t-HGSP: Hypergraph Signal Processing Using t-Product Tensor Decompositions
IEEE Transactions on Signal and Information Processing over Networks(2023)
摘要
Graph signal processing (GSP) techniques are powerful tools that model complex relationships within large datasets, being now used in a myriad of applications in different areas including data science, communication networks, epidemiology, and sociology. Simple graphs can only model pairwise relationships among data which prevents their application in modeling networks with higher-order relationships. For this reason, some efforts have been made to generalize well-known graph signal processing techniques to more complex graphs such as hypergraphs, which allow capturing higher-order relationships among data. In this article, we provide a new hypergraph signal processing framework (t-HGSP) based on a novel tensor-tensor product algebra that has emerged as a powerful tool for preserving the intrinsic structures of tensors. The proposed framework allows the generalization of traditional GSP techniques while keeping the dimensionality characteristic of the complex systems represented by hypergraphs. To this end, the core elements of the t-HGSP framework are introduced, including the shifting operators and the hypergraph signal. The hypergraph Fourier space is also defined, followed by the concept of bandlimited signals and sampling. In our experiments, we demonstrate the benefits of our approach in applications such as clustering and denoising.
更多查看译文
关键词
Tensors,Signal processing,Fourier transforms,Laplace equations,Data models,Symmetric matrices,Point cloud compression,Graph,Signal Processing,Tensor,Data Analysis
AI 理解论文
溯源树
样例
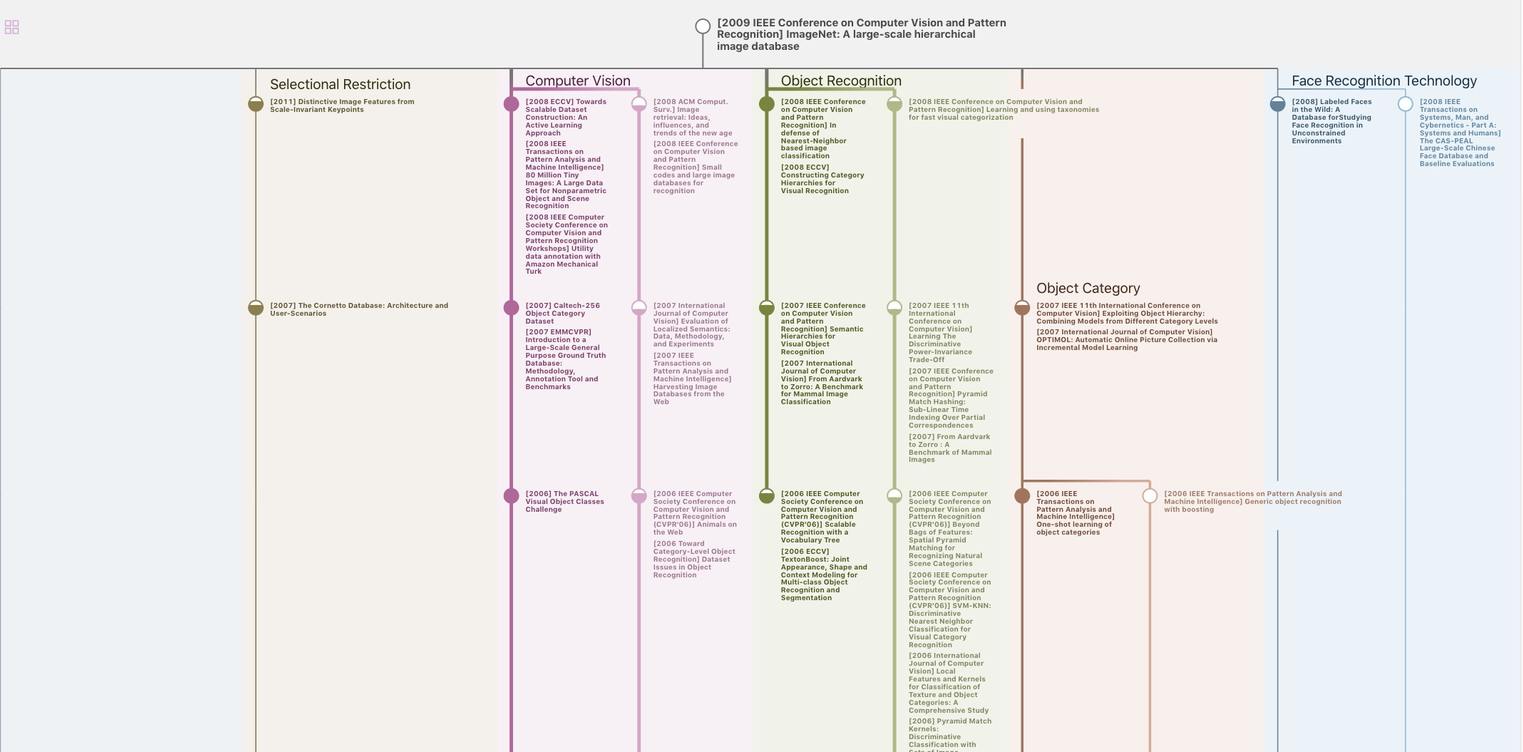
生成溯源树,研究论文发展脉络
Chat Paper
正在生成论文摘要