Qualitative Intention-aware Attribute-based Access Control Policy Refinement
SACMAT '23: Proceedings of the 28th ACM Symposium on Access Control Models and Technologies(2023)
摘要
Designing access control policies is often expensive and tedious due to the heterogeneous systems, services, and diverse user demands. Although ABAC policy and decision engine creation methods based on machine learning have been proposed, they cannot make good access decisions for applications and situations not envisioned by the decision-makers who provide training examples. It results in over- and under-permissiveness. In this paper, we propose a framework that refines pre-developed policies. It creates a decision engine that makes better decisions than those policies. Inspired by multiple criteria decision theory, our method uses the policy manager's qualitative intentions behind their judgments to guide access decisions so that more benefits are expected. In the evaluation, we prepare a coarse and relatively elaborate policy. We refine the coarse policy to obtain a decision engine that is compared for the similarity in access decisions with the elaborate policy using AUC as a measure. The results show that our method improves the coarse policy by a difference of 12-26% in AUC and outperforms the conventional machine learning methods by a difference of 3-11% in AUC.
更多查看译文
关键词
ABAC policy,Machine learning,Decision theory,Actionable AI
AI 理解论文
溯源树
样例
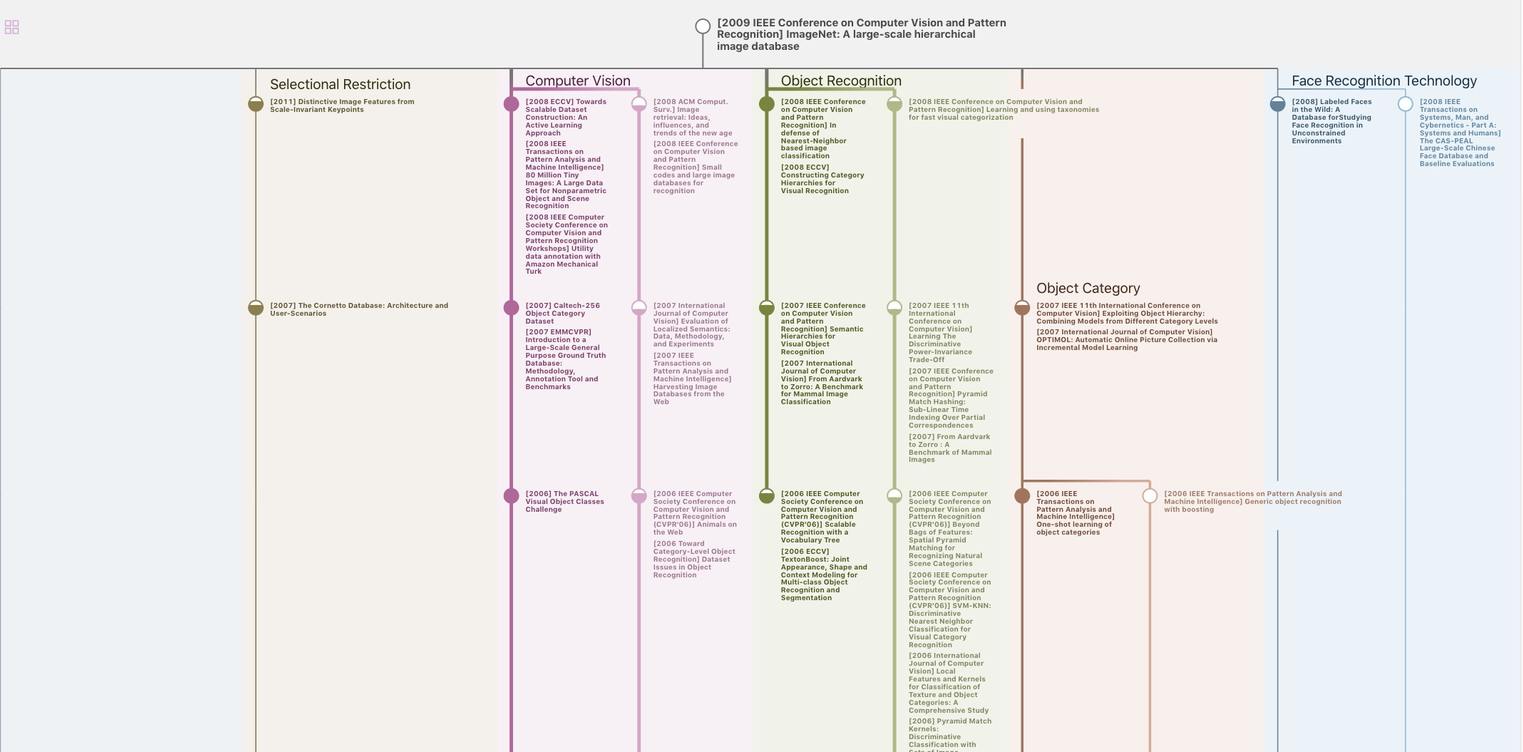
生成溯源树,研究论文发展脉络
Chat Paper
正在生成论文摘要