C2RL: Convolutional-Contrastive Learning for Reinforcement Learning Based on Self-Pretraining for Strong Augmentation.
Sensors(2023)
摘要
Reinforcement learning agents that have not been seen during training must be robust in test environments. However, the generalization problem is challenging to solve in reinforcement learning using high-dimensional images as the input. The addition of a self-supervised learning framework with data augmentation in the reinforcement learning architecture can promote generalization to a certain extent. However, excessively large changes in the input images may disturb reinforcement learning. Therefore, we propose a contrastive learning method that can help manage the trade-off relationship between the performance of reinforcement learning and auxiliary tasks against the data augmentation strength. In this framework, strong augmentation does not disturb reinforcement learning and instead maximizes the auxiliary effect for generalization. Results of experiments on the DeepMind Control suite demonstrate that the proposed method effectively uses strong data augmentation and achieves a higher generalization than the existing methods.
更多查看译文
关键词
deep reinforcement learning,self-supervised learning,contrastive learning,generalization,data augmentation,network randomization
AI 理解论文
溯源树
样例
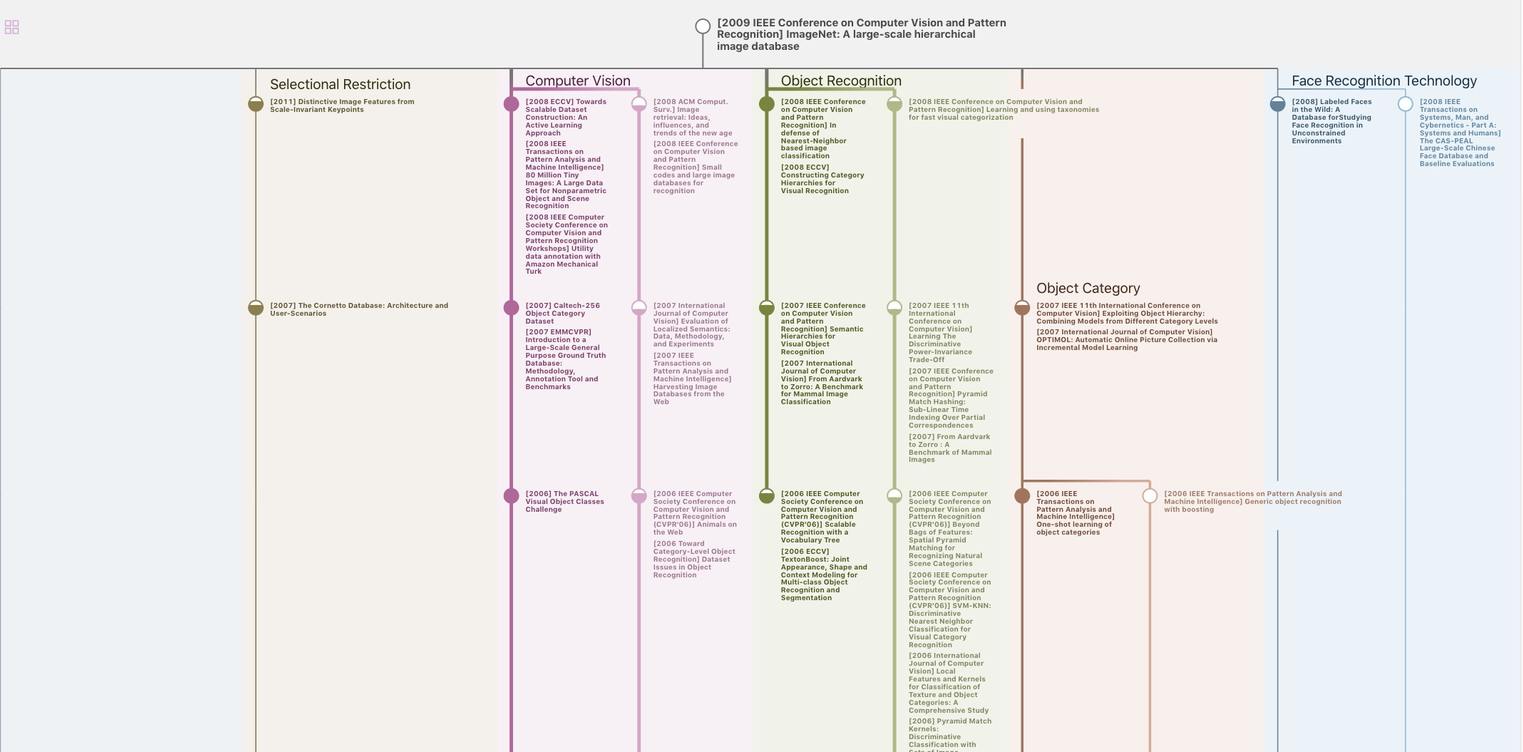
生成溯源树,研究论文发展脉络
Chat Paper
正在生成论文摘要