Counterfactual Probing for the influence of affect and specificity on Intergroup Bias
conf_acl(2023)
摘要
While existing work on studying bias in NLP focues on negative or pejorative language use, Govindarajan et al. (2023) offer a revised framing of bias in terms of intergroup social context, and its effects on language behavior. In this paper, we investigate if two pragmatic features (specificity and affect) systematically vary in different intergroup contexts -- thus connecting this new framing of bias to language output. Preliminary analysis finds modest correlations between specificity and affect of tweets with supervised intergroup relationship (IGR) labels. Counterfactual probing further reveals that while neural models finetuned for predicting IGR labels reliably use affect in classification, the model's usage of specificity is inconclusive. Code and data can be found at: https://github.com/venkatasg/intergroup-probing
更多查看译文
关键词
intergroup bias,counterfactual probing,affect,influence,specificity
AI 理解论文
溯源树
样例
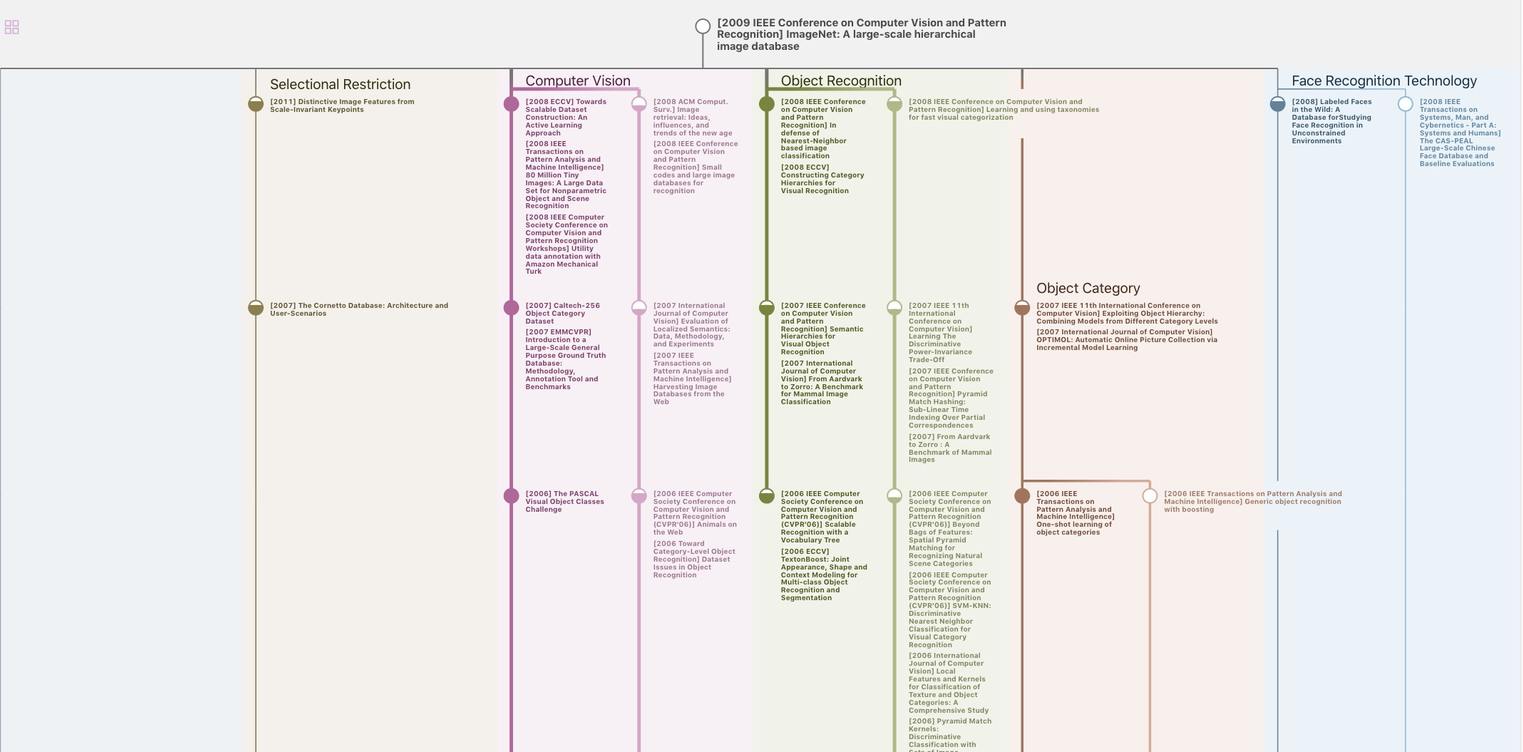
生成溯源树,研究论文发展脉络
Chat Paper
正在生成论文摘要