Hierarchical Reinforcement Learning with Attention Reward
AAMAS '23: Proceedings of the 2023 International Conference on Autonomous Agents and Multiagent Systems(2023)
摘要
Hierarchical Reinforcement Learning (HRL) is a promising approach for complex tasks with greater sample efficiency because it can break a task into sets of short subtasks and provide a denser subgoal-related intrinsic reward, making credit assignments less challenging. However, none of the conventional subgoal-related intrinsic rewards utilize task-specified knowledge,which limits the sample efficiency of these HRL methods. We propose Hierarchical Reinforcement Learning with Attention Reward (HiAR) that motivates agents to focus on the part of the environment controlled by their actions. We introduce a measure of the control over each dimension in the state space and discuss how we integrated it into the HRL method to improve the sample efficiency.
更多查看译文
AI 理解论文
溯源树
样例
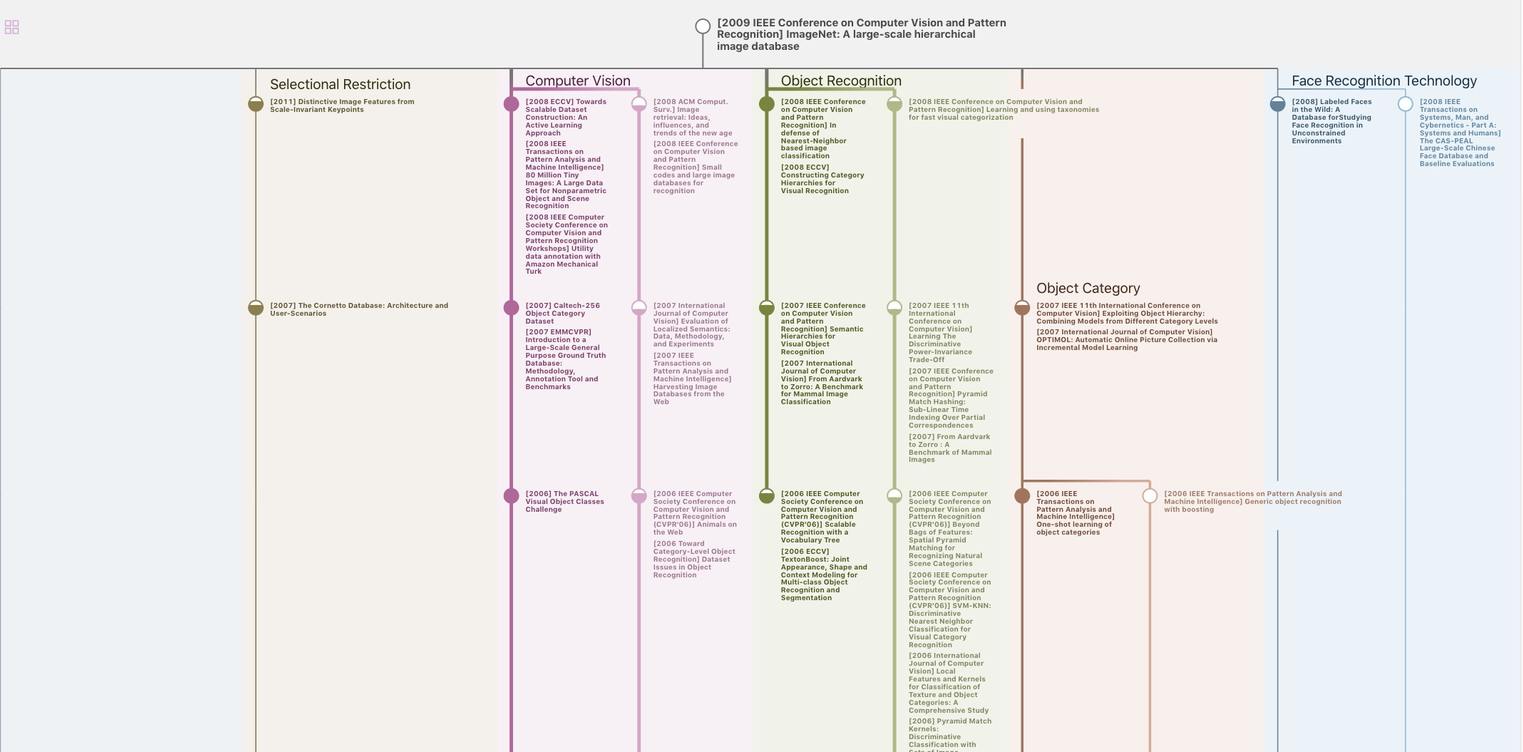
生成溯源树,研究论文发展脉络
Chat Paper
正在生成论文摘要