Not wacky vs. definitely wacky: A study of scalar adverbs in pretrained language models
CoRR(2023)
摘要
Vector space models of word meaning all share the assumption that words occurring in similar contexts have similar meanings. In such models, words that are similar in their topical associations but differ in their logical force tend to emerge as semantically close, creating well-known challenges for NLP applications that involve logical reasoning. Modern pretrained language models, such as BERT, RoBERTa and GPT-3 hold the promise of performing better on logical tasks than classic static word embeddings. However, reports are mixed about their success. In the current paper, we advance this discussion through a systematic study of scalar adverbs, an under-explored class of words with strong logical force. Using three different tasks, involving both naturalistic social media data and constructed examples, we investigate the extent to which BERT, RoBERTa, GPT-2 and GPT-3 exhibit general, human-like, knowledge of these common words. We ask: 1) Do the models distinguish amongst the three semantic categories of MODALITY, FREQUENCY and DEGREE? 2) Do they have implicit representations of full scales from maximally negative to maximally positive? 3) How do word frequency and contextual factors impact model performance? We find that despite capturing some aspects of logical meaning, the models fall far short of human performance.
更多查看译文
关键词
scalar adverbs,language models
AI 理解论文
溯源树
样例
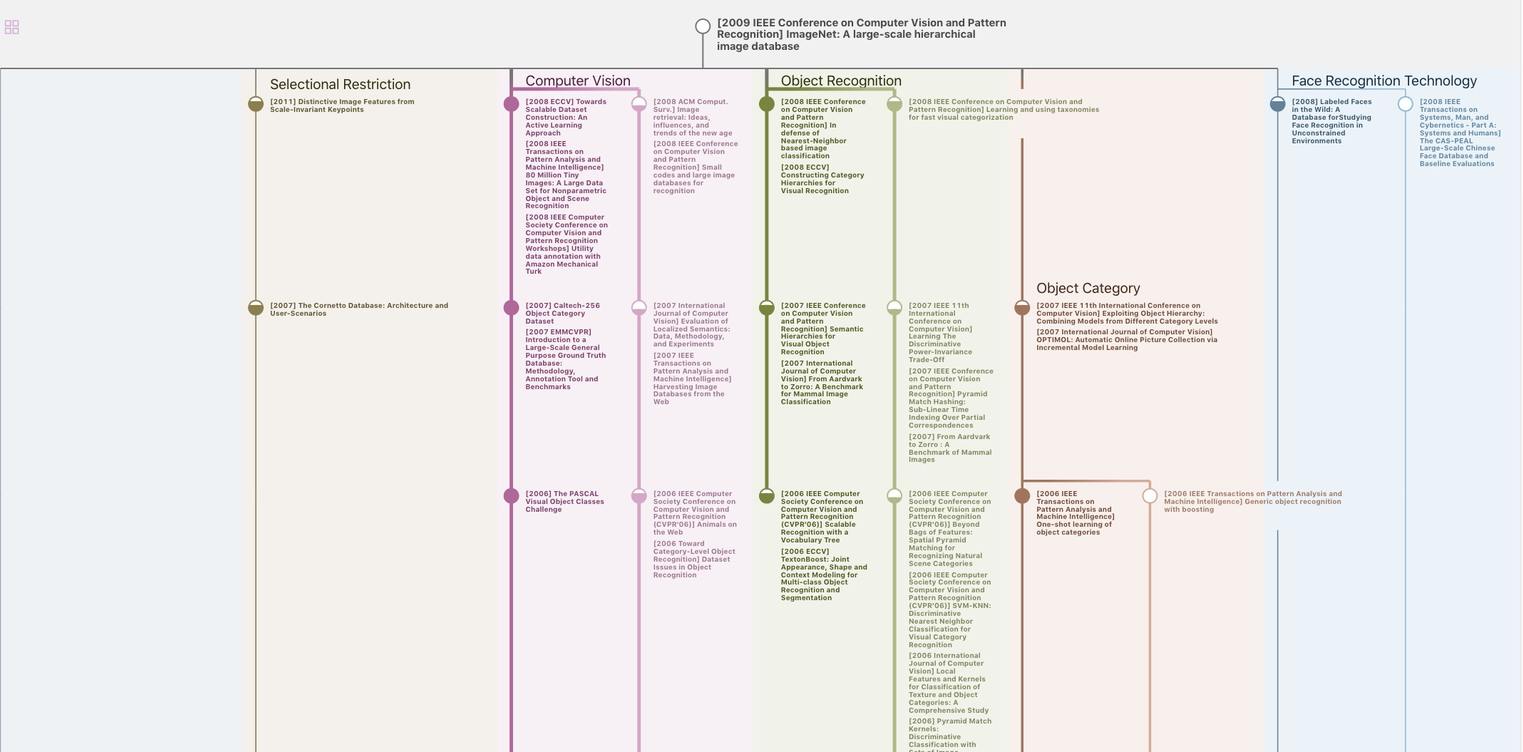
生成溯源树,研究论文发展脉络
Chat Paper
正在生成论文摘要