D-CALM: A Dynamic Clustering-based Active Learning Approach for Mitigating Bias
conf_acl(2023)
摘要
Despite recent advancements, NLP models continue to be vulnerable to bias. This bias often originates from the uneven distribution of real-world data and can propagate through the annotation process. Escalated integration of these models in our lives calls for methods to mitigate bias without overbearing annotation costs. While active learning (AL) has shown promise in training models with a small amount of annotated data, AL's reliance on the model's behavior for selective sampling can lead to an accumulation of unwanted bias rather than bias mitigation. However, infusing clustering with AL can overcome the bias issue of both AL and traditional annotation methods while exploiting AL's annotation efficiency. In this paper, we propose a novel adaptive clustering-based active learning algorithm, D-CALM, that dynamically adjusts clustering and annotation efforts in response to an estimated classifier error-rate. Experiments on eight datasets for a diverse set of text classification tasks, including emotion, hatespeech, dialog act, and book type detection, demonstrate that our proposed algorithm significantly outperforms baseline AL approaches with both pretrained transformers and traditional Support Vector Machines. D-CALM showcases robustness against different measures of information gain and, as evident from our analysis of label and error distribution, can significantly reduce unwanted model bias.
更多查看译文
关键词
active learning approach,mitigating bias,d-calm,clustering-based
AI 理解论文
溯源树
样例
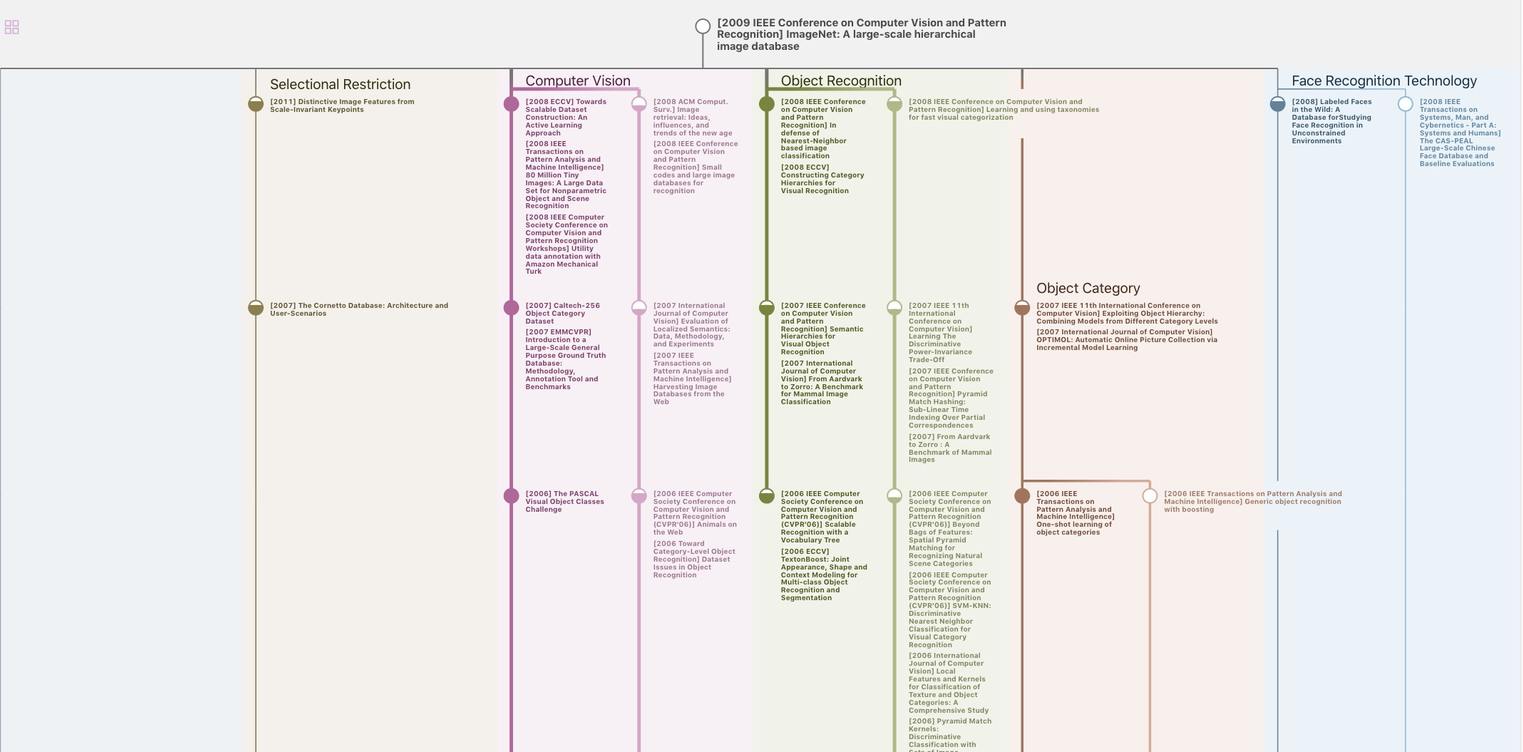
生成溯源树,研究论文发展脉络
Chat Paper
正在生成论文摘要