Explainable Ensemble Classification Model based on Argumentation
AAMAS '23: Proceedings of the 2023 International Conference on Autonomous Agents and Multiagent Systems(2023)
摘要
An ensemble classifier considers several base classifiers to make its predictions. It is generally seen as a black-box which, in addition, overlooks conflicts that may exist between base classifiers' rules. This paper proposes two novel ensemble classifiers that bridge the above gaps. They consider k base classifiers, each of which is a set of classification rules called theory, and a theory of domain knowledge. They build an argumentation system over the k+1 theories for identifying and solving possible conflicts between classification rules, and use the winning rules for making predictions. We show that the two classifiers guarantee some desirable properties including explainability, compliance to knowledge, and a global compatibility of the rules they use for making predictions.
更多查看译文
关键词
explainable ensemble classification model
AI 理解论文
溯源树
样例
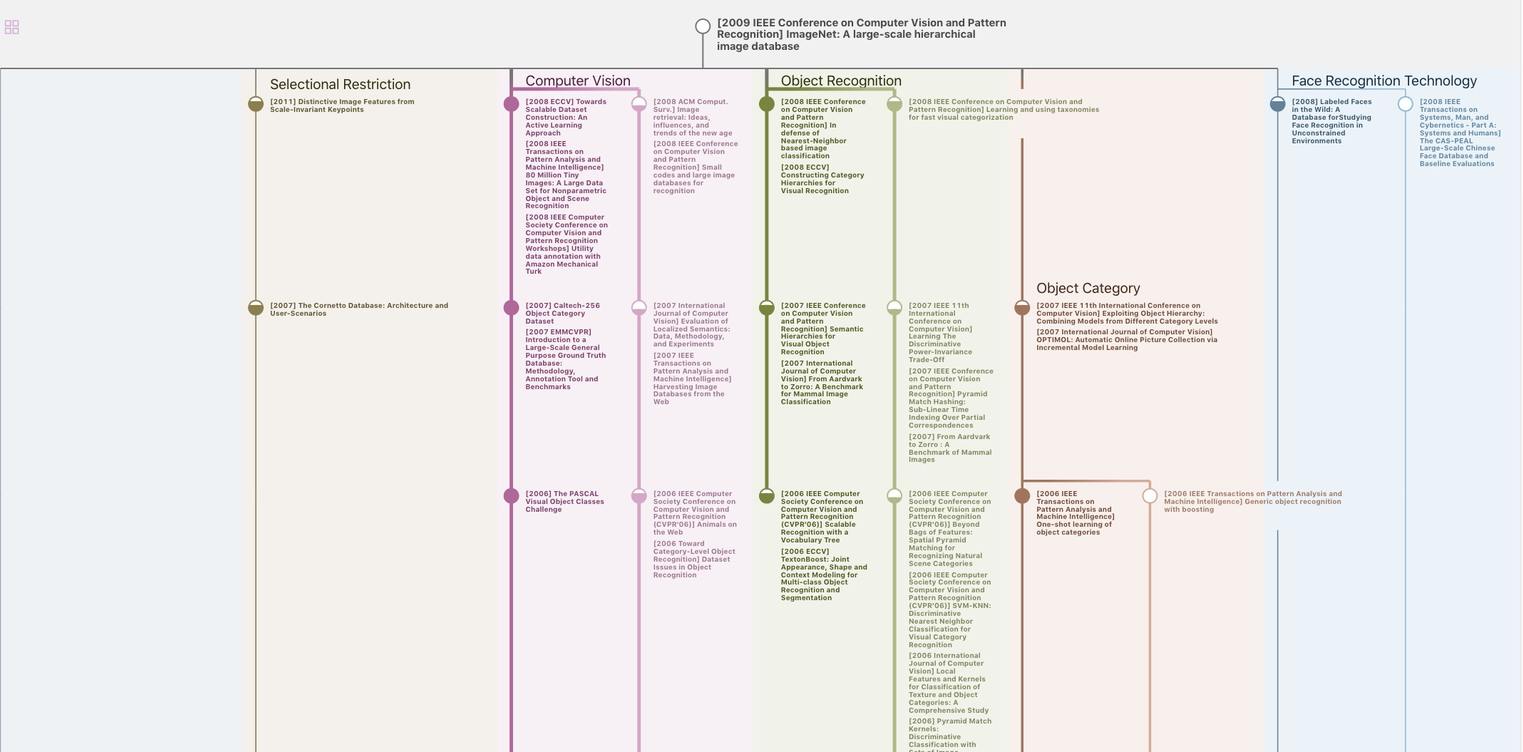
生成溯源树,研究论文发展脉络
Chat Paper
正在生成论文摘要