Manifold Regularization for Memory-Efficient Training of Deep Neural Networks
CoRR(2023)
摘要
One of the prevailing trends in the machine- and deep-learning community is to gravitate towards the use of increasingly larger models in order to keep pushing the state-of-the-art performance envelope. This tendency makes access to the associated technologies more difficult for the average practitioner and runs contrary to the desire to democratize knowledge production in the field. In this paper, we propose a framework for achieving improved memory efficiency in the process of learning traditional neural networks by leveraging inductive-bias-driven network design principles and layer-wise manifold-oriented regularization objectives. Use of the framework results in improved absolute performance and empirical generalization error relative to traditional learning techniques. We provide empirical validation of the framework, including qualitative and quantitative evidence of its effectiveness on two standard image datasets, namely CIFAR-10 and CIFAR-100. The proposed framework can be seamlessly combined with existing network compression methods for further memory savings.
更多查看译文
关键词
deep neural
AI 理解论文
溯源树
样例
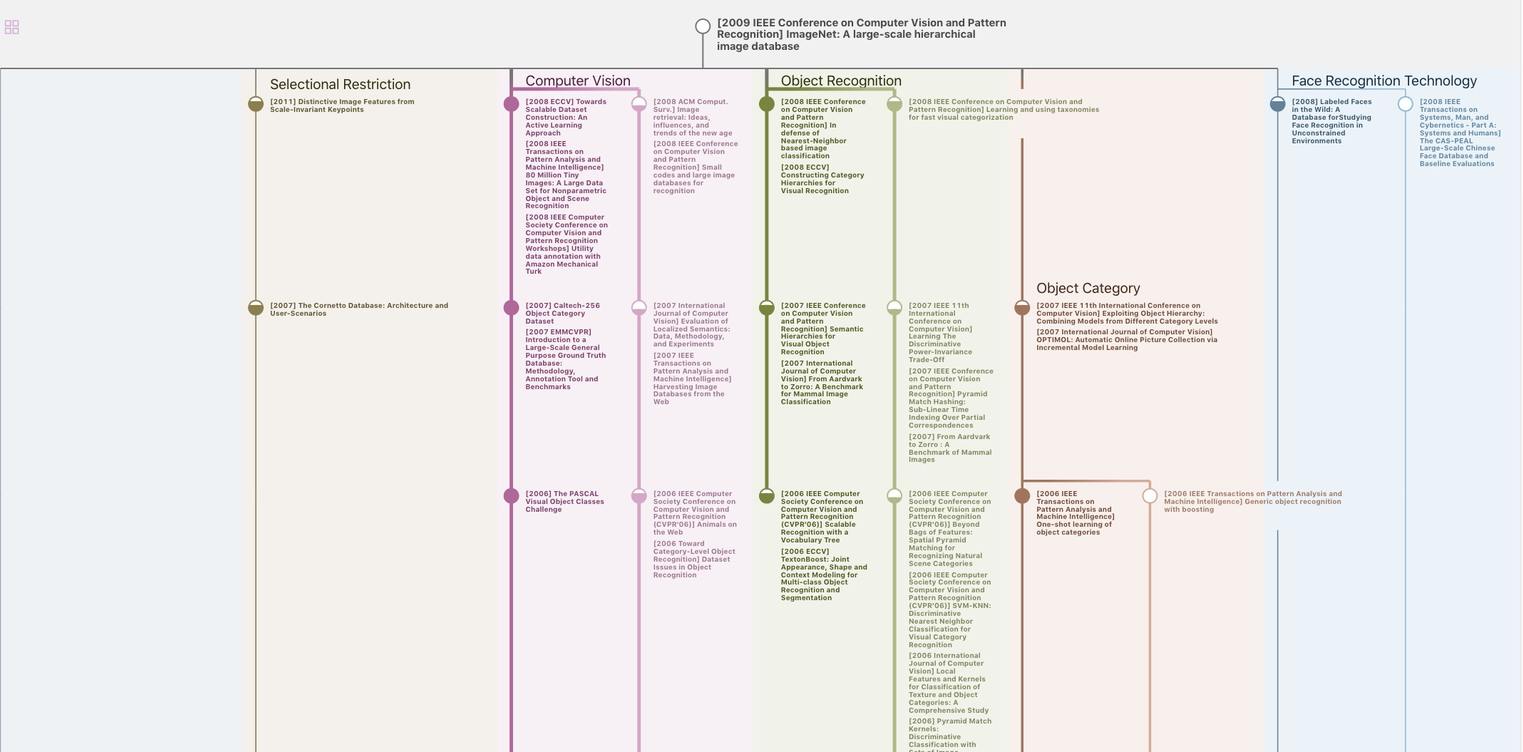
生成溯源树,研究论文发展脉络
Chat Paper
正在生成论文摘要