TripletPower: Deep-Learning Side-Channel Attacks over Few Traces
2023 IEEE International Symposium on Hardware Oriented Security and Trust (HOST)(2023)
摘要
Deep learning has been utilized as a promising technique in side-channel attacks. However, to recover keys successfully, deep-learning side-channel attacks often require thousands of training traces, which could be challenging for an attacker to obtain in the real world. This paper proposes a new deep-learning side-channel attack which only requires hundreds of training traces. Our proposed method, referred to as TripletPower, trains a triplet network, which learns a robust embedding for side-channel attacks with few traces. We demonstrate the advantage of our method in profiling attacks over power traces collected from AVR XMEGA and ARM STM32 microcontrollers using ChipWhisperer. Specffically, experimental results show that our method only needs as low as 250 training traces to train a classffier successfully recovering keys of unmasked AES on XMEGA (or STM32) while a Convolutional Neural Network needs at least 4,000 training traces in profiling attacks. In addition, we extend our method to non-profiling attacks with on-the-fly labeling. Experimental results suggest that our method can effectively recover keys of unmasked AES on XMEGA with only 525 unlabeled power traces in non-profiling attacks. Our method is also effective over power traces collected from masked AES and traces generated with random delay.
更多查看译文
AI 理解论文
溯源树
样例
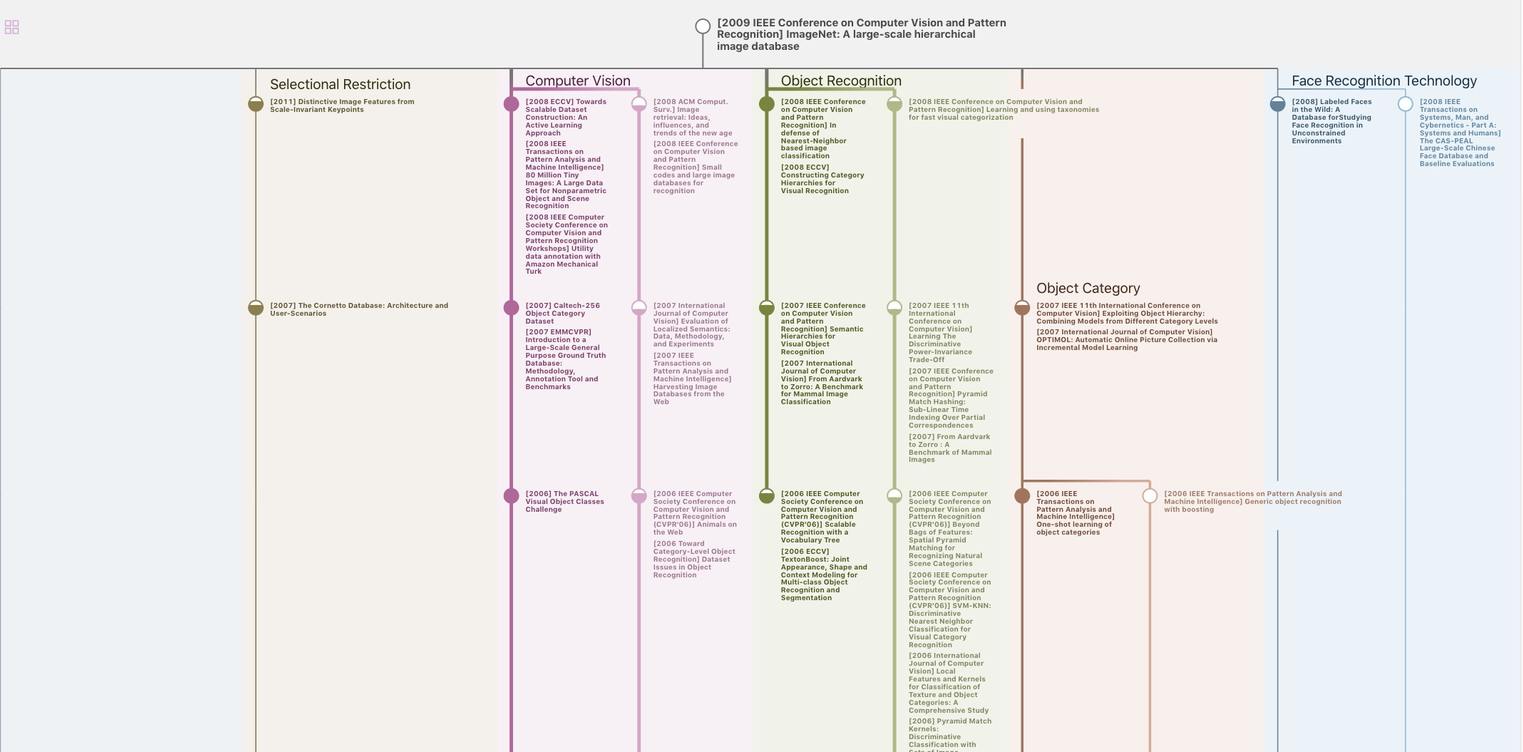
生成溯源树,研究论文发展脉络
Chat Paper
正在生成论文摘要