Towards Robust Contrastive Explanations for Human-Neural Multi-agent Systems
AAMAS '23: Proceedings of the 2023 International Conference on Autonomous Agents and Multiagent Systems(2023)
摘要
Generating explanations of high quality is fundamental to the development of trustworthy human-AI interactions. We here study the problem of generating contrastive explanations with formal robustness guarantees. We formalise a new notion of robustness and introduce two novel verification-based algorithms to (i) identify non-robust explanations generated by other methods and (ii) generate contrastive explanations augmented with provable robustness certificates. We present an implementation and evaluate the utility of the approach on two case studies concerning neural agents trained on credit scoring and image classification tasks.
更多查看译文
AI 理解论文
溯源树
样例
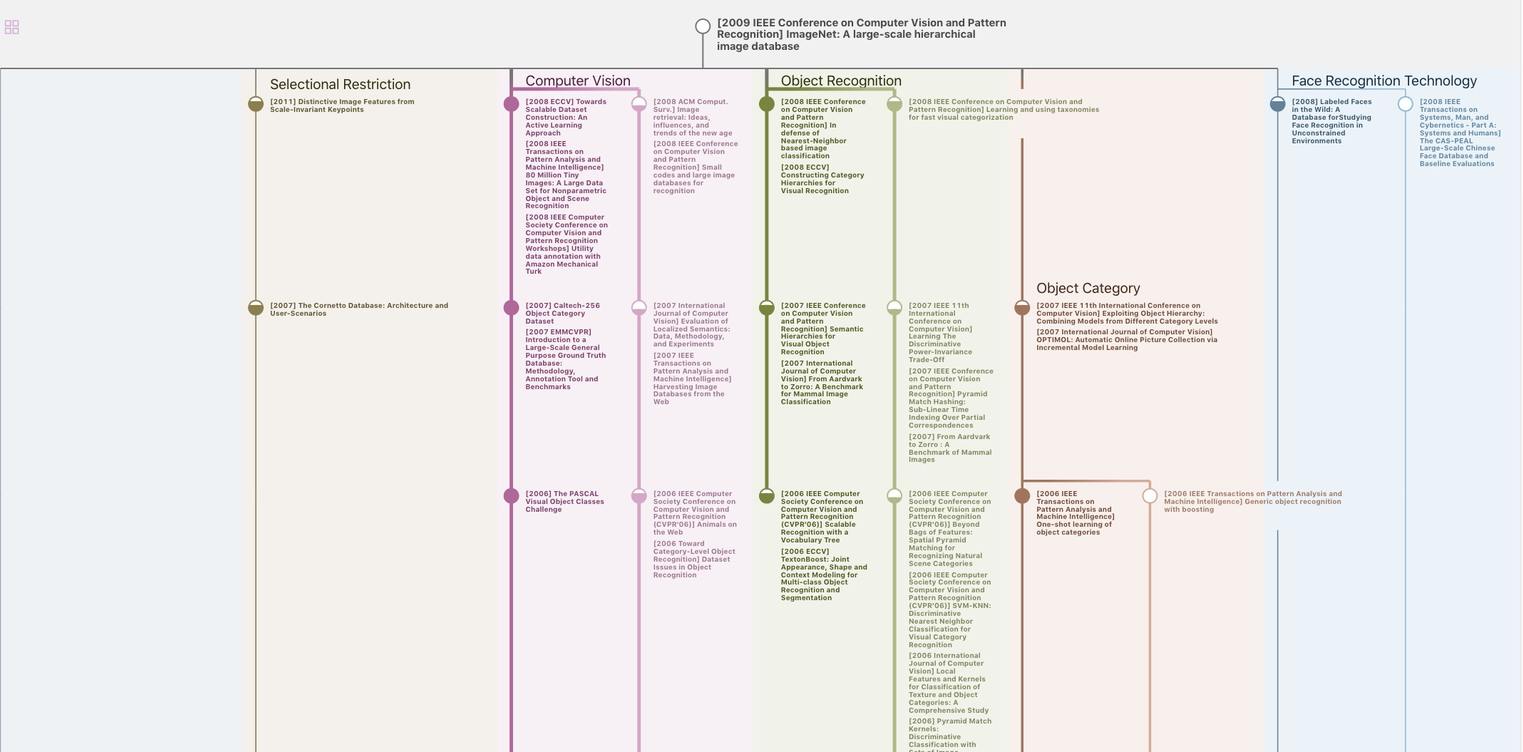
生成溯源树,研究论文发展脉络
Chat Paper
正在生成论文摘要