Attention-mechanism based DiPLS-LSTM and its application in industrial process time series big data prediction
Computers & Chemical Engineering(2023)
摘要
Big data and time series are typical features of modern industrial process data. Effective time series modeling methods are required for ensuring the normal and stable operation of industrial processes. However, the existing time series prediction methods usually cannot take important historical data features long ago into consideration. In this paper, we proposed a new attention-mechanism based dynamic-inner partial least squares long short-term memory (ADiPLS-LSTM) method to predict the time series data. First, the DiPLS method is applied to extract the dynamic features in the selected data group, and the attention-mechanism is used then to define the importance of the responded features. The product of the extracted dynamic features and the attention-mechanism results is regarded as the input of LSTM to predict the future time information. The proposed method uses both the recent data information and important data information in a long run, which helps to obtain more accurate prediction results. The effectiveness of the ADiPLS-LSTM method is verified by the data of a 660MW coal-fired boiler. Compared with BPNN, LSTM, and DiPLS-LSTM, the proposed method also advances in time series data prediction and interpretability.
更多查看译文
关键词
Time series,Attention-mechanism,DiPLS,LSTM,Coal-fired boiler
AI 理解论文
溯源树
样例
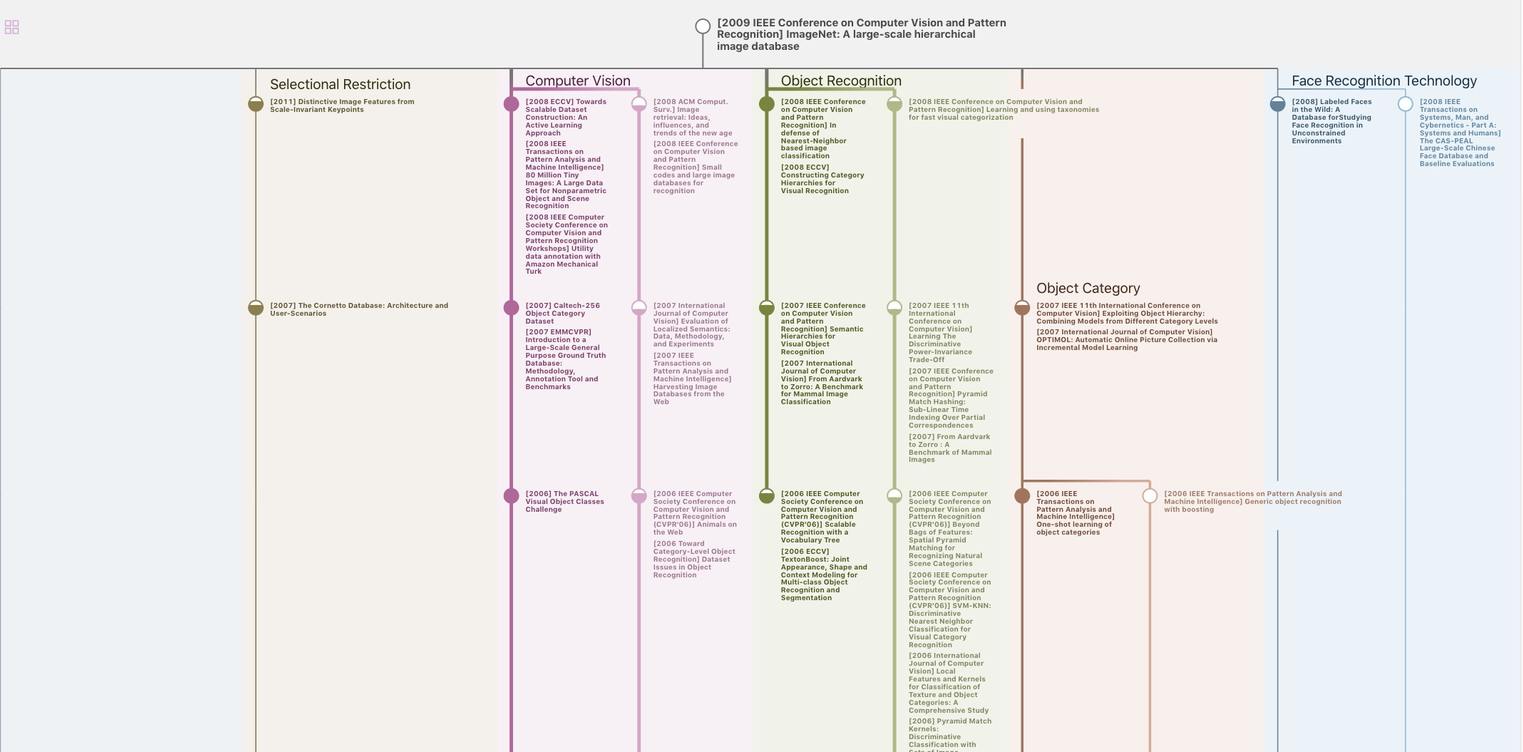
生成溯源树,研究论文发展脉络
Chat Paper
正在生成论文摘要