OOD objects. Our method shows superior performance over previous methods on all benchmarks. Code is available at https://github.com/gyhandy/One-Class-Anything
更多查看译文
关键词
detection,one-class,open-vocabulary,zero-shot,text-image
AI 理解论文
溯源树
样例
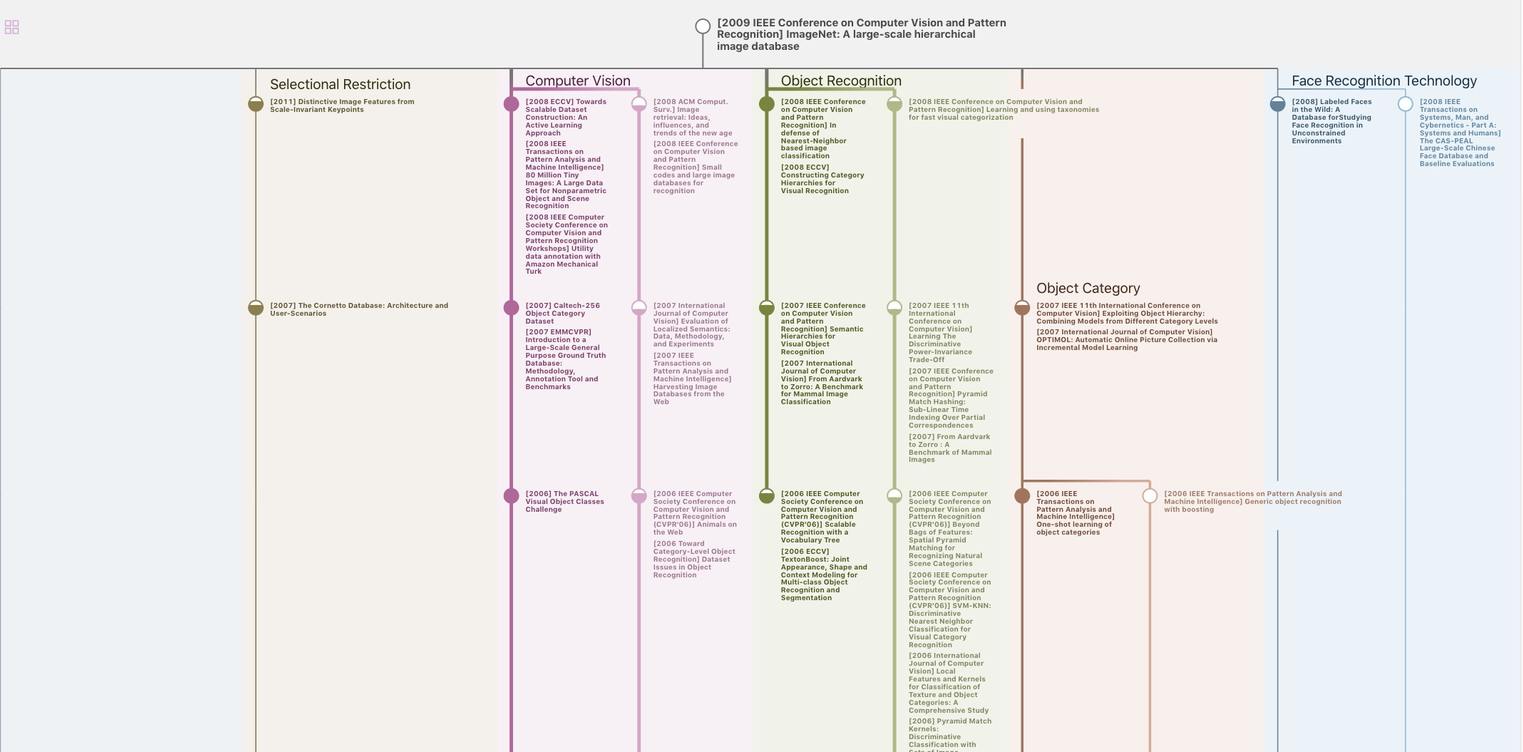
生成溯源树,研究论文发展脉络
Chat Paper
正在生成论文摘要