Policy Synthesis and Reinforcement Learning for Discounted LTL
CoRR(2023)
摘要
The difficulty of manually specifying reward functions has led to an interest in using linear temporal logic (LTL) to express objectives for reinforcement learning (RL). However, LTL has the downside that it is sensitive to small perturbations in the transition probabilities, which prevents probably approximately correct (PAC) learning without additional assumptions. Time discounting provides a way of removing this sensitivity, while retaining the high expressivity of the logic. We study the use of discounted LTL for policy synthesis in Markov decision processes with unknown transition probabilities, and show how to reduce discounted LTL to discounted-sum reward via a reward machine when all discount factors are identical.
更多查看译文
关键词
reinforcement learning,policy synthesis
AI 理解论文
溯源树
样例
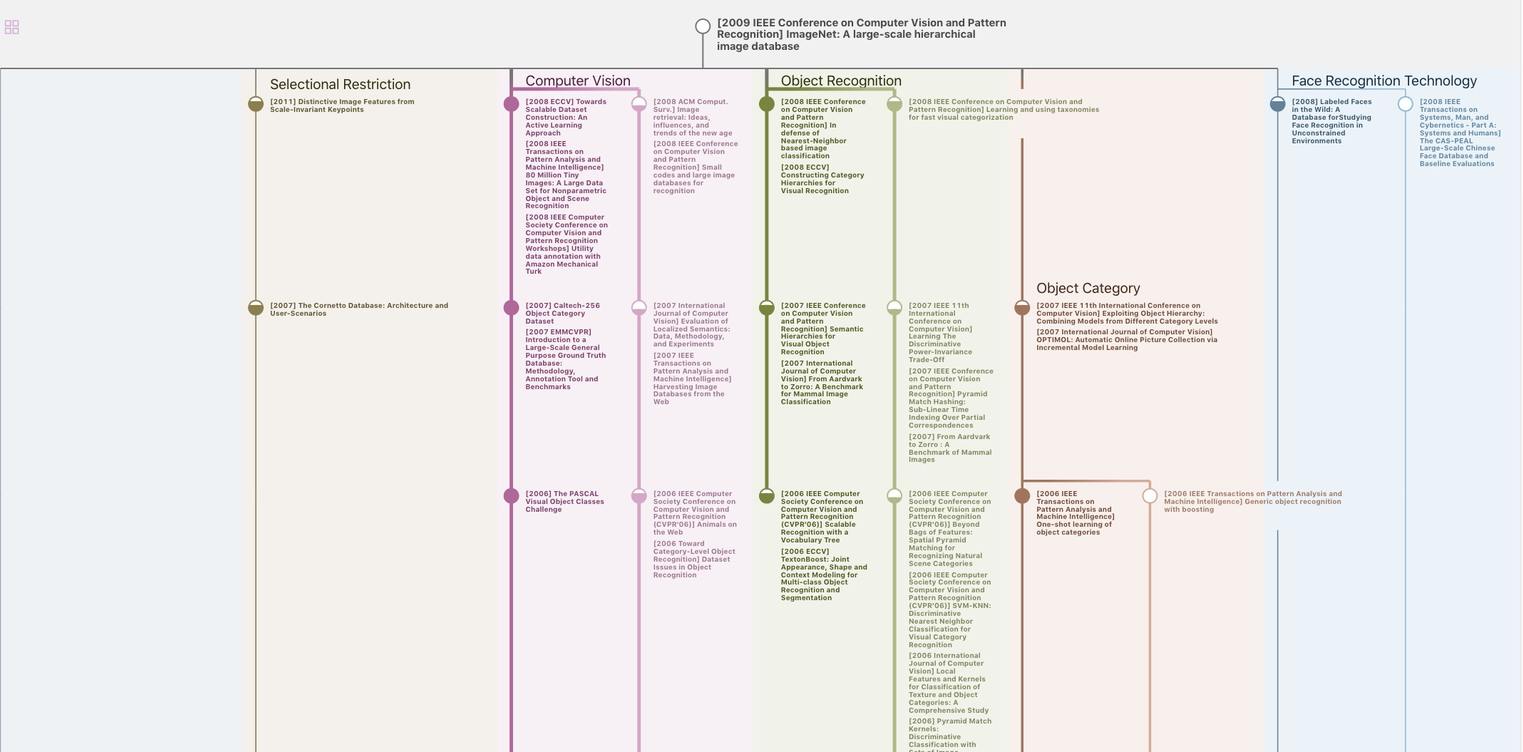
生成溯源树,研究论文发展脉络
Chat Paper
正在生成论文摘要