Future-conditioned Unsupervised Pretraining for Decision Transformer
CoRR(2023)
摘要
Recent research in offline reinforcement learning (RL) has demonstrated that return-conditioned supervised learning is a powerful paradigm for decision-making problems. While promising, return conditioning is limited to training data labeled with rewards and therefore faces challenges in learning from unsupervised data. In this work, we aim to utilize generalized future conditioning to enable efficient unsupervised pretraining from reward-free and sub-optimal offline data. We propose Pretrained Decision Transformer (PDT), a conceptually simple approach for unsupervised RL pretraining. PDT leverages future trajectory information as a privileged context to predict actions during training. The ability to make decisions based on both present and future factors enhances PDT's capability for generalization. Besides, this feature can be easily incorporated into a return-conditioned framework for online finetuning, by assigning return values to possible futures and sampling future embeddings based on their respective values. Empirically, PDT outperforms or performs on par with its supervised pretraining counterpart, especially when dealing with sub-optimal data. Further analysis reveals that PDT can extract diverse behaviors from offline data and controllably sample high-return behaviors by online finetuning. Code is available at here.
更多查看译文
关键词
unsupervised pretraining,transformer,future-conditioned
AI 理解论文
溯源树
样例
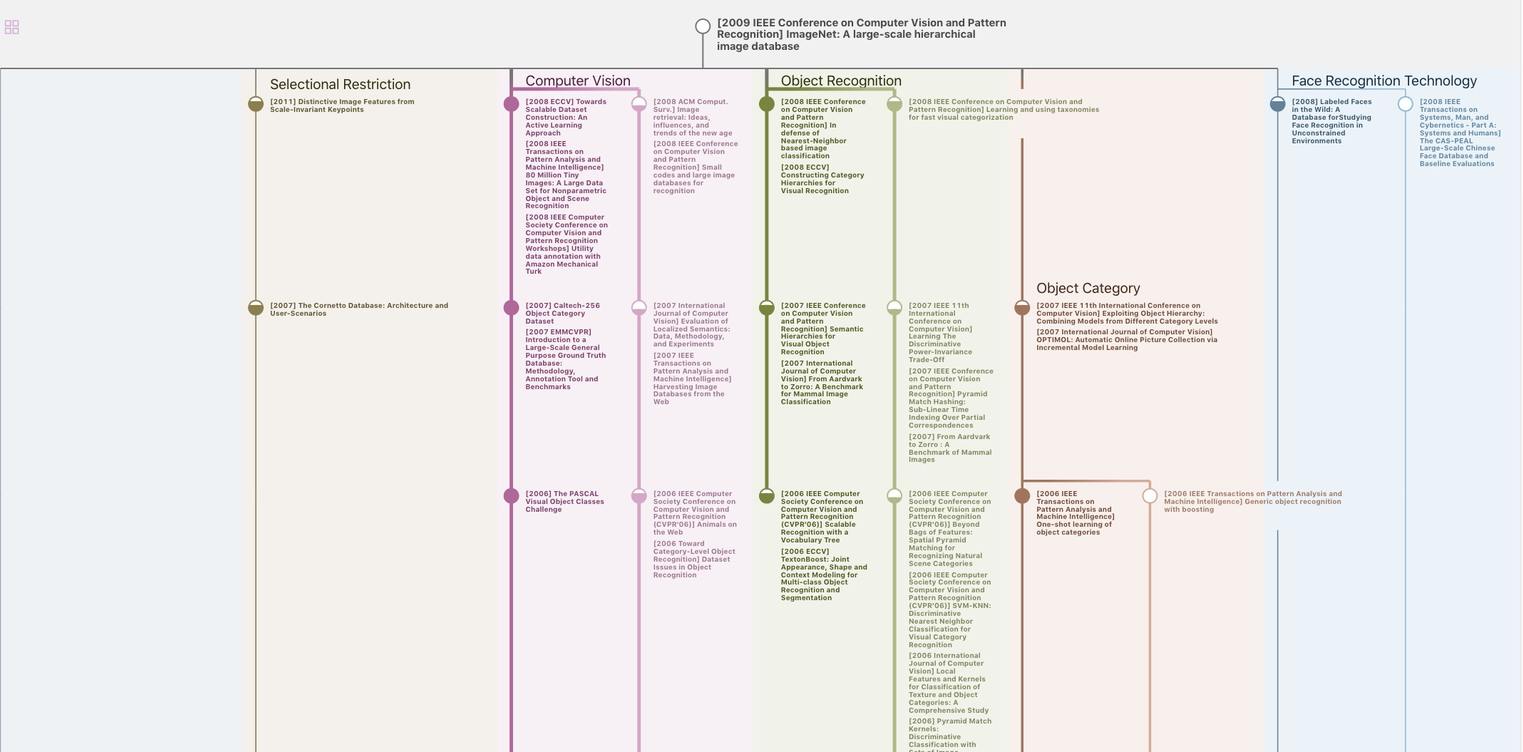
生成溯源树,研究论文发展脉络
Chat Paper
正在生成论文摘要